Company Profile: TetraScience
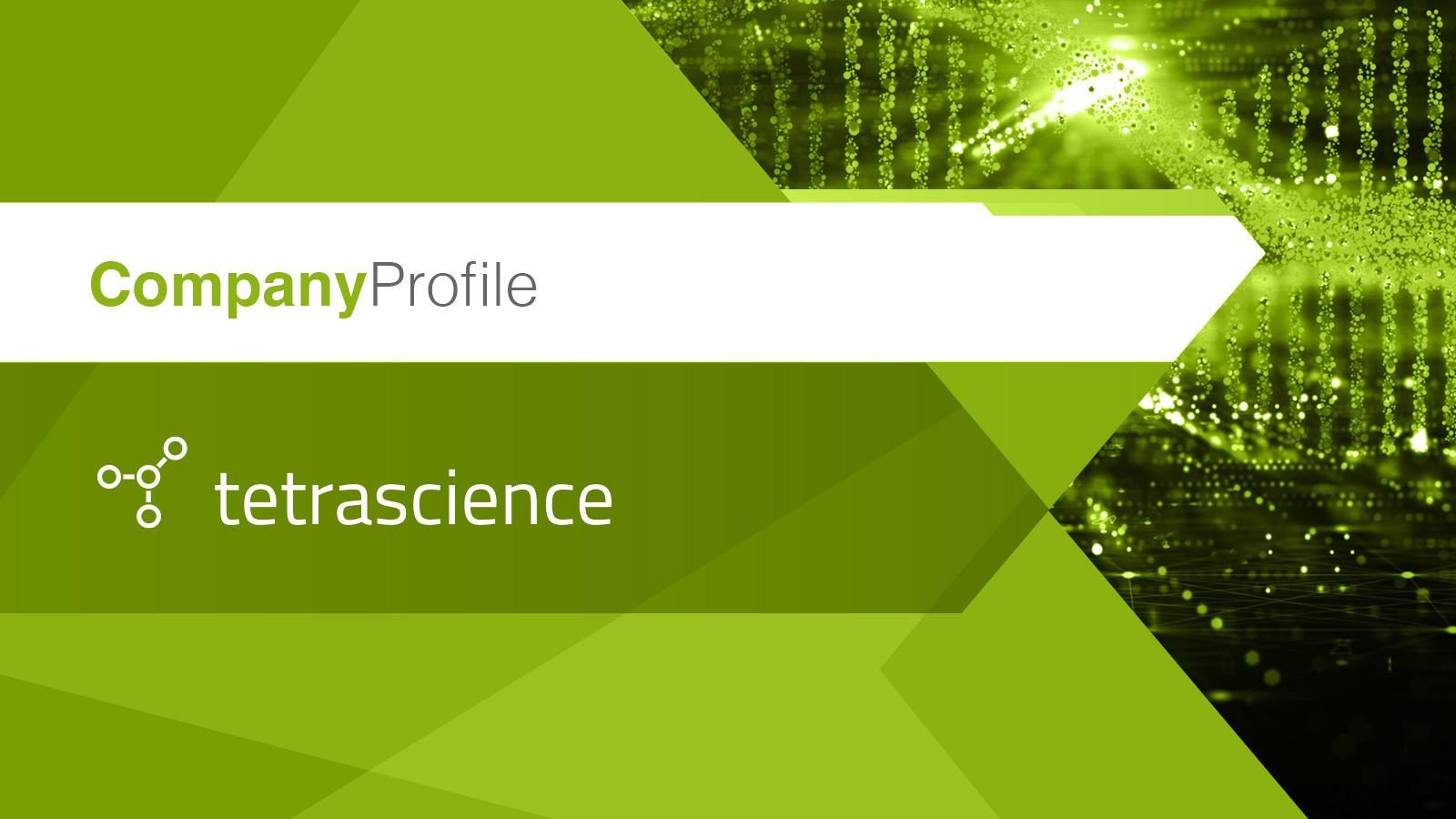
Patrick Grady
Boston, MA, USA
200+
2014
R&D, QA/QC & Manufacturing
Tetra Scientific Data Cloud™
Today, the world’s leading life-science companies can’t access, compare, or fully utilise their most precious commodity - their scientific data. That needs to change.
Scientific data is often locked in silos. Organisations can’t easily locate or access their experimental results. They can’t, with any certainty, know how the data was generated. And they must manually and painstakingly transform data to use it within informatics applications, analytics applications, or AI/ML.
