Artificial Intelligence for better antibody drugs: ready for prime time?
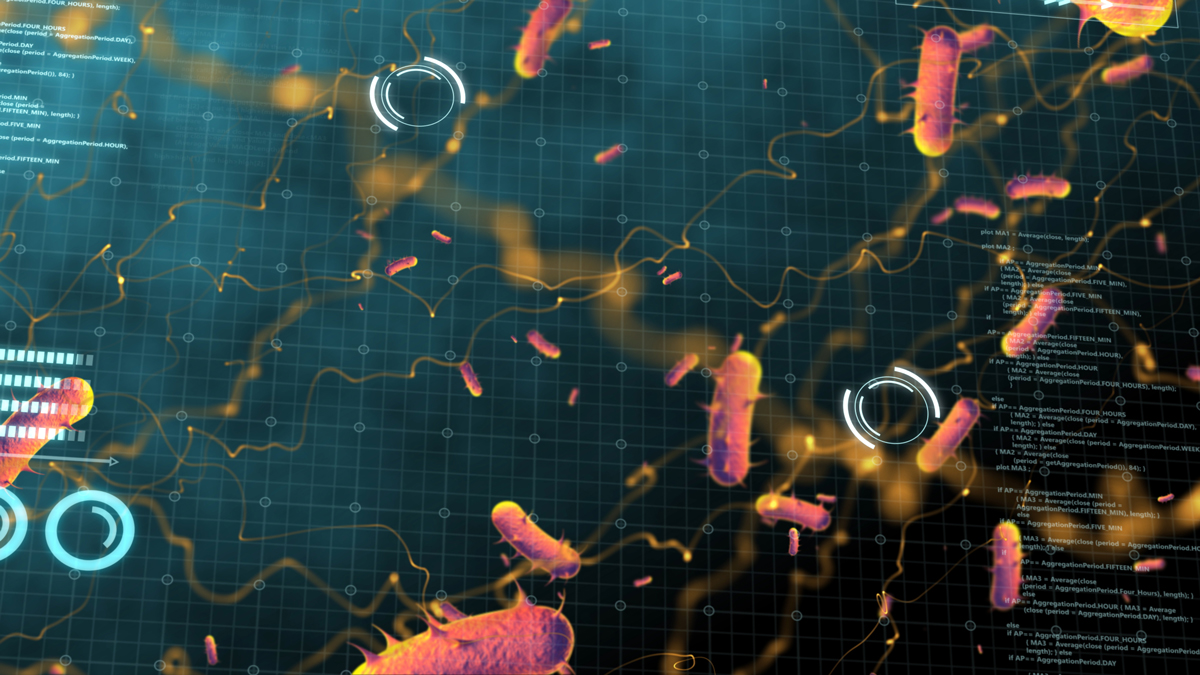
The potential of monoclonal antibodies (mAbs) as therapies is indisputable. The current mAbs market is expected to be around ~USD 150 billion. In 2021, five out of the top 10 selling drugs were mAbs and this trend is forecasted to remain the same over the next five years. Naturally, the pharma industry has seen an explosion of mAbs in the pipeline, which has led to the evolution of new methods for improving and refining the antibody pipeline.
Artificial Intelligence (AI) and deep learning have already transformed several aspects of small molecule drug design. Recent advances in the area are now also impacting more complex tasks, such as design and optimisation of antibodies. As a result, 2022 saw a breakthrough when the first ever computationally designed antibody created by Israel-based Biolojic design entered clinical trial, further fuelling the interest of biopharma companies.
AI for accelerated antibody discovery
Traditionally, mAbs are initially discovered (hit identification, or HI) through experimental techniques, like hybridoma screening or high throughput platforms like yeast or phage display. The process is followed by computationally guided mutagenesis or evolution of the antibody sequence (hit to lead, or H2L, optimisation) to improve the binding and other properties.
Although the space is in a nascent stage, several AI companies have made meaningful progress over the last few years. Adequate case studies are now present to demonstrate the benefits of the AI-guided evolution of hit for improvements to binding, solubility, yield, and immunogenicity, etc. Consequently, the lead optimisation or molecular evolution offerings in the space are relatively more common among the start-ups. However, a handful of companies are also tackling the task for de novo design of the candidates just from the target/antigen, skipping the need for time consuming and costly experimental methods, which could be a game changer.
Here are a few examples on how companies are using AI in diverse ways to accelerate antibody discovery:
1) Generate Biomedicine: Founded in 2018, it is a flagship pioneering company based in Boston and has raised $420M so far. Generate Bio uses a combination of sequence and structure-based approach towards de novo design of a variety of proteins, including antibodies with desired properties. In addition to its proprietary generative AI, the company has its own automation platform to rapidly synthesise and test antibodies in iterations.
2) BigHat Biosciences: Founded in 2019, the California based company has raised about $100M so far. They have developed an integrated platform that combines high throughput synthesis using cell-free system and testing of antibodies suggested by their antibody optimisation AI platform.
