AI and cancer care: 3 ways artificial intelligence may transform cancer outcomes
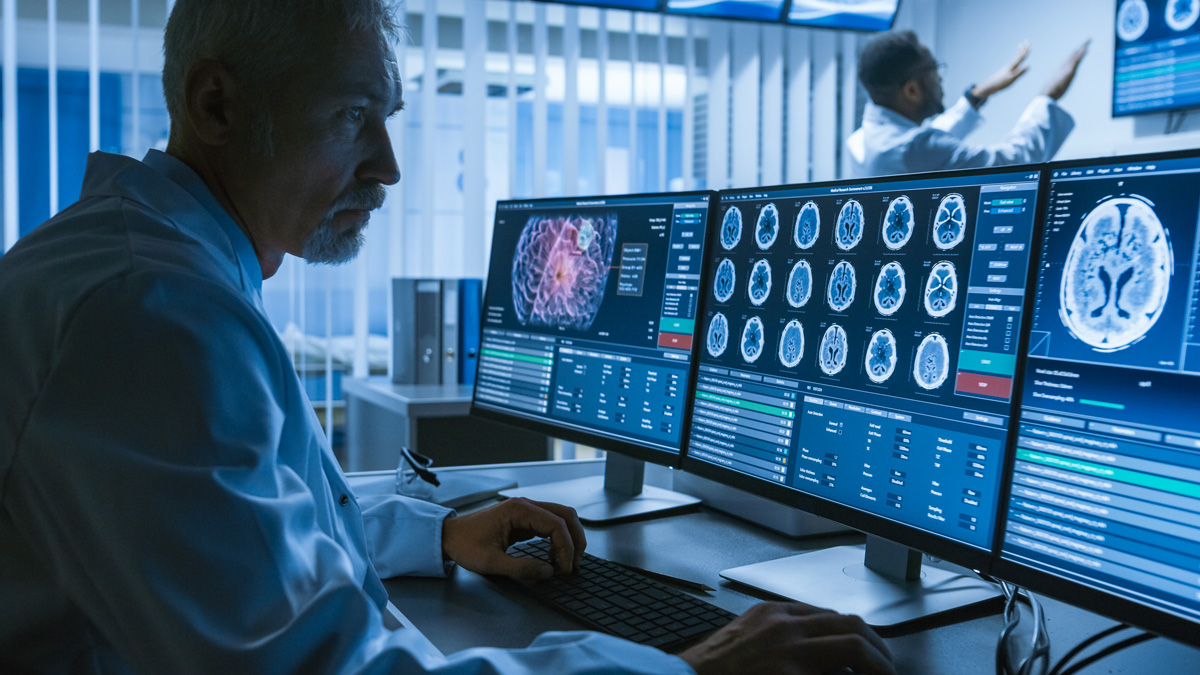
Artificial intelligence (AI) and machine learning (ML) are transforming the way cancers are detected and staged, offering new hope to the millions of people affected by the diseases every year.
We take a look at three ways AI and ML are moving the field forward and ask whether the technology really is the holy grail of cancer care.
1.Liquid biopsies
Tissue biopsies have been a staple of cancer diagnosis for decades, but the invasive procedure comes with its fair share of risks. Using ML-based algorithms to conduct liquid biopsies is a possible solution.
A study published in Nature last week (25 March) suggested that, one day, screening for lung cancer could be as easy as taking a blood test. It found an AI programme designed to detect circulating tumour DNA (ctDNA) in blood samples could prove a useful tool in the early detection of the disease.
The ML method, called lung cancer likelihood in plasma (Lung-CLiP) can “robustly discriminate early-stage lung cancer patients from risk-matched controls”, said the paper. It may also be able to provide a guide to prognosis, by analysing the level of ctDNA in the blood, it added.
Between 60-70% of lung cancers are currently diagnosed at stage four, meaning survival rates are poor. Lung-CLiP, which still needs to be validated in clinical trials, could help identify early stage cases while they are still curable.
The system, which had a 2% false positive rate in the trial, cannot work alone, however.
The authors said: “One potential application of Lung-CLiP could be to serve as an initial screen for some of the approximately 95% of high-risk patients in the United States who, despite being candidates for low-dose computed tomography (LDCT) are not being screened… Patients with positive Lung-CLiP tests would then be referred for LDCT.
“Although Lung-CLiP is less sensitive than LDCT, this hybrid approach could potentially increase the total number of patients screened, and, therefore, the number of lives saved annually in the United States, from the current annual value of around 600 to closer to the projected maximum of around 12,000.”
2.Imaging
Imaging is a central plank of cancer detection, but growing demand combined with a shortage of radiologists is placing pressure on services worldwide.
AI and ML-driven imaging, then, has become something of a hot topic, and headlines proclaiming the technology can detect cancer “as accurately as doctors” have become common.
A study published in the Journal of the National Cancer Institute last year, for example, compared the performance of 101 radiologists to that of a commercially available AI system.
They used 2,652 exams, 653 of which were malignant, from retrospective sources that had been produced by four different mammogram machines. They found that the AI’s cancer detection accuracy rate was comparable to the average rate of the radiologists
"Before we could decide what is the best way for AI systems to be introduced in the realm of breast cancer screening with mammography, we wanted to know how good these systems can really be," said Ioannis Sechopoulos, one of the paper's authors.
"It was exciting to see that these systems have reached the level of matching the performance of not just radiologists, but of radiologists who spend at least a substantial portion of their time reading screening mammograms."
It is not the only study to have shown that technology can be just as, if not more, accurate than radiologists.
Imperial College London and Google Health, for example, published work earlier this year that showed its algorithm, trained using a database of more than 29,000 images, could detect breast cancer as well as experts.
“We ran a simulation in which the AI system participated in the double-reading process that is used in the UK, and found that the AI system maintained non-inferior performance and reduced the workload of the second reader by 88 per cent,” said the authors.
Such systems are not designed to replace healthcare professionals, but rather help them to reduce their workload by focusing on the people who really need their help.
3.Pathology
Pathology is another area of healthcare feeling the effects of an uptick in demand and a lag in training and recruitment. Technology could, then, play a role.
Dartmouth-Hitchcock Medical Center has published work which focused on ML’s ability to determine tumour patterns and subtypes in lobectomy slides.
Their deep neural network, designed to help pathologists grade tumour patterns and subtypes of lung adenocarcinoma, was found to perform on par with three practising pathologists.
“Our study demonstrates that machine learning can achieve high performance on a challenging image classification task and has the potential to be an asset to lung cancer management,” said the authors, who also want to test their model in breast, esophageal and colorectal cancer.
“If validated through clinical trials, our neural network model can potentially be implemented in clinical practice to assist pathologists.
“Our machine learning method is also fast and can process a slide in less than one minute. It could help triage patients before examination by physicians and potentially greatly assist pathologists in the visual examination of slides.”
Common threads
AI and ML advocates are making huge strides in bringing the diagnostic and prognostic power of technology into prime time, but two common threads run through all their work.
The first is that this is early days, and all systems will require extensive testing and validation before they are seen in routine practice.
The second is that these models are not enough to tackle cancer alone. The robots aren’t taking over – they are but another piece of equipment in the healthcare professionals’ cancer care tool kit.