Poor data hindering machine learning in drug R&D
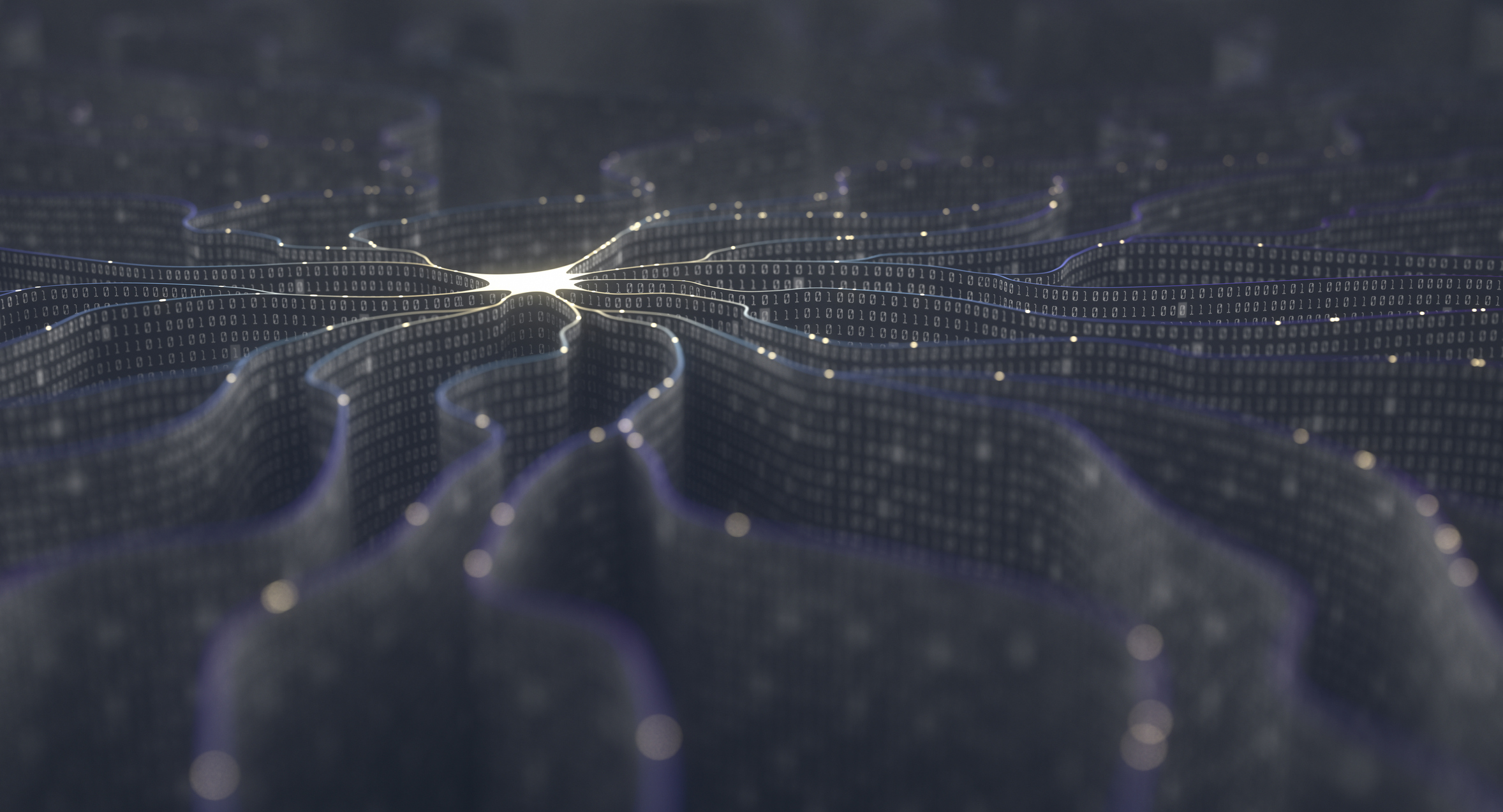
A lack of high-quality data is hindering use of machine learning in drug development, according to a new report from the US government.
The report said that machine learning has the potential to cut costs of research by helping predict what will work, and what will fail during the clinical trial process.
Machine learning is a specialised sub set of artificial intelligence, using data to train computers to make decisions and learn from experiences.
But the new report from the US Government Accountability Office and the National Academy of Medicine pointed out that much of the data used in drug development were not for machine learning purposes.
It’s the latest report to warn of the phenomenon known as “garbage in, garbage out”, where a machine learning system is unable to produce credible results because of the poor quality of the data it was trained with.
Biases in the data, such as under-representation of certain populations, may limit the technology’s effectiveness, according to the two-part report.
Aside from the shortage of data another obstacle is sharing what is available due to costs, legal issues and lack of incentives for sharing.
Acquiring, curating, and storing data is expensive and there are uncertainties over privacy that may limit economic incentives.
There is also a shortage of skilled workers in the field, making hiring and retention challenging for drug companies, according to the second part of the report from the GAO that focused on machine learning.
Drug companies also expressed confusion about regulations, which could limit investment in machine learning.
Other countries’ support of machine learning in drug development could create a competitive disadvantage for the US, the report added.
The report made a series of recommendations for policymakers to implement to help overcome these challenges.
There should be mechanisms and incentives for sharing of high quality data held by public or private organisations, including legislation to prevent improper sharing or use.
Standardisation of data could also allow researchers to combine different data sets, and could also help make algorithms more understandable and transparent to end users.