What makes a good (and bad) pharmaceutical forecast model
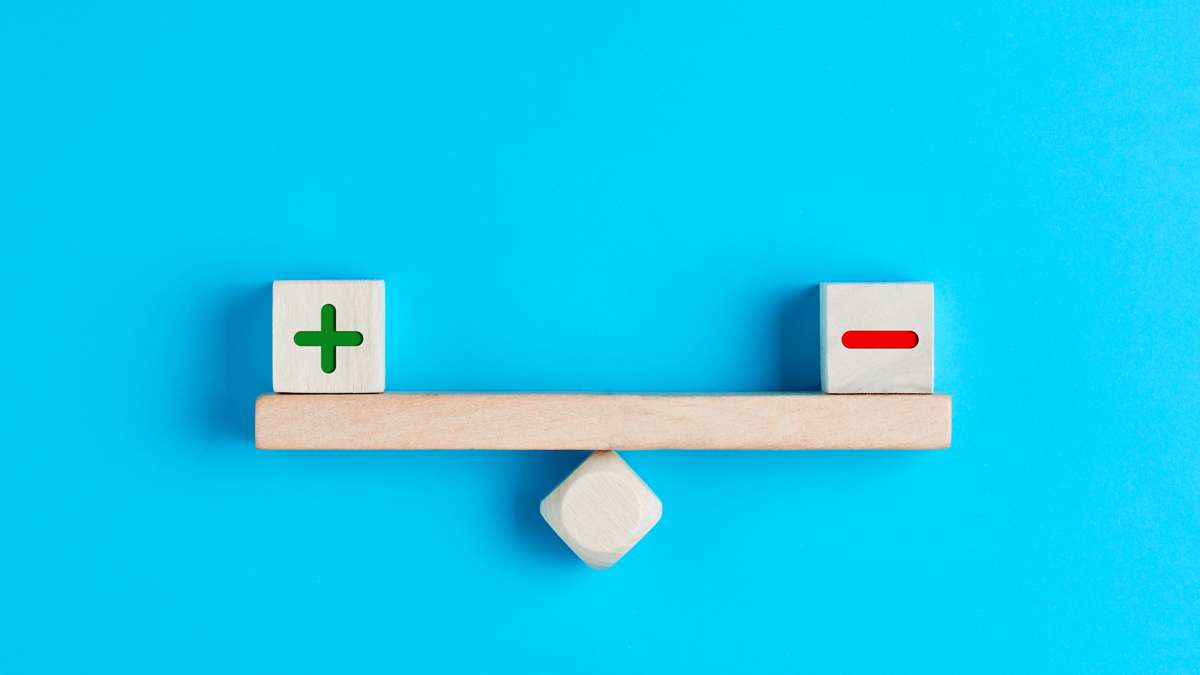
Forecasting is essential in providing clarity on decision making about the future, but poor-quality forecasts can result in decisions which are commercially damaging to organisations. Having good forecast models is critical for effective and efficient decision-making.
It’s widely accepted that there is no such thing as 100% accuracy in long range forecasting, but often inaccuracies are driven by a poorly built model which lacks transparency and doesn’t follow best practice, combined with poor assumptions.
It is possible to design a model that both minimises these inaccuracies and identifies where and why weak assumptions exist. Getting this right will help deliver accurate and reliable insights to help support business objectives and drive brand or company growth, highlight risk, and quantify opportunity.
In the sections below, we draw on our extensive experience of building bespoke pharmaceutical forecasting models to share the key ingredients needed for creating the most successful models.
#1: Know what good looks like when designing the model
Often inaccuracies creep in when teams are inexperienced in building models that use sound pharmaceutical forecasting principles. A lack of understanding of the approach and thought process involved in selecting the right model design and methodology can result in fundamental errors in the resulting forecast. In addition, a model that does not align with the business decision it supports brings little value.
