Breathing life into analytical data
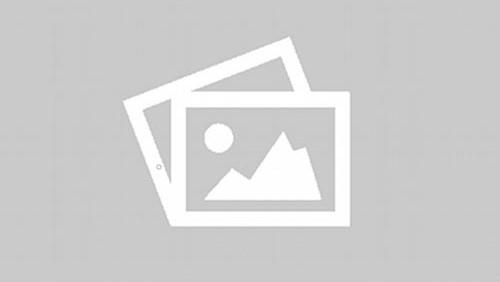
Ryan Sasaki of ACD/Labs explores how we can breathe more life into analytical data in the drug development environment.
A recent survey conducted on CIOs, Global Heads of R&D, QA, QC, and Principal Scientists in the laboratory informatics industry indicated that the main driver behind investment in laboratory informatics solutions is better data management. This is not surprising as data management issues are still highly prevalent within the informatics industry despite the emergence and reasonable level of adoption of new technologies in ELNs, LIMS, SDMS, etc. Interestingly, a 2011 survey of R&D professionals stated there was a sufficient lack of adequate systems to automatically collect data for reporting, analysis, and decision-making.
The term "Big Data" has been making headlines and dominating many of the discussions in laboratory informatics. In the context of this, the question arises: Are some of these reported issues related to the storage and management of large amounts of data? Or is that organizations are not investing enough time and resources in getting more out of the data they are generating?
To answer these questions we must first define the term data management in the pharmaceutical industry. What is data? What type of data are we talking about? There is a huge variety in the types of data that are being generated in laboratories across the globe; these different types of data are answering very different questions with varying degrees of complexity. So while there may be a need for a global data management solution to capture all data and metadata generated in a laboratory environment so it can be leveraged for making better decisions, the bigger need lies in the organization of data in sub-disciples. As Thessen and Patterson said, "data cultures in life sciences are very heterogeneous, and no single approach can suit the needs of everyone. The most successful strategies are those that address needs in the context of sub-disciplines."
As an example, let's look at the generous amounts of analytical data that are being generated in the drug development environment for the purposes of impurity identification and characterization. Regulatory bodies such as the U.S. Food and Drug Administration (FDA) and the International Congress on Harmonization (ICH) have established rigorous guidelines for the identification of extraneous compounds in pharmaceutical agents throughout the development process and post-marketing. During the New Drug Application (NDA) submission process, a list of impurities and a summary of the laboratory studies conducted for detection must be shared, which can include representative chromatograms, impurity profiles consisting of chromatograms of representative batches for analytical validation studies, along with complete impurity profiles of individual batches. In addition, Mass Spectrometry (MS), Nuclear Magnetic Resonance (NMR), and Optical Spectroscopy (OS) data will be generated as a means to characterize the chemical structure of unknown impurities. This adds up to mountains of data that are generated over a period of years. Preparation of progress reports, presentations, and especially an NDA can be very time-consuming, and as a result, quite costly. It is not unusual for days to be spent searching and compiling for the results of a series of specific analytical tests. The most common substitute for this is the costly repeat of experiments and tests, and the generation of new data.
One of the major inefficiencies that significantly increases the time and cost of pharmaceutical drug development is the traditional one-and-done life cycle where knowledge is captured from data, and essentially frozen as 'dead' data in unstructured formats and with none of the context preserved. Emphasis needs to be placed on strategies to capture that data in a standardized format and to put it into chemical context. In other words, breathing life into analytical data to capture analytical content (what) with chemical and reaction-based context (why) in a drug development environment serves as a strong foundation for creating intelligence-from-information. In order to enable such a transformation, 'live' data must be supported with some interpretation that applies context to the analytical data such as chemical structures, mixture components, or reaction-schema.
Improving the overall productivity of drug development and acceptance requires a combination of strategies, but even moderate improvements can substantially increase returns. To put this "mantra" into applied context, a recent FDA letter to industry reported that 54 percent of drug shortages were due to quality issues. Impurity identification and characterization is usually a time critical undertaking often linked to project milestones, such as IND and NDA filings. In addition, groups tasked with the responsibility of isolating and identifying impurities typically require a capital investment of millions of dollars in hardware, and individuals highly skilled in the utilization of the equipment and interpretation of the data.
It stands to reason that the way analytical data is best managed and leveraged in a drug development environment is drastically different than the way clinical or assay data should be managed and leveraged, for example. Therefore, efforts and resources should be spent on identifying how different types of data in different disciplinary areas of the organization can be best captured and leveraged to assist in everyday decision-making that can lead to moderate improvements and substantial returns.
References
1. Building a Smartlab and Optimizing Efficiency in 2013. 2013 Smartlab Exchange Infographic.
2. R&D Informatics: Are you ready for 2012?, http://blog.idbs.com/2011/10/18/rd-informatics-–-are-you-ready-for-2012/, IDBS (October 18, 2011).
3. Anne E. Thessen and David J. Patterson, Data issues in life sciences, PMC (NIH/NLM), http://www.ncbi.nlm.nih.gov/pmc/articles/PMC3234430/, November 28, 2011.
4. Ryan Sasaki and Bruce Pharr, Unified Laboratory Intelligence White Paper, February 2013.
5. Ryan Sasaki and Bruce Pharr, Unified Laboratory Intelligence for Impurity Resolution Management, October 2013.
6. Eric David, Tony Tramontin and Rodney Zemmel, The Road to Positive Returns, Invention Reinvented: McKinsey perspectives on pharmaceutical R&D, McKinsey & Company (2010), 1.
7. Margaret A. Hamburg, M.D., FDA Letter to Industry, http://www.fda.gov/Drugs/DrugSafety/DrugShortages/ucm277675.htm, (October 31, 2011).
About the author:
Ryan Sasaki is the Director of Global Strategy at ACD/Labs, Inc. It is his responsibility to liase with influential industry personnel and authorities in the chemistry and laboratory informatics industries. He has presented at several analytical chemistry and informatics events worldwide and has authored a series of publications on analytical chemistry and laboratory informatics. Most notably, he is the co-author of the white paper introducing the concept of Unified Laboratory Intelligence (ULI). Prior to his career at ACD/Labs, he served as a Process Chemist for a major pharmaceutical company in Canada.
Closing thought: How can we breathe life into analytical data?