An overview of AI in pharma – Part 2
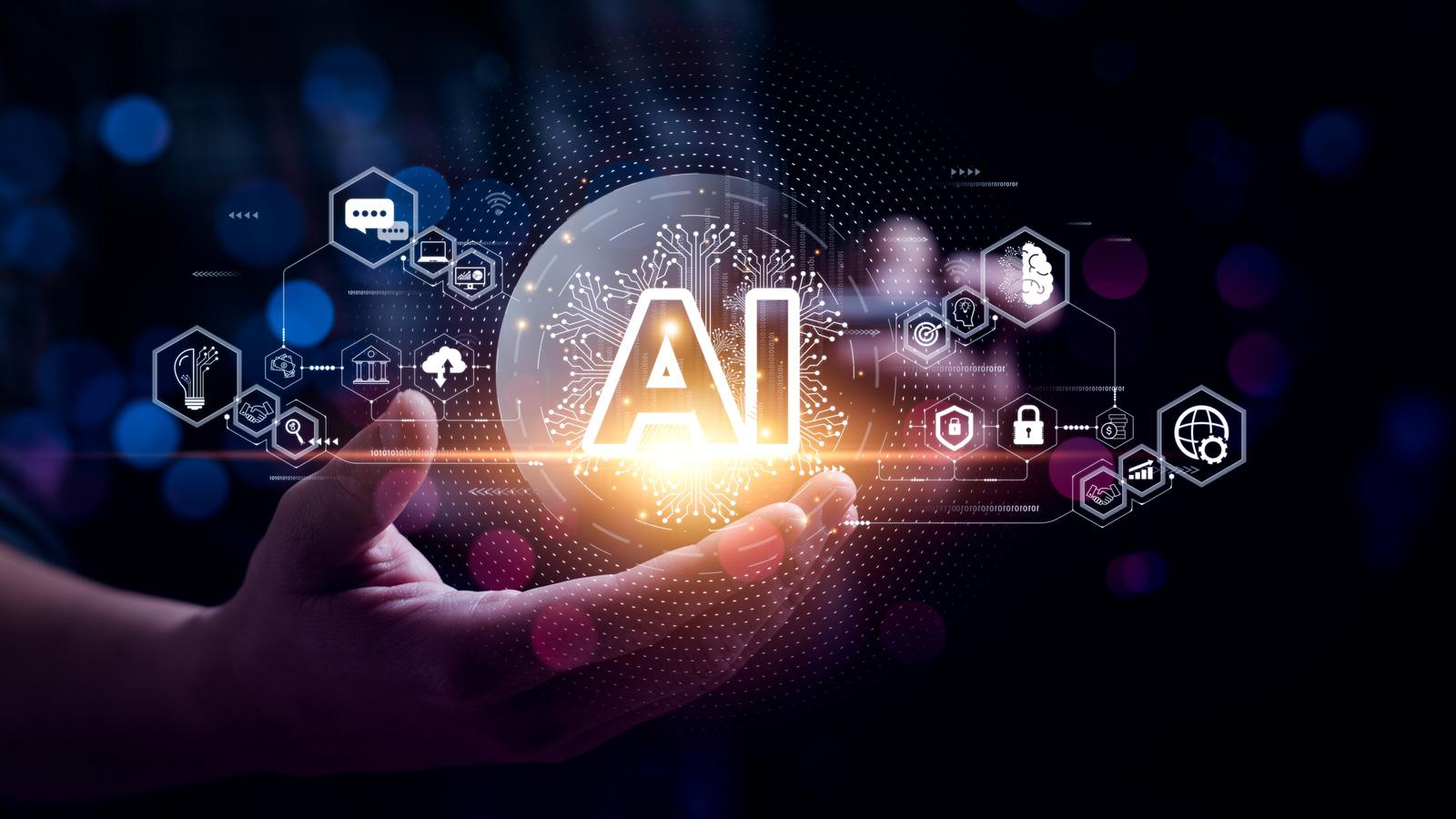
According to Takeda Pharmaceuticals, the average time taken to diagnose a rare disease without technology is 7.6 years, and that comes after countless tests and physician visits. This creates a high cost to the healthcare system, not to mention much suffering for the patient. And some cases are even worse. Rare diseases offer diverse challenges. With pharma offering rare disease medication, if you can find the patient, they will take your drug; however, finding the patient is the tricky part.
Delays in diagnosis due to the rarity of the conditions can often lead to exacerbated severity. In the past, this meant many patients were never diagnosed, or the condition was so late-stage by the time they were, it didn’t help them.
Now, using AI, we can identify all of these patients within the data sets within days, after the initial time spent data wrangling and creating the algorithms and tech stack. Then, each time a new patient enters the healthcare system, that patient is immediately identified using the algorithms.
AI to detect ultra rare disease patients: A case study
This case study below is a project we did many years ago for a client. This is only one way to approach it and we approach it differently now, due to ethics around face recognition.