As a result, these companies struggle to move up the scientific data pyramid. This greatly reduces their ability to reuse scientific data and drive strategic decision making.
The scientific data pyramid
To address the issues above, TetraScience developed the industry’s only scientific data cloud. It combines leading technologies, an extensive ecosystem of life sciences partners, and deep scientific and technology expertise to help organisations unlock the full value of their scientific data across R&D, manufacturing, and QA/QC.
The Tetra Scientific Data Cloud is an open, vendor-agnostic solution that
- Collects data from instruments and applications
- Centralises data in the cloud for easy access
- Enriches data with metadata to provide context and facilitate search
- Engineers raw data to be compliant, liquid, actionable, and harmonised
TetraScience, The Scientific Data Cloud Company, helps biopharma organisations accelerate and improve scientific outcomes - while reducing costs, improving productivity, and minimising compliance risks and efforts.
The Tetra Scientific Data Cloud
The Tetra Scientific Data Cloud provides the foundation for data science and artificial intelligence. It converts primary raw data into enriched and harmonized data that is fully optimised for AI/ML. Companies can readily access and build data sets from the cloud to feed AI/ML models, enabling deep insights that fuel breakthroughs.
R&D, manufacturing, and QA/QC environments rely on many life sciences vendors, complicating data workflows. What if vendors collaborated to streamline the scientific data journey for their customers?
The Tetra Partner Network aims to make this “what if” a reality. It’s the industry’s largest network of life sciences vendors dedicated to connecting data ecosystems and maximising the value of scientific data. It brings together instrument, informatics, and data analytics experts to help customers achieve faster and better science, more efficient operations, and safer, more compliant processes.
To drive innovation, scientific data must be compliant, FAIR (findable, accessible, interoperable, and reusable), universally available, and prepared for analytics. However, getting there is an incremental process.
Step 1: Ingest raw or primary scientific data
The first problem to solve is fragmented, siloed, disconnected data across instruments and applications. These data sources are in different formats ranging from text files, data collected via APIs, data obtained from SaaS software, or proprietary data generated by instruments. Organisations need to integrate instruments and applications to collect and store data from these sources in the cloud. This first step breaks down silos, reduces the chance of losing data, and provides greater access. However, stopping here can be a costly mistake.
Step 2: Enrich with context and ensure traceable provenance
In its raw form, extracted scientific data may provide very little context. Enriching it with metadata (e.g., sample ID, study name, and instrument model) preserves the full meaning and provenance. This process also makes data easier to find and helps maintain an audit trail that tracks changes to data and configurations, aiding regulatory compliance.
Step 3: Harmonise across data sources and formats
Contextualised (but raw) data is still stored in divergent, vendor-proprietary formats, complicating use, reuse, and interoperability. New instruments and applications add to the number of data formats in play, increasing data complexity when scaling. Engineering data to be FAIR is a desired outcome for most biopharmas today.
Thus, the third step of the scientific data journey is harmonising data from different vendor-proprietary formats into an open, vendor-agnostic format. Having a common format simplifies ingesting data from sources and driving data to targets, easing the process of incorporating new technologies. Additionally, and importantly, a large data set in a common format enables analytics, visualisations, and the full power of AI/ML to deliver more complete insights to the organisation.
Biopharma companies need a future-proof data framework that will meet their needs at all stages of drug development. That’s why we developed the Tetra Data Platform. It achieves the three steps above and converts raw scientific data into Tetra Data, which is:
- Compliant with GxP and other regulatory requirements
- Harmonised from disparate formats into an open, vendor-agnostic format that is searchable and accessible
- Liquid to be reused multiple times and seamlessly flow across instruments and software
- Actionable by enabling analytics, visualisation, and AI/ML
With Tetra Data, life sciences organisations have access to high-quality data where they need it, when they need it. Scientists and data scientists spend less time collecting, searching for, and preparing scientific data and invest more time in science.
From discovery to manufacturing, the Tetra Scientific Data Cloud has accelerated and improved scientific outcomes in biopharma, such as:
- 300 hours saved per day by eliminating manual data intervention
- 44% increase in experiment throughput and productivity
- $2.5M cost reduction through end-to-end data automation
To learn more about how you can accelerate and improve scientific outcomes, visit tetrascience.com.