Revolutionising clinical trials: The promise of AI-driven subgroup analysis
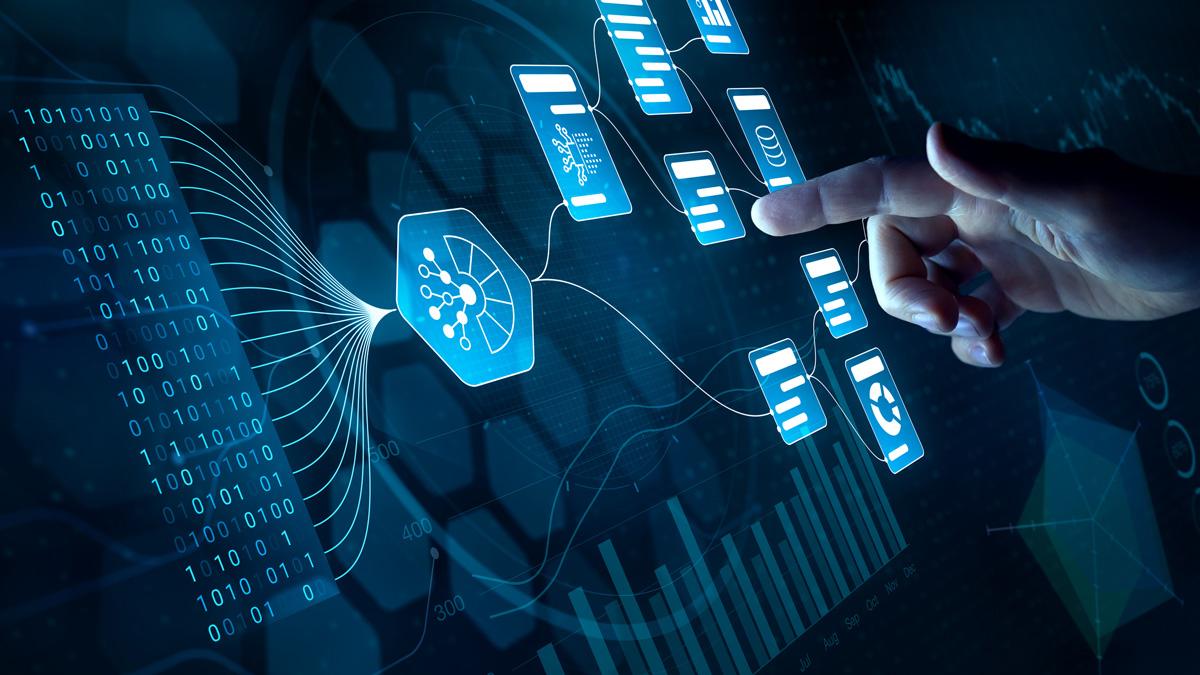
In the pharmaceutical research field, the journey from laboratory to pharmacy shelf is fraught with challenges, such as the high failure rates and increasing costs of clinical trials. Significant money is spent. For example, PhRMA reports that its members spent almost $101 billion in research and development in 2022, and the effectiveness of this research can affect millions of lives in terms of bringing potentially lifesaving therapeutics to market.
With only 12%1 of therapies surviving the gauntlet of Phase III trials to receive FDA approval, and the cost to bring a drug to market soaring beyond the billion-dollar mark, the need for innovation in clinical trial methodology has never been more pressing. Sponsors are seeking to improve how clinical trials are conducted to make drug development more efficient, focused, and effective.
This drive for improvement has seen the emergence of Sub-population Optimisation & Modelling Solutions (SOMS), a new approach to clinical trial methodology and analysis that harnesses the power of artificial intelligence (AI) and cutting-edge analytics to uncover predictive biomarkers within distinct patient subgroups. By identifying these markers, SOMS enables researchers to pinpoint patient populations with higher efficacy or risk for adverse events, significantly boosting the odds of trial success.
Unveiling the power of AI in patient subgroups
SOMS' AI-driven subgroup analysis is transforming clinical trials through real-time data processing and continuous tracking of patient subgroups. This sophisticated system can simulate trials using real-world or simulated data to predict outcomes based on patient pool characteristics. It also offers benchmarking capabilities, comparing potential performance against standard treatments or other therapies in the same therapeutic area.
