AI and the battle against AMR
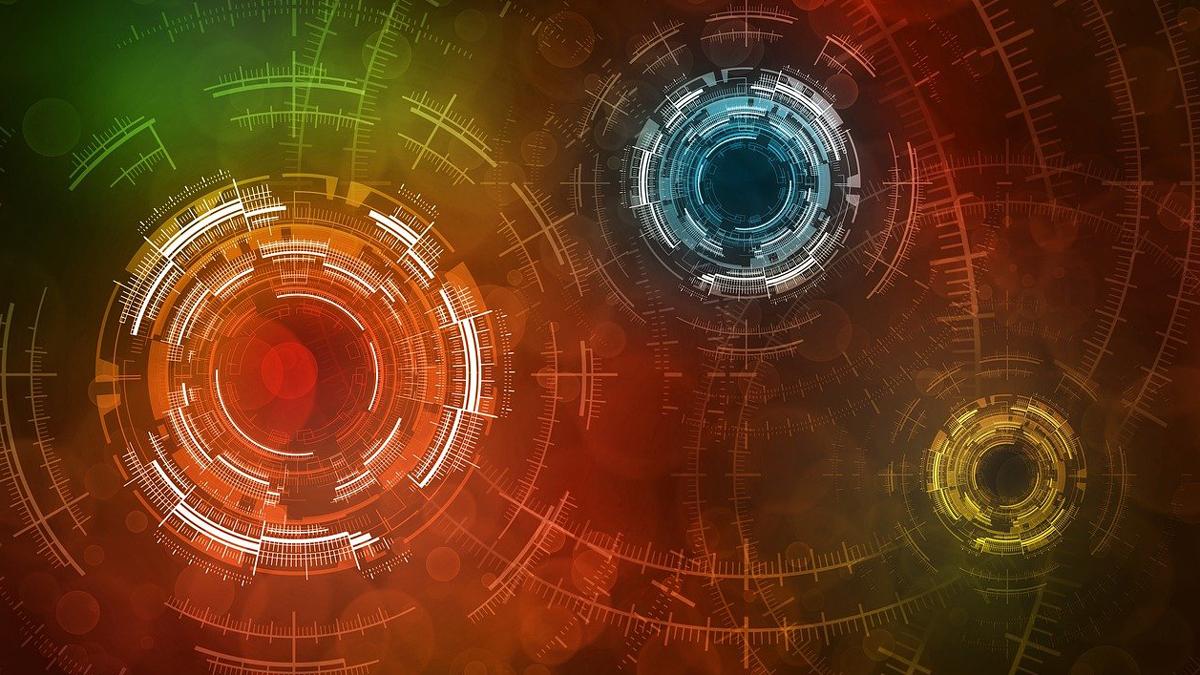
One of the largest threats facing public health is growing resistance to the world’s existing antibiotics. Ben Hargreaves looks at the current challenges facing expanding the pipeline of antibiotics and how AI could prove an essential tool in reinvigorating the fight against antimicrobial resistance.
There is currently a weak pipeline for new antibiotics. These are the words of the World Health Organization (WHO) after it reviewed two reports finding that few of the products in development offered substantial benefit over existing antibiotics. This is a problem, and a significant one, as antimicrobial resistance (AMR) is on the rise. The WHO notes that AMR is one of the top global public health threats, being responsible for 1.27 million deaths in 2019. Rising AMR and a weak global pipeline for new antibiotics place global health in a precarious position – over-reliant on an existing roster of treatments that are increasingly being rendered ineffective.
The challenge is that developing new antibiotics is just as resource-intensive as other drug areas, but without the commercial payoff to provide an incentive. This has led big pharma to gradually exit from the area, while countries try to construct a payment model that might reinvigorate interest in developing antibiotics. This has led smaller companies to explore ways to create a new generation of treatments, such as through new treatment tools, such as CRISPR technology.
