Pharma and the ongoing battle against AI drift
As pharmaceutical companies increasingly rely on artificial intelligence (AI) to optimise trial processes, the potential for AI models to drift over time poses significant risks to trial integrity, patient safety, and the advancement of precision therapies. Indeed, ‘AI drift’ is a critical issue in the context of clinical trial design and patient recruitment, particularly when it comes to personalised medicine.
The employment of such technologies in life sciences is not new, of course. As The Economist reports, pharma “has used computational models for decades, but AI is changing drug discovery in several ways,” permitting analysis of huge quantities of disparate data and identifying promising molecules, while generative AI (GenAI) can conceive entirely new molecules to test. The publication also cited AlphaFold 2 and AlphaFold 3 as prime examples of developments in this latter respect, as well as mentioning AI posterchild start-up Insilico Medicine.
But what about when the AI gets it wrong?
On 1st August 2024, the European Artificial Intelligence Act (EU AI Act) entered into force, aiming to foster responsible artificial intelligence development and deployment in the EU. The Act introduces a uniform framework across all EU countries, based on a forward-looking definition of AI and a risk-based approach (minimal, specific transparency, high, and unacceptable).
Digital Frontier has noted the trans-global differences (or rather, similarities) in such AI monitoring, highlighting that the EU AI Act calls for a “post-market monitoring system” that “actively and systematically” collects, documents, and analyses data on the model’s performance, while the UK government’s Ethics, Transparency and Accountability Framework for Automated Decision-Making calls for continuous monitoring by decision-makers “throughout the lifecycle of the system.” Meanwhile, in the US, the proposed AI Bill of Rights under Outgoing President Joe Biden states that, “automated systems should have ongoing monitoring procedures, including recalibration procedures, in place to ensure that their performance does not fall below an acceptable level over time.”
To what end this ‘ongoing’ process of monitoring the machine, so to speak? Because over time, just like humans, machines can make errors. There have been questions as to whether OpenAI’s ChatGPT – a large language model (LLM) – has been ‘getting dumber’ of late, for instance. Known as ‘AI drift’, generative AI models, when data and context becomes historic to real-world scenarios, quite literally ‘drift’ from their original programming. And in the context of the life sciences, this can be very serious indeed.
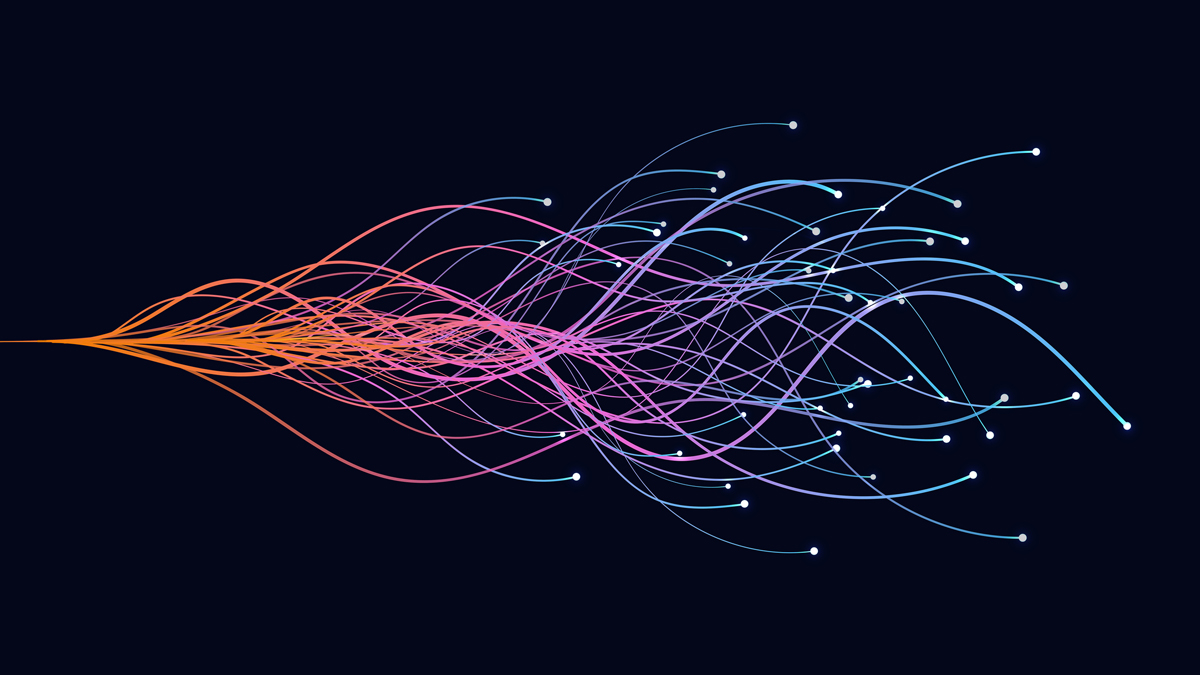
The dangers of drift within life sciences
The essential nature of the drift comes in two stages, according to Microsoft:
- Data drift – occurs when there is a change in input distribution that may (real drift) or may not (virtual drift) affect the class boundaries
- Model drift – occurs when an actual change in the class boundary occurs (i.e., real drift)
A study published in Nature, noted that it is less common for the input data to be monitored for data drift (systemic changes to input distributions) and found that monitoring performance alone is not a good proxy for detecting data drift; rather, that drift-detection heavily depends on sample size and patient features. Model drift, of course, does not occur if there is no data drift. But, when model drift does occur, it can be gradual or it can be sudden. Nonetheless, essentially, every model drifts. Gradual drift can be caused by changes in customer behaviour, the economy, or the law; sudden drift occurs when there is a major change in data distribution, such as a natural disaster or a terrorist attack, or – in the life sciences especially – a product recall. The severity of the drift is termed its ‘magnitude’.
