Combatting longstanding challenges in rare disease detection with innovative deep learning models
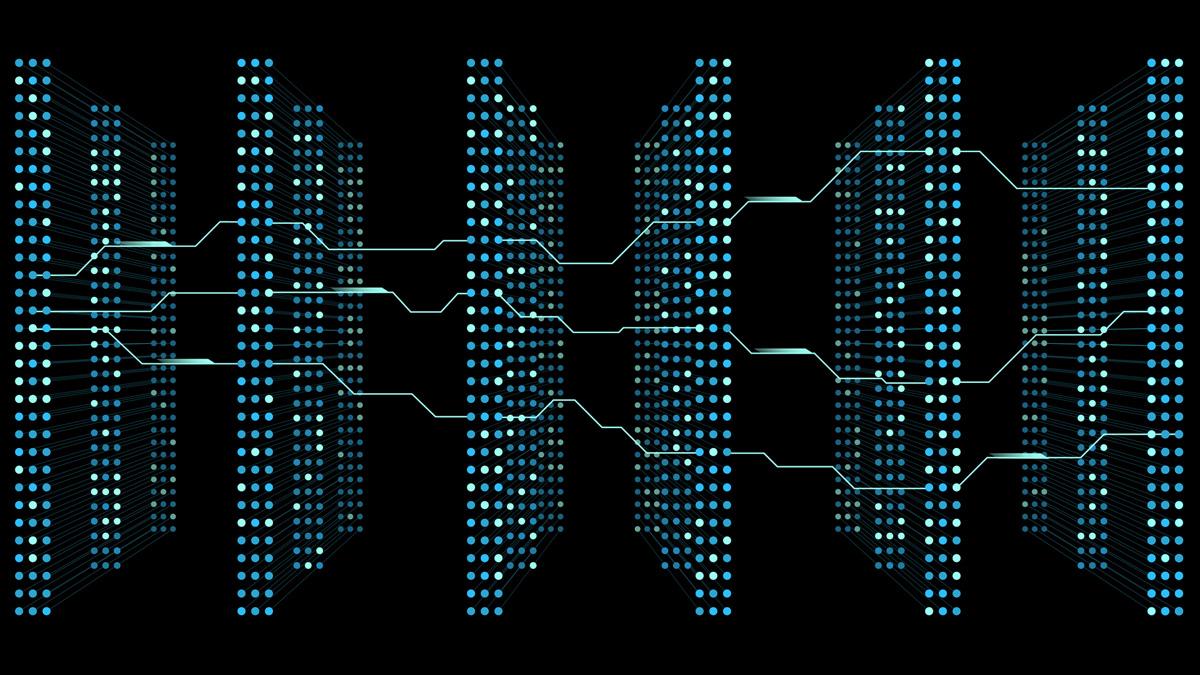
Approximately 350 million people are living with up to 8,000 rare diseases worldwide. For perspective, imagine the entire population of the US and millions more, including children, debilitated by diseases and often unable to receive optimal care due to underdiagnoses or initial misdiagnoses.
The average time for accurate diagnosis of a rare disease is four to five years.1,2,3 In some cases, it can take more than a decade.4,5 Unfortunately, this greatly delays patients’ access to effective treatment options and increases the financial burden on them and their families. According to a recent National Institutes of Health study analysis, people with rare diseases can have up to five times the healthcare costs of those without a rare disease.
Though hundreds of millions of people globally are living with rare diseases, prevalence rates are significantly low, varying anywhere from one in 1,000 to one in 20,000 patients, given such high levels of underdiagnoses and misdiagnosis. It is not only challenging for patients and their loved ones, but also for clinical trial sponsors and their clinical research organisation partners trying to plan and execute clinical trials to further examine these diseases and potential treatments. Rare disease clinical trial design poses several unique challenges to sponsors and study teams, including:
- Inability to define meaningful endpoints, given limited knowledge and history of rare diseases and their progression.
- Identifying patients to engage for trial participation.
- Ensuring enrolled patients accurately represent the target patient population.
- Small sample sizes.
