Understanding the impact of AI on the advancement of gene therapy development
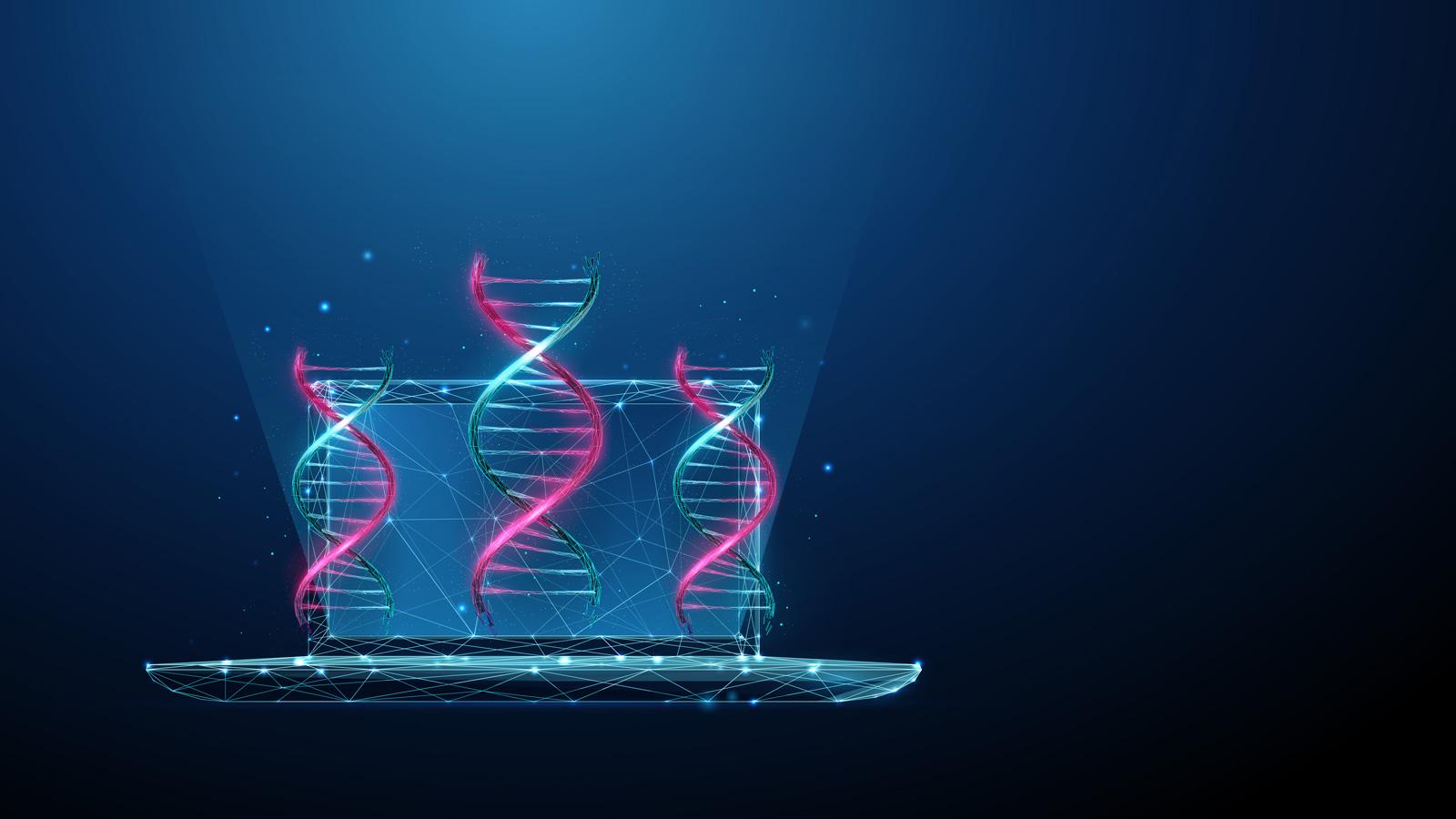
The biopharmaceutical landscape stands at the cusp of a transformative era, with artificial intelligence (AI) leading the charge. For gene therapy developers, every key milestone significantly influences long-term success and the timeline for commercialisation.
