Short-term crucial to future of AI drug discovery
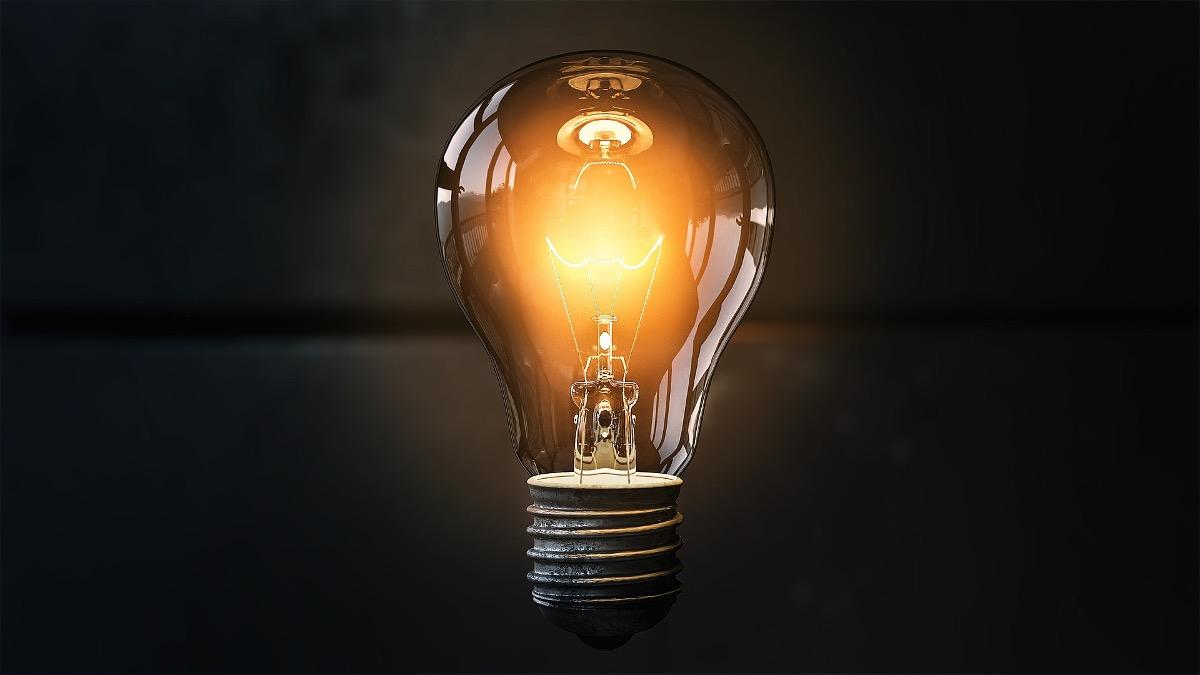
One of the lead prospects in a new generation of AI-developed drug candidates struggled in late-stage trials. Ben Hargreaves examines what happened, why there is such hope for this form of drug discovery, and the potential fallout for the space if more candidates encounter difficulties.
There is no doubt that the pharmaceutical industry faces a conundrum when it comes to R&D. On the one hand, it is extremely expensive to find and develop a potential new treatment, and there is a high failure rate. On the other hand, waiting for smaller companies to successfully progress their own candidates to then acquire them is also costly, and there are numerous examples where this approach has also failed. The appeal of applying artificial intelligence to drug design and development is obvious – if it can boost success rate in trials by only a small percentage, then the returns would be enormous.
AI is currently being placed under the microscope across a variety of industries, including for its chances of replacing physicians, but also for its potential to replace the entire human race. The latter threat was deemed real enough that the US White House convened a meeting with four leading CEOs in the field to develop ‘appropriate safeguards and protections’. However, for the most part, the utility of AI is currently locked into the possibility, rather than reality, of it being applied in various ways.
In the pharma industry, however, it has already been used to develop drug candidates that have gone on to be examined in clinical trials – a process that usually takes the industry years, but has been cut down to a matter of weeks by the technology. It is for this reason that investment in the space is predicted to soar in the short-term, with spend on the technology estimated to reach $3 billion by 2025. Unfortunately, the prestige of AI and its ability to develop successful drug candidates was struck a minor blow when a high profile AI drug development company’s lead prospect failed in late stage trials.
