AI may not be able to keep up with real-world medicine use, experts warn
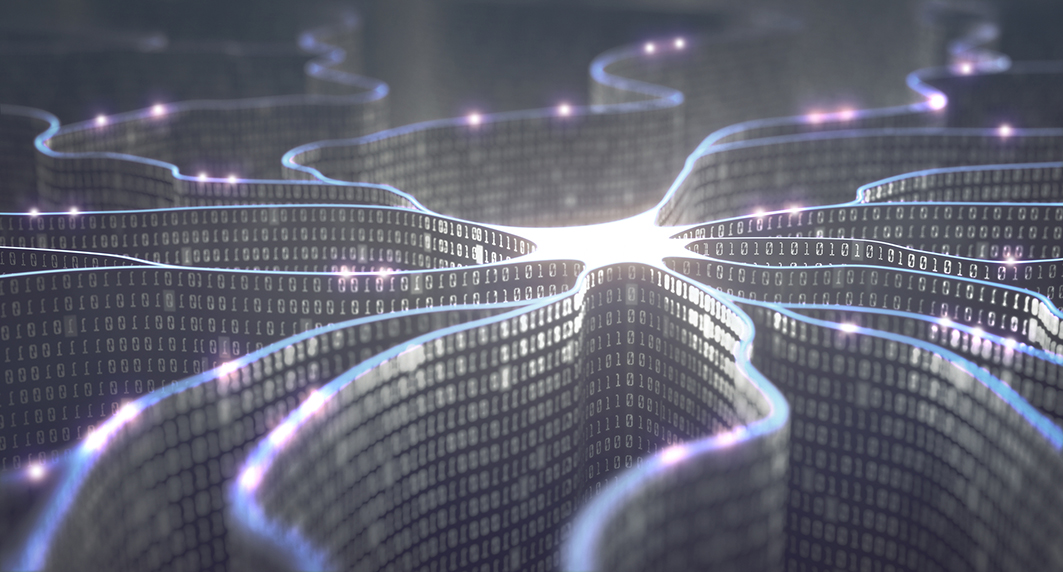
Experts in digital health have published guidance on use of artificial intelligence (AI) in healthcare, warning that the technology may not be able to keep up with how medicines are used in the real world.
Writing in The Lancet, experts led by Marzyeh Ghassemi, of the University of Toronto and Vector Institute, noted AI’s “potential to provide personalised care equal to or better than humans for several health-related tasks.”
But they noted that applying AI to healthcare has challenges including “label uncertainty” when algorithms are based upon information from drug labels.
While regulators will take one view of how a drug is used, this may differ in clinical practice when guidelines for medical treatments might be tweaked after approval.
Algorithms may not always be able to pick up such subtleties and authors proposed several workarounds, such as separating populations into different subtypes, and incorporating diagnostic baselines into algorithms.
Authors wrote: “In the long term, full capture of data from robust sources is needed to match self-reported patient data with expert-verified clinical outcomes.”
Aside from the issues surrounding labels, clinical data can be “messy, incomplete, and potentially biased” with several strategies in play to cover incomplete or missing data.
Many AI-based health technologies rely on data from electronic health records (EHRs) – but this information can be collected from different sources and without the consideration of developing algorithms.
Data can be collected from a wide range of sources, from high-frequency signals collected every thousandth of a second, to vital signs collected hourly.
They can also include images, lab tests, notes, and static demographic data, and formats can vary according to the organisation that collected the information.
Problems with predictions can arise when measurements change considerably – for instance data collected in an urban hospital might not predict those in a rural setting.
Other factors may unintentionally confound measurements, and therefore predictions, such as upgrading to newer equipment, authors said.