Yet, amidst this pivotal moment, a crucial question looms: how do you “separate the wheat from the chaff” in the field of AI? The challenge lies in pinpointing specific offerings and tangible AI innovations that yield substantial improvements in cumbersome and costly steps in the development process. The key to successfully integrating AI into gene therapy development is recognising at which steps AI can be a strategic advantage, and doing it faster than your competitors.
Opportunities to implement AI across the entire R&D pipeline
The gene therapy development process requires engagement with a large network of partners, including large pharma, small and mid-size biotechs, healthcare providers, patients, payors, regulatory bodies, and contract manufacturers.
With the rise of AI, in silico partners have been added to this list of essential drug development partners. These technology vendors offer AI-driven advancements that extend across gene therapy development stages, from pre-clinical testing to post-commercialisation. Another area that has seen major growth is the research and development space, where AI has been leveraged for many things, from target identification to preparing regulatory submissions for first-in-human trials.
Here’s a look at specific use cases where using AI-based tools can decrease costs, alleviate the risk of failure, and expedite the overall gene therapy development timeline.
Accelerating pre-clinical gene target discovery
The overwhelming volume of large-scale, disease-related genomic, other ‘omic, and publicly available real-world datasets surpasses human capacity for meaningful analysis. To address this challenge, AI, including large language models (LLM), has proven invaluable in sifting through and making sense of the vast corpus of high-throughput, diverse, and intricate genomic and molecular data. This capability aids in identifying potential therapeutic targets and extracting crucial features or relationships within biological networks. Through LLM, research groups can pinpoint promising gene targets, validate their therapeutic significance, and thus expedite the discovery and assessment of novel candidates for gene therapy interventions.
Minimising iterative time in vector and capsid design for transfection efficiency and tissue targeting
AI algorithms have enhanced viral vector design, de-risking, and optimisation, leading to improved safety and manufacturability. Computational modelling and simulation make it possible to assess the efficiency of producing encapsidated full vectors, ensuring the highest likelihood of manufacturing success while maintaining cost efficiency. Coupled with some extensive AI-driven AAV capsid design studies, these capabilities open up new possibilities for targeting specific tissues and avoiding pre-existing immunity to AAV vectors - a major limitation of current gene therapies.
Optimising gene therapy product development processes for manufacturing
As development progresses, attention shifts from efficacy to scaling-up production in a cost-efficient way. The complexity and time-consuming nature of optimising the production and purification process pose challenges, with downstream enrichment adding to the high cost of goods. Given the complexity of gene therapies, beginning this troubleshooting early in development can be beneficial, yet, optimising research processes for large-scale and GMP applications presents additional difficulties.
AI algorithms can help identify capsid variants that are highly manufacturable or perform simulations of the manufacturing process early in development to help identify issues before they are encountered later, during pre-clinical or clinical testing.
Expediting regulatory filings for initial human trials
For gene therapy companies, considering these recommendations early in the development of gene therapies will be necessary for a successful path to market, particularly as the FDA formulates its position on protecting the safety of clinical trial participants and manufacturing protocols.
Clinical holds for gene therapies are often due to incomplete Investigational New Drug (IND) applications that need a manufacturing section that communicates the precise composition and attributes of these therapies. Integration of vertical AI to streamline these product characterisation processes will aid in developing a well-defined and organised manufacturing protocol and analytical standard presentations will help to get your gene therapy through the IND approval process. Moreover, GenAI solutions that streamline the creation and management of regulatory documents, will ensure adherence to industry standards, but also alleviate the manual labour burden.
Focused enrolment for clinical trials benefitting eligible patients
Recruiting patients for gene therapy trials, primarily targeting rare diseases, often proves challenging due to the life-threatening nature of these disorders, and ethical concerns about placebo control arms. AI, especially LLM, can be pivotal in identifying suitable patient cohorts, as LLM analyses unstructured medical data to pinpoint individuals meeting trial criteria, expediting recruitment by alerting healthcare professionals and patients.
What’s next for AI in gene therapy research and development?
With the above use cases, it’s clear that AI is positioned strategically to accelerate gene therapy development and we’re starting to learn the extent of improvement that AI will offer, so we can create realistic goals. But what are the next steps, and how can developers practically implement AI in their current workflows and ongoing programmes? Transitions are never easy; finding an in silico partner with a standardised and scalable approach is essential. As AI continues to advance gene therapy, more pragmatic points of initiation in R&D will emerge, further facilitating lower costs and more rapid development.