However, beyond discovering or creating new antibiotics, there may be a solution that already exists: using AI to identify existing compounds with the potential to fight bacterial infections or to target drug-resistant bacteria with the appropriate antibiotics. It is still early on in the process of using AI in this capacity, but there has already been some success in this pursuit.
AI and building a pipeline
In December 2023, MIT researchers revealed that they had used AI to discover a class of compounds that can kill methicillin-resistant Staphylococcus aureus, otherwise known as MRSA. The potential antibiotics were found to be able to kill MRSA in a lab dish and in mouse models with MRSA infection. In addition, the potential treatments were found to have very low toxicity against human cells, which could make the compounds suitable as drug candidates.
The choice of MRSA as a target was significant because this type of gram-positive bacteria is responsible for difficult-to-treat infections that can be resistant to a number of commonly used antibiotics. It is estimated that MRSA is responsible for 9,000 deaths and 70,000 severe infections in the US per year, and there were an estimated 121,000 total deaths globally in 2019. MRSA is a leading example of the threat posed to public health by infections that are resistant to current treatment options.
This made the bacterium an appropriate target for the researcher’s AI tools. According to MIT, one of the innovations of the study was being able to identify what kinds of information the deep-learning model was using to make its antibiotic potency predictions. Previous iterations of the model had learnt to recognise chemical structures with antimicrobial activity, which it could then measure against millions of other compounds. At this point, the model could then make predictions on compounds that could potentially be used as antibiotics. However, the team involved noted that a limitation of this approach was that there was no way of knowing what features of the compounds the model was basing its prediction on.
In the latest research, the study opened up this ‘black box’ of the machine learning’s process to include a ‘search’, an adapted form of the Monte Carlo tree search algorithm. With this in place, the model could generate an estimate of each molecule’s antimicrobial activity, and also which substructures of it would likely account for this activity. The model was able to screen about 12 million commercially available compounds, before selecting 280 and testing them against MRSA grown in a lab dish. This pool was then narrowed down to two compounds that were tested in mouse models and found to be effective – reducing MRSA population by a factor of 10.
In terms of the next steps, the researchers shared their findings with Phare Bio – a non-profit start-up, linked with the researchers, that is aiming to develop a pipeline of AI-generated antibiotic candidates. The company will carry out an analysis of the chemical properties and potential clinical use of the compounds discovered.
Targeted treatment
On the other side of the battle against AMR is the need to treat patients who have contracted an antibiotic-resistant infection. As AMR sees the risk of this increasing, a quick and effective test to determine whether the infection is resistant to certain antibiotics will rise in importance. A team from the University of Oxford set out to develop such a test using AI and fluorescence microscopy.
The researchers created the test by using deep-learning models to analyse bacterial cell images to detect structural changes that may occur when treated with antibiotics. The result was a test that was effective across different antibiotics with at least 80% accuracy.
Achillefs Kapanidis, director of the Oxford Martin Programme on Antimicrobial Resistance Testing, said, “Antibiotics that stop the growth of bacterial cells also change how cells look under a microscope, and affect cellular structures, such as the bacterial chromosome. Our AI-based approach detects such changes reliably and rapidly. Equally, if a cell is resistant, the changes we selected are absent, and this forms the basis for detecting antibiotic resistance.”
Although there are existing tests to determine resistance, the method developed through this research was found to be 10 times faster. This could be important in a treatment situation where dosing a patient with an ineffective antibiotic in an urgent situation, such as an infection with sepsis, may lose valuable time. In addition, the need to dose patients with multiple antibiotics can also contribute to even greater antimicrobial resistance to antibiotics.
As noted by one of the study’s authors, time is beginning to run out for existing antibiotics – the focus should now be on extending their lifespan, and moving quickly to expand the pipeline of new antibiotics. Despite the gravity of the threat, the commercial interest is not there yet. However, AI could prove to be the tool that manages to circumvent this.