A strategic framework for evaluating AI in drug discovery
Artificial intelligence (AI) has emerged as a transformative force in the life sciences industry, showcasing its exceptional ability to process large-scale datasets, uncover patterns, and generate predictions.
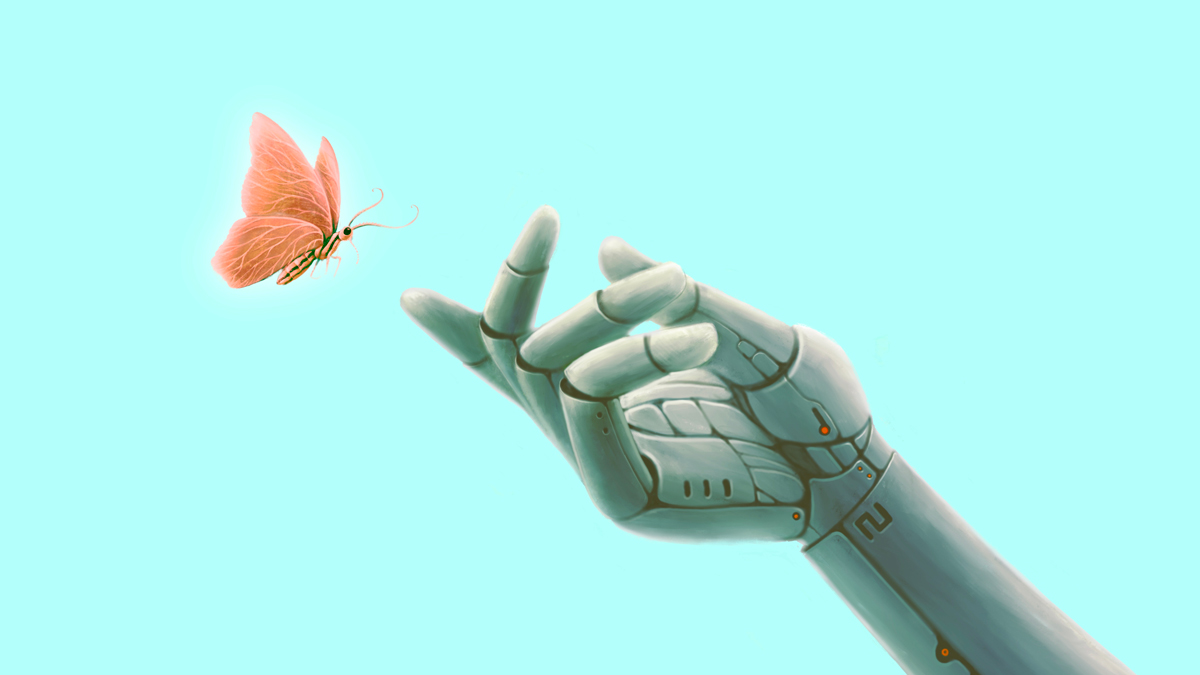
Its applications are transforming the field, bringing efficiencies to drug discovery, improving clinical trial processes, and enhancing patient treatment strategies. These technological breakthroughs promise not only to revolutionise the development of life-saving treatments, but also to influence strategic planning within life sciences companies.
A framework for measuring AI maturity
In this article, we present a framework designed to evaluate the progress and impact of AI tools across various use cases in drug discovery. This framework helps organisations better understand the current status of their AI initiatives and their potential to drive meaningful value.
It can also help to assess the stages of development and deployment of AI solutions, offering organisations clear insights into the readiness and future potential of their AI efforts. The framework measures AI maturity through five key criteria:
- The right problem: Determines the suitability and advantages of AI solutions over traditional approaches
- The right data: Evaluates current data availability, accessibility, and quality
- The right capabilities: Examines the available tools, platforms, and expertise required for effective execution
- The right market configuration: Considers the number of active players and the extent of collaboration interest from pharmaceutical companies
- Demonstrated impact: Evaluates AI's ability to generate novel insights and identifies tangible successes achieved thus far.