AI drift in patient selection algorithms may result in various issues in the life sciences setting:
- Biased participant pools that don't accurately represent target populations: As AI models drift, they may inadvertently introduce or amplify biases in patient selection. This could lead to trial populations that don't reflect the diversity of real-world patients.
- Ineffective stratification of patients for personalised medicine trials: Precision medicine relies on accurate patient stratification. AI drift could lead to misclassification of patients into incorrect subgroups, potentially masking treatment effects or exaggerating differences between patient cohorts.
- Inaccurate predictions of trial outcomes and side effects: Drifting AI models might provide increasingly unreliable forecasts of trial outcomes or fail to anticipate important side effects. This could lead to poorly designed trials, inefficient use of resources, or even safety risks for participants.
AI drift is, for now then, what one might term a serious design flaw. So, when it comes to safeguarding against such a time, it is suggested that companies continuously test their AI, to ensure that their technology is operating in alignment with organisational standards – and in the pharmaceutical industry that means making sure that there is no threat to patients’ health and safety.
As Suresh Venkatasubramanian, professor of data science and computer science, professor of humanities, and interim director of data science at Brown University, puts it, “You can’t get the benefits of AI but treat it like word processing software […] Build systems that you can inspect, analyse, query, and know what they’re doing and not just rely on a black box to do it for you.”
In a sense, then, avoiding dangerous AI drift involves intermittently training the AI, just as a human employee would be required to undergo Continuing Professional Development courses for their role. Training, and putting in place an alert system, can similarly help to ensure that fallout from sudden or gradual onset AI drift has minimum impact on company operations. However, a co-authored 2023 paper from researchers at Northeastern University and Snap Inc cautioned against retraining the technology too frequently or too sporadically. Rather, the paper suggested a ‘fairness monitoring model’.
It is about adaptation, a behaviour shift for the technological workforce, as it were. Yet, model adaptation is often one of ‘remembering and forgetting’, or ‘blind global replacement’, which can be an expensive process. A better way is ‘informed adaptation’.
The monitoring of any AI drift is a learning process in itself for the developers of these advanced technologies, and it must be detected early, before erroneous information leaks into the system: this is where the nuance between drift detection and data validation come into effect, where ‘blind’ adaptation is swapped out for ‘informed’, via online incremental learning algorithms that update the model upon arrival of each data point, as Microsoft distinguishes it.
As BioProcess International notes, “AI and ML are proving to be excellent assistive tools for such activities – and are uniquely capable of enabling such initiatives as Pharma 4.0”, referring to the application of Industry 4.0 principles and technologies to pharmaceutical manufacturing, aiming to improve product quality, patient safety, operational efficiency, and innovation, while simplifying compliance and regulatory oversight.
As has been noted, such technologies have their limitations, though, as well as their risks. While it is often said that the ‘human in the loop’ is the caveat that keeps us safe, if we return to the concern over bias, such issues derive from the very human programmers’ training of AI, often with samples not fully representative. And in the life sciences, it is paramount that the diversity of humanity be understood, for machine and human both.
So it is that technologies like the Linux Foundation’s Open Neural Network Exchange (ONNX) program have been developed, standardising deep-learning models and helping to alleviate concerns about the “black box” perception. As BioProcess International also highlights, “faulty design, misapplication, neglect of control, and improper operation could compromise AI’s use” – but this is the case with any such tool.
