Taking the right forecasting approach for rare diseases
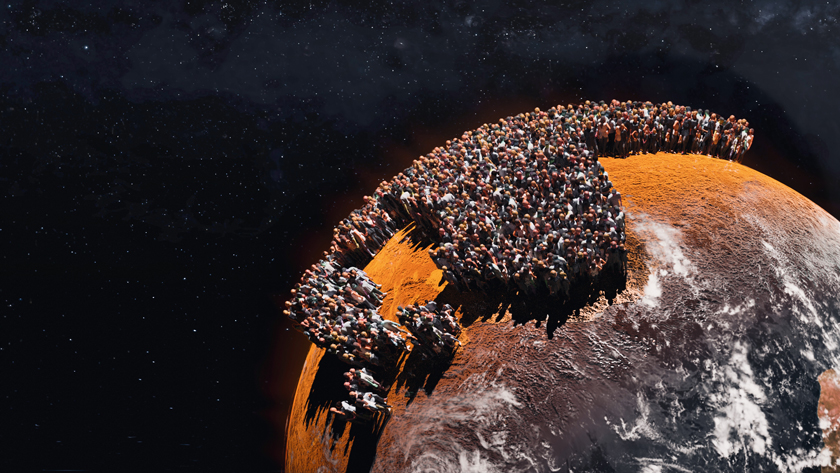
There are approximately 7,000 different types of rare disease and researchers estimate that there are more than 300 million people worldwide living with such a condition, according to the National Institutes of Health (NIH). However, only 5% of rare diseases are estimated to have at least one approved treatment, known as “orphan” therapies.
A rare disease is any disease that affects a small percentage of the population. With over 40% of all medicines in the pipeline aimed at rare diseases, and around 50 new therapies approved around the world each year, the future looks positive for increasing the number of treatments available.
There are clearly challenges to developing a drug targeting a rare disease, due to the small and often undiagnosed populations, and these challenges are also present when developing an accurate forecast of their market potential. The aim of the following is to identify some of these forecasting challenges and discuss ways in which they can be overcome.
Accuracy and an epidemiological approach
Generating accuracy in a rare disease forecast is extremely challenging, but at the same time, due to the value of an individual patient, absolutely imperative.
For a rare disease, an epidemiological approach is the right way to go. Partly, as there is unlikely to be any historical sales data to support a sales-based approach. But, also, because an epidemiology model will provide vital insights around patient numbers - key variables that will need to be strategized.
Data and market insights
The first point to make when talking about developing an epidemiology model for a rare disease is to keep it simple and only driven by the available data. It’s not advisable to create a multi-stepped patient funnel, looking at total population and prevalence through to target population, then number diagnosed, biomarker tested treated, etc. Starting a model with 500 million people and then overlaying the standard variables makes no sense when the treatable population is in the hundreds and there is little data to get you from one number to the next. Therefore, start at the point the data allows - usually, prevalence per 100,000.
Case study: severe asthma
Some years ago, as new biologic treatments for severe, uncontrolled asthma were in development, and specific patient groups were being proposed for these treatments based upon blood eosinophil (EOS) counts. This could then result in many layers of epidemiology filters in order to reach the final target patients, with several assumptions such as percentage of patients with an EOS of greater than 400. This approach gave a weak basis for population data and thus created a high level of uncertainty in the model outputs.
Data validation and bottom-up analysis
Next, run a bottom-up validation. By looking at the market from the bottom up, it is possible to validate the prevalence per 100,000 estimates. Often, for a rare disease there will be just a handful of treatment centres, or a small number of specialists, so, go local and contact clinics or patient advocacy groups at a country or even sub-national level to help validate patient numbers. This approach may also provide more detailed insights around patient specific variables, such as age of severity, that will help improve your forecast accuracy.
Case study: CAR-T treatment
This is a complex and innovative new treatment, which is highly personalised, as it involves collecting and using the patients’ own immune cells to treat their condition. It is currently used for specific oncology indications, such as some forms of leukaemia and multiple myeloma. When developing a forecast for smaller countries outside of the US or EU5, such as Switzerland, treatment is typically focused on a small number of specialist centres. For this situation, a forecast was constructed by profiling the number of hospitals prescribing Car-T treatments and then the likely number of patients being initiated per treatment centre. Taking this approach allowed the countries to forecast in an appropriate way, making it quicker, easier, and more accurate. A more conventional approach, using epi data and product market shares, would have resulted in inaccuracies and less transparency in the model.
Scenario planning and forecasting
Once an acceptable market size estimate has been developed by the forecaster, the challenge then is forecasting product uptake and its peak share. Again, lack of data makes this more challenging. Direct analogues are not available, so alternatives from a comparable market will provide useful insight, along with market research with prescribers and payors. With so many unknowns, scenario analysis is an absolute must to provide the likely impact of various investment, pricing, and uptake parameters.
Case study: pulmonary arterial hypertension (PAH)
When developing forecasts for new treatments in development, it was identified that a high level of uncertainty existed around key product assumptions and, therefore, it was critical to understand the impact of different assumptions. By copying the forecast model, it allowed for easy creation of alternative scenarios, giving complete flexibility around the assumptions that changed between the models. In addition, it was identified that sensitivity analysis on key, pre-set assumptions would allow rapid assessment of the related impact, thus providing several different approaches to understand potential impact of the uncertainty.
Treatment bandwidth and strategy
There is also an additional consideration which comes into play for rare diseases related to speed of uptake and peak share, and that is treatment bandwidth. With a rare disease, existing patients will take many years to diagnose, so a key strategy may be to shorten this timeline and drive patients to diagnosis and treatment sooner. However, the forecaster must consider the bandwidth of patient management, and how many patients can be diagnosed, treated, and managed with the existing treatment structure.
Closing comments
Additional disease and treatment challenges around, for example, drug delivery and dosing patient progression also need to be considered. But, despite the challenges, the pipeline of potential orphan products continues to grow significantly and holds great promise for novel treatments. Advances in clinical trial design and data analyses and availability, including mobile health technologies (wearable devices), offer the potential to revolutionise how clinical research is conducted, which will lead to better market insights and data upon which to base forecasts for these vital treatments in the future.
About the author
David James has a long history within global product forecasting and consulting and co-launched J+D in 2005. He became CEO in 2011, as the company moved from purely consulting to developing forecast models, and has continued to evolve the business, using innovative technology to drive efficiencies for clients’ processes. J+D Forecasting provides pharmaceutical forecasting solutions.