Since this project, we have employed newer ways to find both rare disease and undiagnosed patients rapidly, using AI with big data sets (usually EHR and claims data, but also public government big data sets and other more lateral data sets as well). However, due to the children’s lives saved in this case study, it does remain one of my favourite ones, and an innovative one, so it may be of interest.
The client challenge
The client had a rare disease drug with no competition.
- The illness is so rare, however, that the main difficulty was finding patients.
- Very few physicians were likely to encounter these patients.
- Finding these patients early is critical as the illness progresses.
The solution
Certain facial traits were common to sufferers of the condition.
- Eularis created facial recognition software that identified patients with the condition.
- It then crawled internet properties (Facebook, etc.) to find patients from online photos.
- This allowed for faster identification and treatment before it was too late for these patients. The people who had uploaded the photos had a retargeting cookie dropped on them (the same sort they used to do with advertising).
- However, due to sensitivity, the identification was addressed in a more palatable way.
- It was discovered that the parents of these babies and toddlers had no idea anything was wrong with them until they started to potty train. Then, they would search about potty training to try and get help.
- Once patient photos were identified by the algorithm, targeted advertising of keywords on Google PPC was prepared.
- Therefore, only when a search was conducted on the symptoms of the child and potty training by the family would this be activated.
- This triggered an ad with a picture of a child with the condition (age-appropriate to the subject’s child so that it caught their attention, as it would look like their child) leading them to our client’s disease awareness website where they could self-diagnose.
The outcome
Thirteen new patients were identified very rapidly, as the parent saw the image and went to the website and then took that information to their doctor and got the medical genetic test required to diagnose them - and they got the drug, and their lives were saved, as they would have died before reaching their teens without treatment. This provided remarkable patient outcomes.
Compliance and AI
It goes without saying that compliance in the context of AI in the pharmaceutical sector is critical for maintaining patient safety, data integrity, and public trust. Below are some key aspects and challenges:
Real-world data utilisation: AI applications in the pharmaceutical industry often rely on real-world data collected from various sources, such as electronic health records, wearables, and social media. Ensuring compliance with data quality standards and appropriate consent mechanisms for using this data is crucial for maintaining ethical standards and regulatory compliance.
Clinical trial optimisation: AI can significantly enhance the efficiency of clinical trials by identifying suitable patient populations, optimising trial protocols, and predicting patient responses. However, compliance challenges arise, especially when dealing with vulnerable populations like children or the elderly.
Post-market surveillance and pharmacovigilance: AI can be instrumental in post-market surveillance to detect adverse drug reactions and monitor drug safety. However, complying with reporting obligations and ensuring seamless integration of AI-based surveillance systems with existing pharmacovigilance processes is essential to meet regulatory requirements.
Interoperability and standardisation: The pharmaceutical sector involves various stakeholders, including drug manufacturers, healthcare providers, regulatory bodies, and researchers. To ensure effective collaboration and compliance, AI systems must be developed with interoperability and standardisation in mind. This will facilitate data sharing and streamline regulatory reporting processes.
Continued monitoring and auditing of AI systems: AI algorithms used in pharmaceutical applications can evolve over time due to updates, retraining, or new data inputs. To maintain compliance, continuous monitoring and auditing of AI systems are necessary to detect any deviations from the intended use or regulatory standards.
Ethical AI governance: Apart from regulatory compliance, ethical considerations play a significant role in the use of AI in healthcare. Implementing robust AI governance frameworks that prioritise patient welfare, fairness, and transparency is essential to build trust with stakeholders and ensure compliance with ethical principles.
Patient-centric approach: Compliance in the pharmaceutical sector should align with a patient-centric approach. AI applications should be designed to benefit patients directly, leading to improved diagnostics, personalised treatments, and better overall healthcare outcomes.
Safeguarding intellectual property: In AI-driven drug discovery, protecting intellectual property becomes crucial. Compliance efforts should include measures to safeguard AI-generated innovations and ensure their proper patenting and licensing.
Cross-border regulatory challenges: Pharmaceutical companies operating in multiple countries may encounter variations in AI-related regulations. Tighter compliance requires adapting AI applications to meet the specific requirements of each jurisdiction without compromising patient safety or data privacy.
In conclusion
Embarking on the journey of integrating AI into the pharmaceutical industry can be both exciting and daunting. To harness the full potential of artificial intelligence in pharma, it is essential to lay a strong strategic foundation. A well-crafted AI strategic blueprint tailored to the specific needs and goals of each pharmaceutical company is the key to success.
This blueprint should encompass clear strategic goals and align these with the areas where AI can be most impactful for both the organisation and the business units. Then, an additional analysis must be made so that the final AI solutions chosen have high feasibility of success, a high impact on the organisation, and a strong cost-benefit analysis.
With the expertise and experience in data-driven AI solutions, trusted partners can play a pivotal role in guiding pharmaceutical companies on this transformative path. Empowering organisations to develop a comprehensive AI strategy that maximises efficiency, improves decision-making, and ultimately revolutionises the pharmaceutical landscape, such collaborations can unlock the potential of AI, leading to breakthrough innovations and advancements that drive the future of medicine.