NIH algorithm matches patients to clinical trials
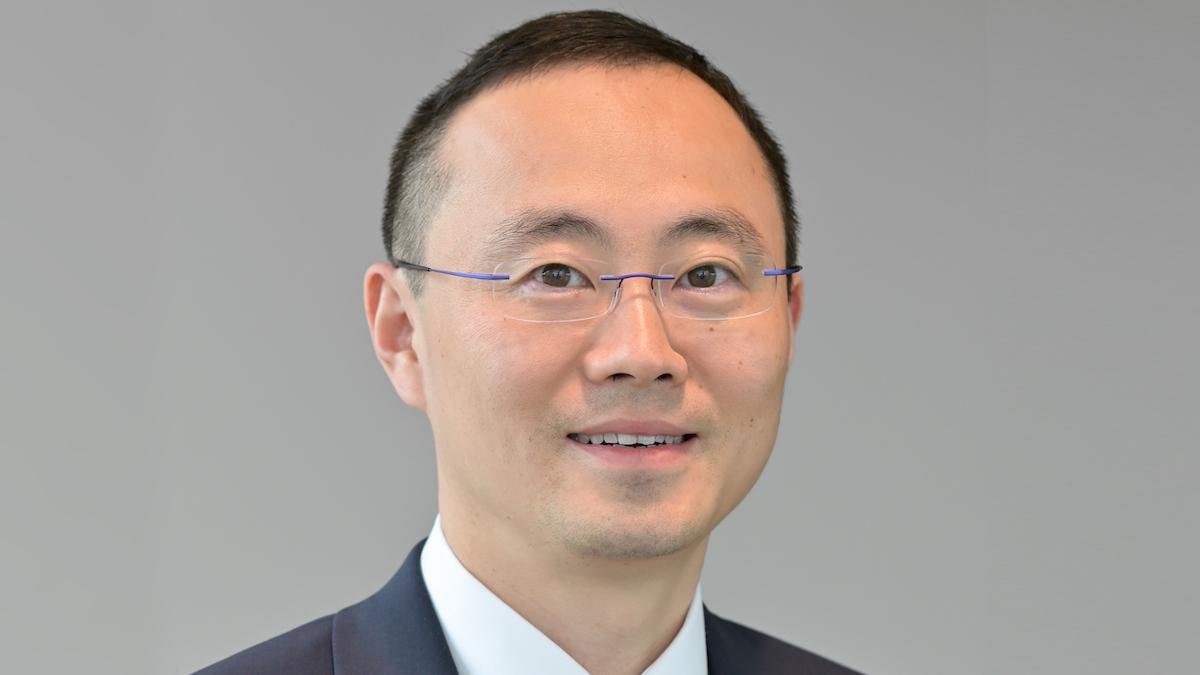
Zhiyong Lu, senior investigator NIH/NLM
Researchers from the National Institutes of Health (NIH) are applying artificial intelligence to the recruitment of volunteers into clinical trials, aiming to solve a major obstacle in clinical research.
Patient recruitment remains a major challenge for trial sponsors and can lead to delays and increased costs, and traditional methods can be time-consuming and inefficient.
The NIH team's large language model (LLM) – called TrialGPT and derived from ChatGPT – takes out the legwork in the process by identifying relevant clinical trials for which a person is eligible and providing a summary that explains how that person meets the criteria for study enrolment. They have published a paper on the platform in the journal Nature Communications.
It's been estimated that 80% of trials fail to recruit the required number of patients on time, a desultory performance sometimes blamed on a lack of innovation among trial sponsors in their processes – and may in part explain why drug development can take 10 to 15 years and many medicines never make it out of R&D.
In addition, it is thought that around 40% of cancer trials fail because of insufficient patient enrolment, according to lead author Zhiyong Lu of the NIH's National Library of Medicine (NLM), which developed TrialGPT with scientists at the National Cancer Institute (NCI).
"TrialGPT could help clinicians connect their patients to clinical trial opportunities more efficiently and save precious time that can be better spent on harder tasks that require human expertise," said Lu.
In the study, TrialGPT was used to match patients to suitable trials listed in the clinicaltrials.gov database and was able to match patients with an accuracy close to the performance achieved by human clinical research experts, according to the paper.
The researchers also carried out a pilot user study in which they asked two human clinicians to review six anonymous patient summaries and match them to six clinical trials. For each patient and trial pair, one clinician was asked to manually review the patient summaries, check if the person was eligible, and decide if the patient might qualify for the trial. For the same patient-trial pair, another clinician used TrialGPT to assess the patient's eligibility.
The researchers found that, when clinicians used TrialGPT, they spent 40% less time screening patients, but maintained the same level of accuracy.
"Machine learning and AI technology have held promise in matching patients with clinical trials, but their practical application across diverse populations still needed exploration," said Stephen Sherry, acting director of the NLM.
"This study shows we can responsibly leverage AI technology so physicians can connect their patients to a relevant clinical trial that may be of interest to them, with even more speed and efficiency," he added.
Patient recruitment is just one way that AI is being deployed to improve the clinical trials process. Sponsors have also started using advanced analytics to increase patient retention – another major problem with getting studies done on time and on budget – and to identify patients at high risk of illnesses who can then be encouraged to take part in trials.
The team behind TrialGPT have been invited to take part in a Director's Challenge Innovation Award to carry out further testing of the LLM and see how well it performs in real-world clinical settings.