What to do
When building a model, it’s necessary to select the right model structure and forecast methodology, this decision is not only about reflecting the structure of a disease that is being forecasted, but also about what data exists and precisely how much complexity is needed. Creating a sophisticated model for a complex disease can only work if the forecaster has access to the data and assumptions that make it work. Our approach is to create a strawman schematic of the required model design based on the disease and therapeutic structure, along with a future event audit. Then, map this against the available data. Where this data doesn’t exist, there are several options – change the model to reflect the lack of data, collect the data, or model the variability this lack of data creates.
#2: A good forecast will have no secrets
Forecasting exists to help organisations make important decisions around investments, such as the launching a new drug. A poorly built model will not deliver transparency, which means that vested interests will often drive assumptions and therefore deliver the outcomes a particular stakeholder is looking for, rather than an accurate forecast.
It will never be possible to drive politics out of your forecast completely, but creating a model where assumptions and their impact is clear and transparent will help highlight overly or negatively ambitious input drivers.
What to do
Apply a trending and event-based forecast methodology. This will allow for stakeholders to see where a market is tracking, based on the historical trend or baseline. Then, use events to model how the market will change in the future if, for example, a new product comes to market. The event will clearly communicate assumptions such as time to peak, source of business, or peak share. This approach will make the forecast clear and transparent to stakeholders and allow for them to challenge, or perhaps accept, with full knowledge.
#3: A model designed for everyone
Many models we see are overly complex, not due to their structure, but due to poor design. They are organic beasts that that have grown as more and more information has been added. It is not uncommon for the team to review models with hundreds of lines of data and more tabs than can be counted. The result is a web of confusing numbers with no clear logic or guidance and this type of chaotic approach not only leads to error and a lack of trust in the numbers, but it becomes a one-user model where only the creator knows how it works.
What to do
A forecast model, like any other software, should be built with users in mind. We have invested in model useability and apply a step-by-step approach to models that follows best practice principles with each section clearly explained. Other simple suggestions are to limit the number of colours and font sizes and avoid having too many rows on one tab, in order to keep each data section within one screen view.
#4: Flexibility built in
The model should be flexible in two ways. Firstly, it should be simple for new data or assumptions to be entered when they become available; secondly, it should allow for assumptions to be adjusted in order to pressure test the market or create independent scenarios that reflect a more positive or pessimistic future.
The benefit of a model that is easily updated is self-explanatory. However, why the need for pressure testing or scenarios?
Pressure testing forecast assumptions is a key forecasting step. It can identify key market levers that drive the most change. It can also allow for communication of the impact of less than perfect assumptions or data, so that the decision maker is fully cognisant of variance these might create.
Scenarios are key for planning. They allow for different future outcomes to be modelled and planned for, such as if a competitor does or doesn’t launch, or they can be used to evaluate returns of possible investment levels.
What to do
Using the trending and event approach previously discussed is the first step. Then, the key is to create varying versions of the model that are clearly labelled and filed.
#5: Keep it simple
There is often a push for increased complexity within models, perhaps because varying stakeholders want to see the data in differing ways, or perhaps because complexity is confused with accuracy. But, as any good forecaster knows, superfluous complexity is the enemy of a good forecast. At best, it can make the model difficult to maintain and communicate; at worst, it leads to guess upon guess being layered into the model, which will undermine confidence in the final forecast.
What to do
To be successful, a forecast model must be as simple as possible, but at the same time provide outputs that will support decision making. To determine where the balance should lie, it’s important to question the remit of the model: What functions of the business does the forecast model feed into? What outputs do these functions need to make accurate, informed decisions? By defining the remit, you can begin to identify whether features within the model are necessary additions or ‘nice-to-haves’ to keep it simple.
About the author
David James has a long history within global product forecasting and consulting and co-launched J+D Forecasting in 2005. He became CEO in 2011, as the company moved from purely consulting to developing forecast models, and has continued to evolve the business, using innovative technology to drive efficiencies for clients’ processes.