Thus, rather, standardisation becomes the crucial factor; for example, as through the FDA’s ALCOA+ principles. The term ALCOA stands for ‘Attributable, Legible, Contemporaneous, Original and Accurate’, while ALCOA+ adds ‘Complete, Consistent, Enduring and Available’. Put in place in 2018 for companies handling data under 21 CFR Part 11, it is a regulatory framework issued by the FDA that guides drugmakers on how to create, manage, and maintain electronic records and electronic signatures.
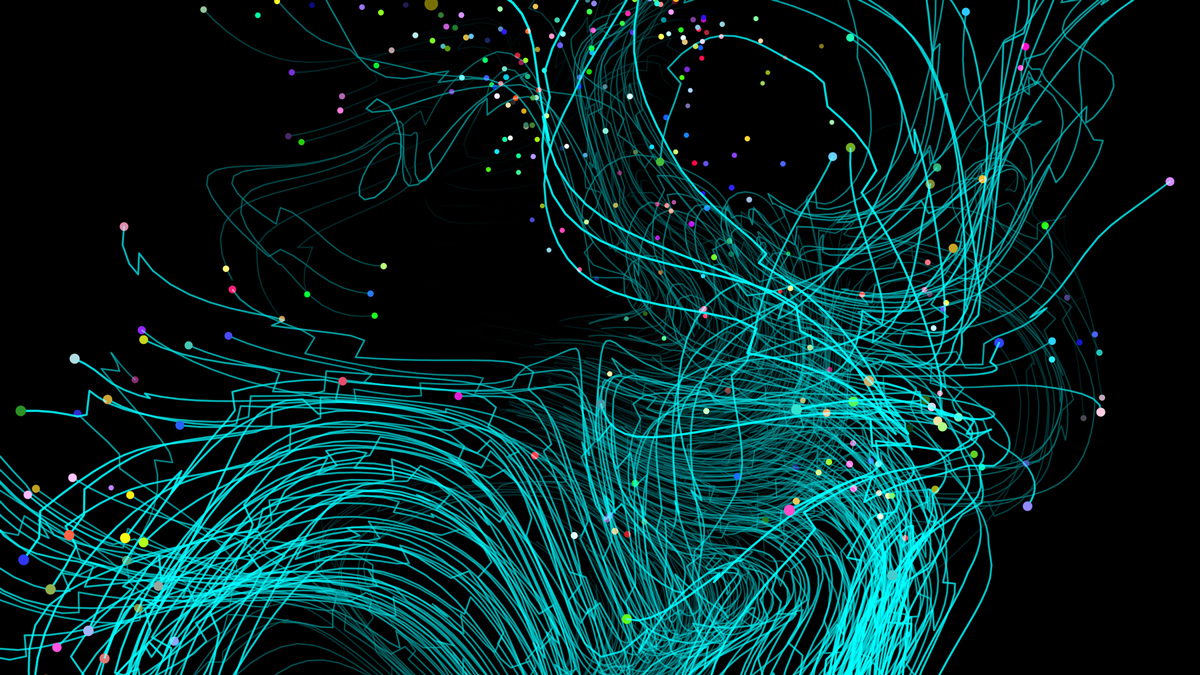
The hope for synthetic data
As argued above, all advanced technology programs require monitoring to ensure that they continue to function and perform as originally intended – but, like a parent, adaptive guidance is needed along the life course of those technologies, monitoring and training the algorithms to stay current with an ever changing world. And advanced techniques for data explainability, transparency, and security are helping biopharma in this regard – especially when it comes to demonstrating value.
Yet, what could assist in prevention against AI drift is synthetic data, data which has been artificially created. As Techopedia reports, synthetic data is cost-effective, avoids bias, so too privacy concerns, and permits multiple scenario testing. There are still ethical and ‘real’ considerations, of course, but in the context of clinical trials it permits accelerated processes. The hesitation in its use, though, is that even if synthetic data is used, if the generation algorithms themselves are biased, then synthetic data can amplify that bias further.
No panacea, then, it seems at the moment standardisation, monitoring, continuous training for real-world applicability, and adaptation are the necessary prescription for protection against AI drift – just like any ‘seasonal’ bug.
About the author
Nicole Raleigh is pharmaphorum’s web editor. Transitioning to the healthcare sector in the last few years, she is an experienced media and communications professional who has worked in print and digital for over 18 years.
Supercharge your pharma insights: Sign up to pharmaphorum's newsletter for daily updates, weekly roundups, and in-depth analysis across all industry sectors.
Click on either of the images below for more articles from this edition of Deep Dive: AI 2024