Improving trial predictability: leveraging AI-powered analytics to guide trial design, strategy, and operations
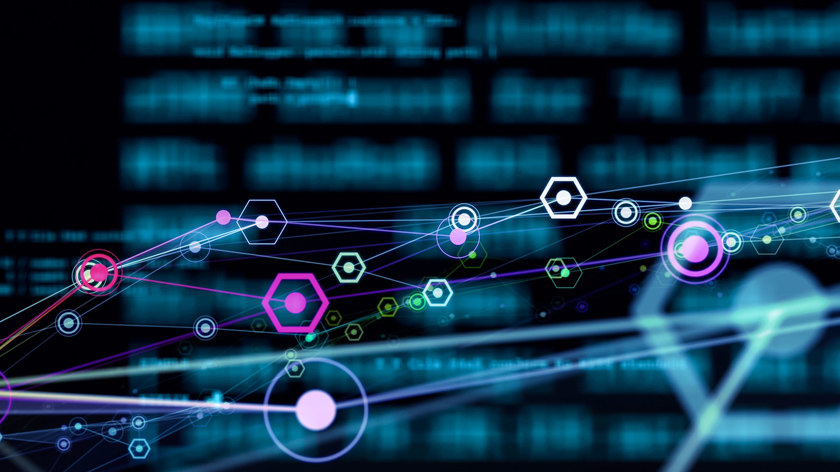
Enrolling for clinical trials has become more challenging in recent years, with trial competition, increasingly complex protocols, and precision medicine targeting more niche patient populations. This emphasises sponsors’ need for enrolment predictability. The challenge the industry faces, however, is that operational certainty is exacerbated by the impact of global disruptions, such as the effect of COVID-19, natural disasters, and regional conflicts. These problems are compounded with site-level concerns about protocol complexity, staffing shortages, low patient interest, and budgetary constraints — leaving sponsors to question how to plan trials that deliver against timeline commitments to support launch goals.
Using data-informed insights derived by effectively implemented datasets, artificial intelligence/machine learning capabilities, and technology sponsors can realise increased predictability in their study planning from protocol design through enrolment strategy.
Critical analysis in protocol design
Nearly eight out of 10 (78%) phase II protocols and 69% of phase III protocols have at least one substantial protocol amendment, and they average 2.7 and 3.3 per protocol, respectively. It’s estimated that pharmaceutical and biotechnology companies spend between $7 billion and $8 billion ($US) annually to implement protocol amendments.* Previous research showed that 23% of these amendments were considered “completely avoidable” because they were due to protocol design flaws, inconsistencies, or errors.† Protocol design factors leading to operational challenges include design consistency, patient burden, site burden, study complexity, and eligibility criteria, including diversity and inclusion requirements. These potential study risks can lead to costly deviations and amendments, enrolment difficulties, and reduced patient compliance.
AI-powered analytics, which leverage a wealth of real-world data, including electronic health records, claims data, public data sources, and disease registries can help sponsors proactively navigate ambiguities in trial design, fine tune study parameters, and evaluate scenarios to optimise design decisions and mitigate potential risks. Analytics help extract meaningful patterns from the data, such as how study design procedure specifications differ from real-world patient clinical care or how they influence patient engagement differently based on race and ethnicity. These insights allow sponsors to identify opportunities to decrease assessment frequency or eliminate non-core procedures, thus reducing costs, operational complexity, and patient burden before protocol finalisation and study execution.
Country expansion to support enrolment
Beyond developing trial strategies and optimising protocols to avoid amendments and mitigate other risks, sponsors need insights to support enrolment strategy. Historically, sponsors and clinical research organisations alike have depended on core countries and sites for conducting their studies because of their predictability and reliability. However, in certain cases, this led to an overdependence on a subset of known countries and top-performing sites. With the acute impact of the pandemic on site resources, patient interest, and increased study complexity many sites reported capacity constraints. Additionally, the industry is experiencing site and investigator burn out, and sponsors have had to mitigate unforeseen global risks.