Through advances in machine learning and deep learning methodologies, sponsors can leverage expansive datasets available in today’s broader healthcare system (e.g., genomic sequencing data and electronic health records) to equip themselves with the right expertise to improve rare disease detection and accelerate much-needed trials.
Rare disease patient identification: The traditional AI approach
In recent years, by using tremendous amounts of EHR data, researchers have trained deep learning models to extract disease progression insights, but this predictive modelling has primarily focused on chronic and prevalent diseases, such as Parkinson’s disease or cardiovascular conditions, not rare diseases. It is understandable, because these models only include patients with a confirmed diagnosis, which is limiting and makes extracting disease patterns difficult.
Though there are many patients who for years have a question mark on their diagnosis due to the time it takes to accurately identify a rare disease, these individuals can be potentially beneficial in improving learning models’ performance, given their similarities to patients with confirmed diagnoses. But it is also challenging to apply these patients to learning models for rare disease detection without generating false positive cases, because there is a broader level of classification. Individuals with uncertain diagnoses may be healthy or have a similar disease, but not the specific rare disease being evaluated, making it difficult for learning models to distinguish between patients with the target rare disease and those with similar conditions.
To help address this concern, researchers have relied on generative adversarial networks, or GANs, which are ML-based models that can create synthetic patient data, aiming to secure a sufficient amount of data to make predictive insights on disease patterns. For rare disease detection specifically, the use of GANs has limitations that need to be considered:
- These models help generate synthetic data that is as realistic as possible, but often this data loses the nuances and subtle insights needed to accurately represent an actual patient with the rare disease. Pattern insights are not as refined as they need to be for rare diseases.
- Due to low prevalence rates, it can be difficult to sufficiently augment data based on the rare disease group alone.
- Often, GAN-based methods apply a discriminator to separate real from synthetic data, but it does not target rare disease detection in the process.
Finessing disease detection: Furthering pattern augmentation
Because there is such potential for rich — and needed — disease pattern insights to be gained from both identified and unidentified patients with a specific rare disease, advanced ML methodologies are being leveraged to dive deeper into extracted findings, supplementing what has been done through traditional GAN methods.
Complementing the GAN model for enhanced pattern augmentation, an ML model trained with the capability to detect and classify positive (for a disease) patients and negative patients improves rare disease detection. Additionally, enhanced deep learning models can be trained to better classify both generated and original embedding data to learn from treatments, procedures, and diagnoses contained within a patient’s electronic health record. Profiling an individual in this manner provides valuable input to predict whether others will later receive a rare disease diagnosis. The advanced models augment additional algorithms to secure and extract the complexities and nuances of patient-level insights from synthetic data that GANs cannot. By improving discrimination between healthy and identified rare disease patients, these models can help reduce false positive cases, allowing researchers to focus on the rare disease at hand and patterns of interest.
Phenotypic profiling for early disease detection
Through the multilayered structure of EHR data, beginning with patient information and followed by provider visit notes, including patient experiences and diagnosis, procedure and medication codes, along with claims insights, patient registries, clinical trial data, and more, data scientists can translate this information numerically to feed AI-based algorithms that transform data into patterns to learn from and build predictive insights for disease identification. Using advanced ML, including enhanced natural language processing capabilities, computational phenotyping approaches can be applied to develop a definition of an optimal patient profile. This level of profiling can not only help create the patient profile benchmark for a trial, but also provide insights to better define patient segments for trial recruitment based on phenotype through disease progression patterns, if there are multiple root causes. Equipped with targeted insights to support patient identification, sponsors can apply relevant country data sets to help find individuals who fit the desired phenotype for the trial.
Synthetic trials
A concept leveraged in clinical research that is used in other industries called “digital twin modelling” or “synthetic modelling” can also be beneficial in rare disease studies. Through a virtual model that is designed to model a real object (e.g., a patient), sponsors can simulate the types of responses a patient may have in different scenarios, allowing faster insight generation for less cost than working through actual patients alone. For the rare disease space, this learning model provides flexibility to secure meaningful insights when there is not sufficient data from identified patients with the rare disease (the real object). Within its use of real-world evidence guidance for drug development, the US Food and Drug Administration notes the potential use of synthetic control arms when there is an insufficient patient population size available for evaluation.