BigHat uses a sequence-based approach that requires hit data or initial sequence of antibodies discovered through other methods. The initial antibody sequence is then passed through machine learning (ML) models to optimise properties like binding to target, solubility, immunogenicity, and yield, etc. Data generated in each test cycle is used to update AI/ML models for the next cycle of iteration.
BigHat acquired, Frugi Biotechnology, a company developing cost-effective and high-quality cell-free protein synthesis (CFPS) technology, in 2022. They have five programs in internal pipeline and partnered programs.
3) Biolojic Design: Founded in 2009, the Israeli company uses a structure-based approach, where their model is trained on millions of Antibody-Antigen pairs in order to identify a template antibody against the desired target from existing human antibodies. It then uses a different ML model to predict mutations and guide evolution of the identified template to improve affinity and other biophysical properties.
While the above three companies are amongst the most evolved, the space is observing rapid growth. Here is a long list of biotechs in the AI antibody space:
Company name | HQ | Founded | Disclosed Funding |
---|---|---|---|
AbCellera | Vancouver, Canada | 2012 | Public co. |
AbSci | Washington, US | 2011 | Public co. |
Antiverse | Cardiff, Wales | 2017 | $2.1M |
BigHat Biosciences | California,US | 2019 | $99.3M |
ImmunitoAI | Bangalore, India | 2020 | $1M |
RubrYc Therapeutics | California, US | 2017 | $17.5M |
MabSilico | Tours, France | 2017 | - |
NeoX | Beijing, China | 2018 | $40M |
NeoX | Beijing, China | 2018 | $40M |
Natural Antibody | Szczecin, Poland | 2020 | $796.5K |
Biolojic | Rehovot, Israel | 2009 | - |
Totient | Cambridge, US | 2017 | $10M |
AI Biologics | Dublin, Ireland | 2020 | - |
Deep Biologics | Toronto, Canada | 2020 | $300K |
Aganitha AI | Michigan, US | 2017 | - |
Generate Biomedicine | Somerville, US | 2018 | $420M |
Deep CDR | Basel, Switzerland | 2019 | $80K |
Nabla Bio | Allston, US | 2020 | $11.3M |
Biomatter design | Vilnius, Lithuuania | 2018 | $608K |
AI Novo biotech | Cambridge, US | 2021 | - |
EVQLV | New York, US | 2019 | $40K |
AI in the antibody space is gathering momentum
Observing the potential of these platforms, biopharma companies have started exploring collaborations with a select few companies as well as building internal capabilities. Amgen partnered with Generate Bio for a discovery collaboration for five targets with a deal value of up to $1.9 billion, plus royalties. Chugai published promising results from their internal AI platform MALEXA-LI for antibody discovery. In August 2021, Genentech acquired Prescient Design. Four leading pharma companies - AstraZeneca, Merck, Pfizer, and Teva – along with Amazon Web Services, Inc. (AWS) and the Israel Biotech Fund (IBF) have also launched AION labs, an incubator to accelerate AI-driven antibody discovery.
Biopharma companies are not the only ones watching this space keenly. The space has already started witnessing M&A activities by CROs and large AI companies, with multiple acquisitions of early-stage start-ups. In 2019, Evotec acquired Just Biotherapeutics, an AI/ML antibody discovery start-up (founded in 2014) for $90M. AbSci, an experimental antibody discovery platform company, acquired deep learning company Denovium Inc. (founded in 2018) and Totient bio (founded in 2017), with large datasets to train the AI/ML models last year.
AI-biologics vs. AI-small molecule discovery spaces
Compared to the small molecule AI drug discovery ecosystem, this space is evolving differently. ‘Closed-loop’ companies that bring the best of both worlds, combining high throughput experimentation with AI technologies, are more prevalent as compared to only in-silico platform companies. Consequently, by learning from the evolution of the small molecule AI space, the majority of these companies are also focusing on internal pipeline, as they realise the significant upside that those assets can offer, as compared to a services-only model.
Moreover, the number of players is likely to be significantly less than in the small molecule AI space, considering the complexity of building AI models for intricate molecules like antibodies. Limited availability of datasets makes these ‘closed-loop’ companies even more attractive as they continuously generate their own data to improve the predictions.
Recent milestones include several large deals between AI companies and pharma, and exit opportunities through M&A has intrigued investors to further explore potential investment opportunities in the space. However, the AI-biologics discovery space is three or four years away from attaining the level of maturity of the AI-small molecule discovery space. Nevertheless, these are exciting times for biopharma, where such platforms are affecting the status quo and transforming the way we discover drugs.
About the Author
Amandeep Singh is a life sciences strategy consultant at MP Advisors, a biopharma-only strategy and financial advisory firm. He is skilled in growth strategy and driving business growth through organic and inorganic opportunities. He works with several start-ups developing AI platforms to catalyse their globalisation journey, and with several pharma groups to design and execute successful digitalisation and AI implementation strategies in drug discovery.