As a result, sponsors must adapt by employing a more diversified approach to country strategy development. Both sponsors and CROs will need to invest in expanding their predictable pool of countries and sites to support future enrolment strategies. Ultimately, this will also yield benefits for broader industry goals, such as enhancing diversity in trials, experience with new therapies, and patient access to medicines.
AI-driven insights generated by global data will lead the way in developing strategies built on standard of care, trial competition, regulatory requirements, and disease prevalence. Even as enough new data is captured, the industry will need to reduce its reliance on historical performance. In the interim, near-term trends can be used to support site and enrolment predictability. When possible, both historical and recent performance trends can be simultaneously assessed, creating real-time data and feedback loops that enable the models and increase predictability.
With a fresh take on a data-driven trial strategy, sponsors can create a new path for trials that also accounts for potential risks. These predictors can help sponsors better understand trade-offs of various trial planning scenarios and make more informed decisions for individual trial needs and goals.
Integration of data is key
The integration of trial planning and execution data will be a key win for clinical development. When planning and execution are aligned to the same data points and trend analysis, sponsors and CROs will be able to adjust strategies more seamlessly, with more flexibility and quickness to respond to potential risks. Integrating real-world data and healthcare treatment trends at the site level can mitigate challenges throughout the lifetime of the study. Studies are often planned months before enrolment begins, and the environment can change dramatically during that time.
Those changes, coupled with an inability to quickly adapt, negatively impact enrolment, which is partially why nearly half (48%) of sites miss their enrolment targets. This is especially evident in oncology studies that require failure of a prior therapy for eligibility. If the therapy has been recently approved for use and launched, then uptake and patient availability will vary by site. Using real-time data to track the trends at the physician level can be critical for successful enrolment in these scenarios.
AI-powered analytics are not intended to guarantee successful trials, but extracting meaningful insights is key to helping sponsors make more informed decisions to improve trial outcomes. Understanding how each stage of drug development ties together and how actionable data provides stakeholders with a closer look at protocol design and trial strategy analytics, we can predict the possible impacts in terms of cost, timelines, site, and patient experiences and more. Helping take the guesswork out of the process gives sponsors reason to be more confident in decisions made to optimise success from trial design through operationalisation.
*Getz, Kenneth. Pharmaceutical Outsourcing. March 2022. Accessed at: https://www.pharmoutsourcing.com/Featured-Articles/584137-Doubling-Down-on-Protocol-Amendments-and-Deviations/
†Ther Innov Regul Sci. 2016; 50(4):436-441; Tufts CSDD Impact Report. 2016;18(1).
About the authors
Natalia Kotchie, vice president, R&DS Applied Data Science Center, IQVIA
Natalia leads the Applied Data Science Center (ADSC) for R&D Solutions at IQVIA, which is responsible for data and analytics to support clinical trial strategies, including trial design and feasibility. ADSC is focused on creating data insights and supporting their translation to enhance study operations. The team also develops new global data-driven machine learning and artificial intelligence technologies to address clinical development challenges, focusing on developing solutions which drive greater efficiency, increased predictability, reduced timelines, and value for sponsors.
Cara Willoughby, senior director of design analytics, R&DS Applied Data Science Center, IQVIA
Cara leads global delivery of design analytics services, including the Data-informed Protocol Assessment (DIPA) solution leveraging IQVIA Connected Intelligence™ to pressure test protocols. Cara has 25 years of pharma, consulting, and CRO experience in clinical research, including combining applied data, analytics, and novel technologies to support planning and design trade-off decisions, protocol optimisation, regulatory submissions, medical writing, developing and implementing international data interchange standards, and leading strategic transformation and modernisation initiatives.
Monica Goins, senior director of feasibility analytics, R&DS Applied Data Science Center, IQVIA
Monica is the head of IQVIA’s Feasibility Analytics team. This team specialises in a variety of analytical assessments augmented by real-world data and historical trial data, such as competitive landscape analysis, country algorithms, and enrolment rate analysis, that drive trial planning and trial strategy decisions. Monica has 14 years of clinical research experience extending across the industry in the CRO and site-based knowledge areas.