By addressing these dimensions, organisations can measure the maturity of their AI capabilities and optimise their strategies to unlock its full potential.
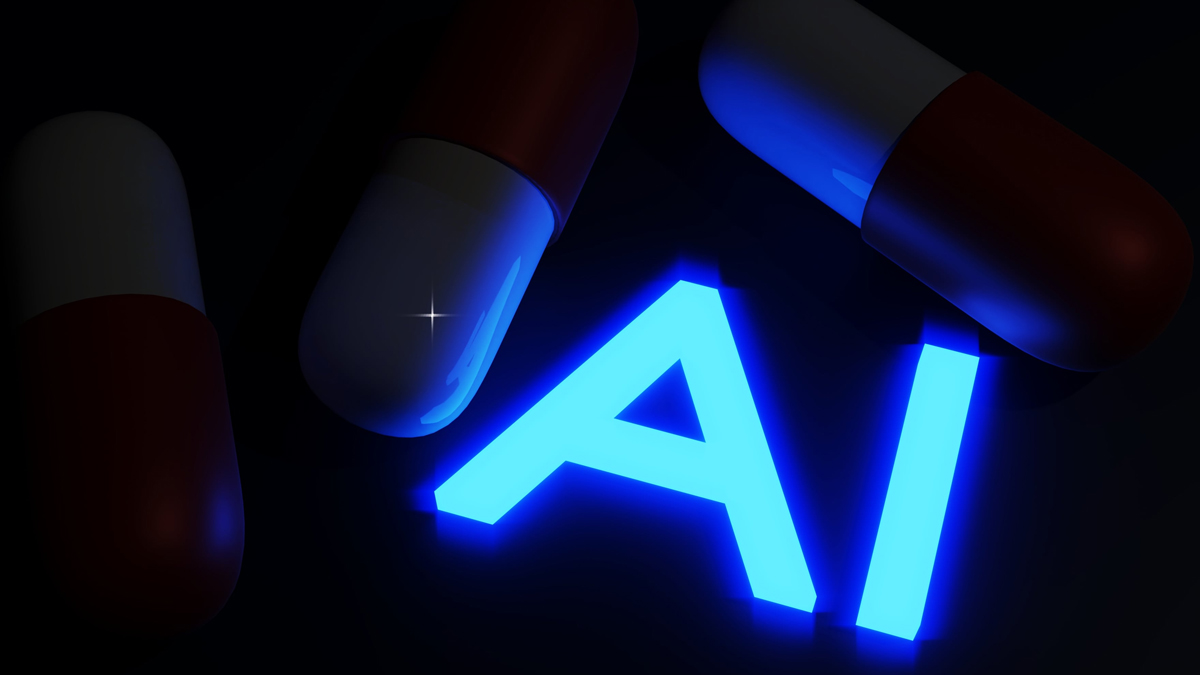
Key applications of AI in drug discovery
There are many applications of AI in drug discovery. In this article we have chosen to focus on four to illustrate how the framework can be used to assess AI maturity.
1. Repurposing existing drug candidates:
One of the most effective applications of AI in drug discovery is repurposing existing drugs. This approach allows AI to quickly identify new therapeutic uses for existing compounds. More than 250 companies are currently using AI in this area. For example, Healx, a UK-based company specialising in rare diseases, used AI to repurpose existing drugs for new therapeutic applications.
In this case, Healx repurposed cimetidine, a drug originally designed to treat stomach ulcers. By leveraging AI, Healx identified cimetidine as a promising treatment for fibrodysplasia ossificans progressiva (FOP), a rare and debilitating genetic disorder.
Although many AI-identified compounds are still under evaluation, the future looks optimistic. As datasets become richer and more accessible, this area is likely to see significant growth.
2. Identifying drug targets:
AI has proven highly effective in building disease models and identifying drug targets or biomarkers more efficiently than traditional techniques. It can process vast amounts of biomedical data, but integrating unstructured datasets continues to pose challenges. AI excels at extracting insights from diverse sources such as academic journals, omics databases, medical imaging, and real-world patient data.
