How AI-powered adaptive design helped Eisai launch Leqembi
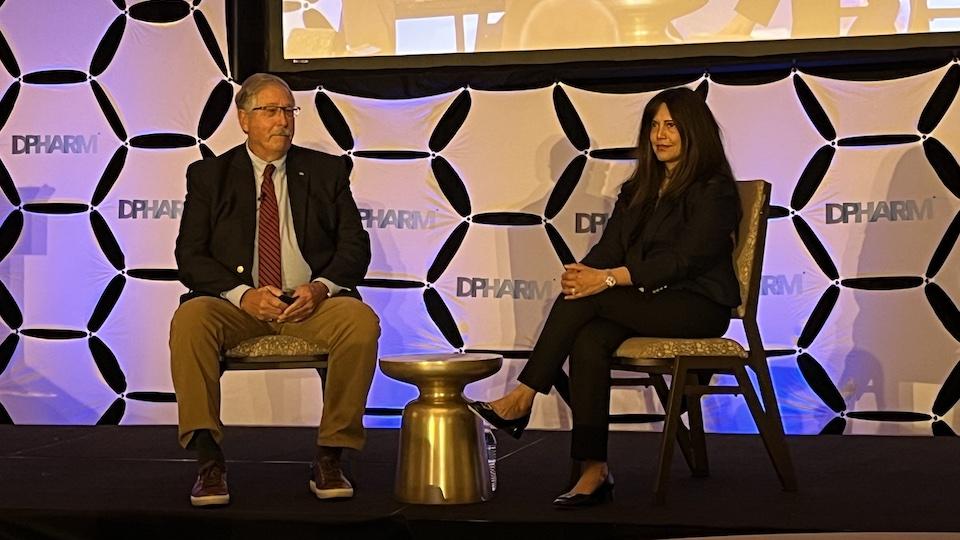
In July, when the FDA approved Eisai and Biogen’s Alzheimer’s drug Leqembi, we noted the historic nature of the news: it’s the first approved Alzheimer's treatment clinically shown to slow disease progression and cognitive and functional decline. But Leqembi is also notable for innovative methods used in an earlier phase of its development.
At DPHARM 2023 in Boston this week, Dr Lynn Kramer, Eisai’s chief clinical officer for Alzheimer’s disease and brain health (ADBH) and Shobha Dhadda, Eisai’s SVP and global head of biostatistics and project operations for ADBH, shared the story of Leqembi’s phase 2 trial, which employed an adaptive, Bayesian design.
Long theorised but only recently implemented with any regularity, adaptive clinical trial designs are those that can be modified as they progress when new information emerges, either from the trial itself or externally.
“When we were starting to design the study around 2012, there was a shift toward early Alzheimer's disease stage. And there were several phase 3 drugs that had failed in the last two decades,” Dhadda said. “So we were very clear that we had to have a strong clinical proof of concept before we could move into a large phase 3 programme. And there were still many unknowns around lecanemab.”
The phase 2 study needed to broadly prove out the drug, as well as answer questions about dosing and duration that would set up the phase 3 trial for success.
“If we had designed a traditional study with five or six dose arms, it would have cost us a lot of money, would have taken a long time, and would have needed at least four times the sample size. So we decided we would try this Bayesian adaptive design and see how that works,” she said.
They used a response adaptive randomisation, which means that rather than determining how many patients would be in each intervention group at the start of the trial, they instead built an algorithm that would change those allocations based on effectiveness data as it came in. In effect, if a dosage was an obvious failure early on, they could stop assigning subjects to it.
Note that one of the reasons these designs have only recently begun to see use is the complexity of that algorithm, which necessitated a lot of upfront work.
“It took almost six months to run all these simulations and finalise. But at the end of it, what you get is a computer algorithm that's ready, that can look at different dose-response scenarios. Is it going to be a linear slope? Is it going to be S curve? Is it going to be U curve?” Dhadda said. “Once it's built, you have to just implement that algorithm into the study that you don't touch during the study.”
The use of computers allows a study design to be both adaptive and double-blind, Kramer pointed out.
“This not an unblinded study,” he said. “Computers do these allocations. People never see the result during the entire study until the very, very end. That's why it takes six months to do all these simulations and have the system set up so that it can do this effectively regardless of what's happening in the study.”
At 12 months, the study actually failed to meet its primary endpoint. However, it did succeed in identifying the most effective dose regimen at 18 months, which set the company up for phase 3 success.
The team used a number of different biomarkers to feed into the algorithm, including some that they otherwise might not have included, such as ADCOMS, an Eisai-developed measurement that, according to the company, “combines items from the ADAS-cog scale for assessing cognitive functions, MMSE and the CDR scale for evaluating the severity of dementia to enable highly sensitive detection of changes in clinical functions of early AD symptoms and changes in memory.”
“It's not a validated endpoint in Alzheimer's disease trials,” Kramer said. “It was developed to get us to the allocation rates faster because it's a little more sensitive early in the disease. And then, the results in the phase 3 study were almost identical to the phase 2 study. So it predicted exactly what we needed to do.”