A fly in the ointment
BenevolentAI is a UK company that is among leaders in a wave of pharma companies that are developing pipelines of potential treatments through an AI platform. BenevolentAI’s platform was deemed promising enough that AstraZeneca signed a long-term collaboration with the company in 2019, and the two have already developed five targets. However, last month, the company faced a major setback when its lead drug candidate, BEN-2293, was unable to show improvements in the treatment of symptoms of atopic dermatitis (AD).
The drug candidate was selected through its AI technology platform, and is part of a group of treatments known as pan-TRK inhibitors. This type of treatment is approved to treat cancer, but BenevolentAI had pursued the potential for its use to relieve inflammation and related itching in AD. In phase 2a results for topical application of BEN-2293, the treatment was not able to meet its secondary efficacy endpoints to reduce itch and inflammation. The data showed that though the treatment was safe and well-tolerated, it was not able to demonstrate a statistically significant impact on the Eczema Area and Severity Index (EASI) or in the pruritus Numerical Rating Scale.
On announcing the results, BenevolentAI made it clear that it is not giving up on the potential treatment. The company identified a smaller percentage of the participants with more severe symptoms who showed a greater response to the treatment, opening the possibility to narrow the therapy indication down to this sub-set of patients. This was the conclusion reached by the company’s chief scientific officer, Anne Phelan, who stated on the findings: “Although the top-line efficacy findings are not conclusive, the significant relationship of EASI efficacy with affected BSA (body surface area) requires further investigation and supports the hypothesis of utilising a PanTrk inhibitor in AD. We will continue to review and analyse the complete dataset in order to fully understand the outcome and the next steps.”
A question of investment
As one of the few companies to have progressed AI-generated drug candidates through to clinical studies, the failure to show efficacy and to have a clear road through to phase 3 trials means that there will be an inevitable impact for AI-based, drug discovery companies. Setbacks in developing fields can discourage investment. This is particularly true in the current financial climate, with high inflation and global economic uncertainty leading to a more cautious approach from sources of capital to the area.
In a recent analysis by Morgan Stanley, Vikram Purohit, an analyst, identified that the next two years will be crucial for the area. This is driven by the number of readouts that are expected, as AI-developed drugs make their way through the pipeline. In a statement by Purohit, they said, “If initial readouts are consecutively strong, we believe stocks across the space could rise as investors gain confidence in a well-defined total addressable market for AI-enabled drug development.”
The inverse of this statement is also true. This is why the next readouts in the field are especially important, as it could see investment either dwindle or boom. Proving the reliability of the technology will be crucial to address this.
The importance of data
Investment interest is held on the basis that AI drug discovery could make the process more consistent, but that will require continued development of the technology. In a paper to understand the potential and challenges facing AI drug discovery, the authors identified the major barrier for the field to be the availability of suitable data. In particular, the research honed in on the fact that AI-based approaches require a large volume of information to be trained. This means that low quality or inconsistent data can affect the accuracy and reliability of results. As a result, the authors observed that, at present, “current AI-based approaches are not a substitute for traditional experimental methods, and they cannot replace the expertise and experience of human researchers.”
Harvard Medical School biomedical informatician, Marinka Zitnik, recently launched a platform that was created to address exactly this problem of data quality. The aim is to develop more realistic data sets and higher-fidelity algorithms to make the models translatable into real-world scenarios. Currently, Zitnik stated, there are new AI models that are emerging and being published that suggest near-perfect accuracy, but that this is contradicted by the not-as-yet widespread adoption of machine learning in drug discovery.
“This is because there is a big gap between performing well on a benchmark data set and being ready to transition to real-world implementation in a biomedical or clinical setting,” Zitnik explained. “The data on which these models are trained and tested are not indicative of the kind of challenges these models are exposed to when they’re used in real practice, so closing this gap is really important.”
What will close that gap is increased investment into the space. This has already developed rapidly in the preceding years, as big pharma has started forging drug development relationships. However, for the area to really take-off, and for the companies involved to gain the milestone investments backloaded into such deals, it will require a company to successfully progress an AI-developed drug into a position to be commercialised. BenevolentAI’s struggles with its lead candidate are a blow to this, but there are many more prospects coming through the pipeline, including within that company’s own portfolio. With Morgan Stanley predicting that AI drug discovery represents a $50 billion opportunity, with the potential to bring through an additional 50 novel therapies over a 10-year period, the long-term incentive exists. The question will be whether the interest in investment continues in the short-term if the coming data readouts in the next two years highlight that this approach is still no magic bullet for drug development.