The technology's power lies in its ability to analyse multiple variables simultaneously, evaluating exponential permutations to identify optimal patient subgroups. This dynamic, data-centric approach uncovers patterns and correlations that human researchers might overlook, potentially identifying subgroups more likely to respond positively to a treatment or face increased risks of adverse events.
SOMS processes data rapidly, generating analyses within seconds for smaller trials or hours for larger datasets. Its flexibility allows researchers to modify criteria as needed, ensuring repeatability across trials within a portfolio. This transformative tool is helping to turn the tide in favour of researchers and patients alike, redefining how we approach clinical trials and moving beyond traditional methods that rely on predetermined variables.
Real-time insights, real-world impact
SOMS is proving invaluable in identifying and rescuing struggling studies by implementing rescue strategies for trials facing challenges such as unexpected adverse events or lack of efficacy. By swiftly analysing vast amounts of data, it can predict which subgroups are more susceptible to specific outcomes, allowing researchers to take targeted action.
A prime example of SOMS' effectiveness was demonstrated in a Phase III multiple myeloma trial. Researchers used the system to analyse 25 biomarkers, including demographic and disease characteristics. This analysis revealed two specific biomarkers indicating an increased risk of cardiac issues, enabling sponsors to focus on high-risk groups and implement protective measures.
The system's core strength lies in its ability to identify and optimise effective subgroups within a trial. It employs three variations of the Subgroup Identification Based on Differential Effect Search (SIDES) algorithm, allowing for high accuracy and configurability in patient subgroup identification.
SOMS's impact extends throughout the entire clinical trial process. In early phases, it can leverage existing data to simulate patient responses and inform trial design. During the critical transition from Phase II to Phase III, SOMS helps refine inclusion/exclusion criteria and identify responsive subgroups, optimising overall trial design and increasing the chances of success.
The system's value proposition goes beyond just rescuing struggling trials. By enhancing the quality and relevance of recruited patients, SOMS promotes a "fewer but right" approach that can lead to better long-term outcomes. In one notable case, SOMS analysis of 26 biomarkers in a Phase III antibacterial treatment trial identified a subpopulation with a strong enough response to secure FDA approval, potentially saving hundreds of millions of dollars in development costs.
Navigating the ethical landscape
As with any powerful technology, the use of AI in clinical trials raises important ethical considerations. How do we ensure transparency in AI-driven decisions? How can we safeguard patient privacy while leveraging vast amounts of personal health data? These are critical questions that the industry must grapple with as it moves forward.
The key lies in developing robust frameworks for "explainable AI" – systems that can not only make predictions, but also provide clear rationales for their decisions. This transparency will be crucial in maintaining trust among patients, regulators, and the broader medical community.
A new era of personalised medicine
As we look to the horizon, the potential of SOMS and similar AI-driven technologies in clinical trials is boundless. Future developments may include integration with data management systems for real-time analysis, incorporation into risk-based quality management tools, development of specialised algorithms for specific therapeutic areas and AI-assisted protocol design, and patient recruitment.
This technology heralds a new era in personalised medicine, where treatments can be tailored to specific patient subgroups based on a complex interplay of biomarkers, genetic factors, and other individual characteristics. The implications are profound, potentially offering patients access to more effective treatments with fewer side effects, while providing the pharmaceutical industry a path to developing drugs that might otherwise have been deemed failures.
The road ahead
SOMS represents a fundamental shift in clinical trials. The integration of AI-driven subgroup analysis promises a fundamental reimagining of how new treatments are developed. Reducing failure rates, cutting costs, and accelerating innovation will drive more targeted drug development that reduces the time of bringing new treatments to market while improving patient outcomes and accelerating medical progress.
However, it's important to note that SOMS' impact depends on various factors, including specific trial findings and how researchers act on the information provided. Not every trial will have clear subgroup distinctions, but, when patterns exist, SOMS excels at finding them.
As the pharmaceutical industry continues to embrace AI-driven solutions, tools like SOMS are set to play an increasingly crucial role in streamlining the drug development process. The future of clinical trials is data-driven, AI-powered, and more promising than ever before, driven by the enduring human desire to heal, innovate, and push the boundaries of what's possible in medicine.
Reference
- https://www.ncbi.nlm.nih.gov/pmc/articles/PMC9293739/