Tools like knowledge graphs have been pivotal in uncovering new relationships between entities. However, their effectiveness depends on the standardisation and labelling of input data. To date, at least 20 drugs with disease-target associations identified through AI are in Phase 1 or 2 trials. By expanding datasets and refining algorithms, companies expect to discover more innovative and entirely novel targets in the coming years.
3. Designing small-molecule drugs:
AI has revolutionised small-molecule drug design by simulating complex chemical properties and generating drug structures with unprecedented speed and accuracy. In this use case, AI tools can either screen chemical libraries or create entirely new compound designs.
A key limitation remains the size and scope of training datasets, which are small compared to the billions of possible compounds in chemical space. Additionally, data availability differs by target class. For example, kinases and G protein-coupled receptors are better characterised, which limits generalisable models and the novelty of resulting drug candidates.
Nonetheless, AI tools for small-molecule drug design have become a cornerstone of the development process. Predictive solutions are continually improving, and AI-designed small molecules outnumber AI-designed antibodies.
Companies such as Exscientia/Recursion and Insilico Medicine are leading efforts in this space, with AI-designed small molecules now in Phase 2 clinical trials. These trials are expected to provide valuable insights into the maturity of AI technologies in drug design.
4. Designing antibody drugs:
AI is gaining momentum in antibody drug design, focusing on optimising current structures and creating new candidates. Compared to small molecules, designing antibodies is more complex due to the computational demands of working with larger molecules.
One major obstacle is the lack of sufficient datasets for antibody sequences and interactions. A significant portion of training data is derived from conventional antibody design libraries, perpetuating challenges like balancing specificity and affinity.
Despite these difficulties, interest in AI-driven antibody design is growing. Over the past year, numerous pharmaceutical companies have disclosed partnerships with start-ups or introduced internal AI capabilities. Notably, Xaira Therapeutics secured over $1bn in funding to focus on de novo antibody design, employing experts in generative modelling and genomics.
This influx of resources and expertise is expected to drive the maturity of AI applications in antibody drug design, enhancing the potential for novel therapeutic candidates.
Generative AI marks a significant advancement for the life sciences sector. This technology autonomously creates new molecular structures and other complex data, presenting exceptional opportunities for both cost savings and innovation when compared to traditional predictive AI approaches.
Although challenges, such as data availability and algorithm optimisation, remain, innovation and collaboration continue to drive progress. As AI becomes increasingly integrated into life sciences, it is set to unlock new possibilities in problem-solving and deliver transformative results across a range of applications.
By embracing AI's full potential, the life sciences industry can significantly accelerate therapeutic innovation, making advanced treatments more efficient and cost-effective. The future of AI in this field is poised to transform healthcare and drive the next generation of medical breakthroughs.
About the authors
Anne Dhulesia
Anne Dhulesia is a Partner in L.E.K. Consulting’s London office and a member of the Life Sciences European practice. She advises clients on a wide range of assignments in the sector (from biopharma to pharma service providers), including the identification of business development opportunities, business plan development, market potential assessments and the definition of long-term strategies.
Within biopharma, Dhulesia advises clients on a range of topics: BD, R&D, and commercial. She has a PhD in Chemistry from the University of Cambridge: her work focused on protein structure and protein folding.
Stephen Roper
Stephen Roper is a Partner at. L.E.K. Consulting and a member of the firm’s healthcare practice. He focuses on the life sciences sector and has extensive experience in strategic and transaction-related assignments for pharma, biotech, and diagnostics companies, as well as contract services providers and investors.
Roper advises clients on growth strategy, opportunity assessment, revenue forecasting and valuation, indication prioritisation, commercial due diligence, and acquisition screening. He has a particular interest in the applications of emerging big data and AI-powered tools in supporting pharma R&D
Supercharge your pharma insights: Sign up to pharmaphorum's newsletter for daily updates, weekly roundups, and in-depth analysis across all industry sectors.
Click on either of the images below for more articles from this edition of Deep Dive: AI 2024