Going a step further, researchers also have access to a breadth of omics data (e.g., genomics, proteomics, phenomics, transcriptomics, etc.), making a holistic view of an unhealthy cell possible. Using computational methods to create digital twins of cells based on omics data is helping to provide predictive outcomes on cell reactions to molecules. This is the base of how sponsors can test the viability of billions of drug combinations using AI-driven solutions to gauge treatment paths that may address rare disease patient needs.
Necessary components for AI-effectiveness
For sponsors, it can be enticing to dive into what seems like limitless possibilities through AI-driven solutions for accelerated, personalised, and safe drug development for patients in need. As with any use of AI-driven methodologies and solutions, it is vital to ensure tech-enabled models and deep learning techniques used to address the long-standing issue of rare disease detection are based on the specific needs and goals of the rare disease program and related patient population.
These innovative methodologies must be underpinned by extensive domain experience, including therapeutic, clinical trial operations, data science, and technology that work together early in the trial design process to review the specific rare disease under investigation and collaborate for potential avenues of solutions that may make a difference. Also, ML methodologies to augment disease patterns and ultimately improve rare disease detection can only be as good as the breadth and quality of data available to extract meaningful insights from and how these datasets are structurally inputted into fine-tuned algorithms that get to the heart of what is needed to keep rare disease drug development moving forward.
It is through this rounded approach that deep expertise and advanced data patterns meet science-based tech solutions that enable sponsors to make a genuine change in rare disease drug development, providing reliable options to better uncover therapeutic solutions for rare diseases that impact millions worldwide.
References
- Global Commission on Rare Disease [Internet]. [cited 2021 Dec 6]. Available from: https://www.globalrarediseasecommission.com/Report
- Accurate Diagnosis of Rare Diseases Remains Difficult Despite Strong Physician Interest - Global Genes [Internet]. Global Genes. 2014 [cited 2019 Aug 21]. Available from: https://globalgenes.org/2014/03/06/accurate-diagnosis-of-rare-diseases-remains-difficult-despite-strong-physician-interest/
- Yan X, He S, Dong D. Determining How Far an Adult Rare Disease Patient Needs to Travel for a Definitive Diagnosis: A Cross-Sectional Examination of the 2018 National Rare Disease Survey in China. Int J Environ Res Public Health. 2020;17. Available from: https://doi.org/10.3390/ijerph17051757
- Molster C, Urwin D, Di Pietro L, Fookes M, Petrie D, van der Laan S, et al. Survey of healthcare experiences of Australian adults living with rare diseases. Orphanet J Rare Dis. 2016;11:30. Availabile from: https://ojrd.biomedcentral.com/articles/10.1186/s13023-016-0409-z
- Heuyer T, Pavan S, Vicard C. The health and life path of rare disease patients: results of the 2015 French barometer. Patient Relat Outcome Meas. 2017;8:97–110. Available from: https://pubmed.ncbi.nlm.nih.gov/28979171/.
About the authors
Greg Lever, director of AI solutions delivery at IQVIA, began his career in life sciences and technology more than 13 years ago. After obtaining his PhD at the University of Cambridge for his work combining quantum physics and machine learning to develop new approaches for small-molecule drug discovery, he worked as a postdoctoral associate at MIT. Shifting to industry, Lever was an integral part at several technology start-up companies in London and then joined Genomics England in the early stages of the 100,000 Genomes Project, seeing it through project completion. Currently, as director with the IQVIA Analytics Center of Excellence, he leads a team of expert ML engineers to help clients discover innovative ways to bring life-changing drugs and therapies to patients faster.
Lucas Glass is VP of the Analytics Center of Excellence at IQVIA, a team of over 200 data scientists, engineers, and product managers who research, develop, and operationalise machine learning and data science solutions within the R&D space. Glass has launched more than a dozen machine learning offerings within R&D, including site recommender systems, trial matching solutions, enrolment rate algorithms, drug target interactions, drug repurposing, and molecular optimisation. His machine learning research, which is dedicated to R&D, has been published by AAAI, WWW, NIPS, ICML, JAMIA, KDD, and many others.