How AI and predictive analytics are set to transform patient care
The healthcare industry is on the cusp of change, with artificial intelligence (AI) and predictive analytics paving the way for unprecedented advancements in patient care and operational efficiency. This evolution is being driven by the burgeoning amount of healthcare data that is being collected and made available through electronic patient records (EPRs), alongside the urgent need for systems that can analyse and leverage this information in order to improve patient outcomes and resource management.
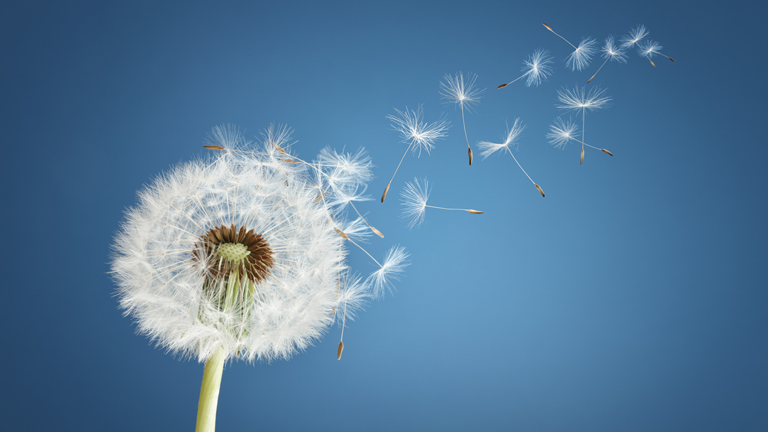
In the past, clinicians primarily used healthcare data as a decision support resource to pull in basic information on the number of medication errors that had occurred over a given time period, for example, and to use that basic intelligence to shape the clinical choices that were made.
Today, the technological capabilities available to healthcare teams have ramped up several levels. No longer just theoretical concepts in a healthcare context, AI and predictive analytics are now proving themselves to be critically important in tackling some of the most pressing healthcare challenges. From reducing medication errors through advanced decision support systems to predicting antibiotic resistance with sophisticated AI algorithms, these technologies are setting new standards for care quality and safety.
Moreover, their application in public health surveillance and chronic disease management is showing promise in pre-empting health crises, and improving long-term health management.
The benefits of these technologies are increasingly tangible. For example, initiatives that bring together vast volumes of patient data across different healthcare providers have demonstrated significant improvements in operational efficiency, and patient engagement. Equally, they have proven to be beneficial in terms of healthcare coordination. Insights about a particular patient can be made available quickly to help inform a 360-degree treatment plan for the patient, with care and social workers, dietitians, GPs, and hospital doctors all coming to together to discuss the evolution of the plan.
Analytics can also play a key role in patient surveillance, helping to ensure that a patient diagnosed with hypertension, for example, is taking their medication as prescribed, and rapidly analysing the blood pressure readings they take at home to ensure that no dangerous patterns are emerging.
AI in oncology: Enhancing care across the board
No area is perhaps more illustrative of these multiple benefits than oncology, the branch of medicine that deals with the prevention, diagnosis, and treatment of cancer.
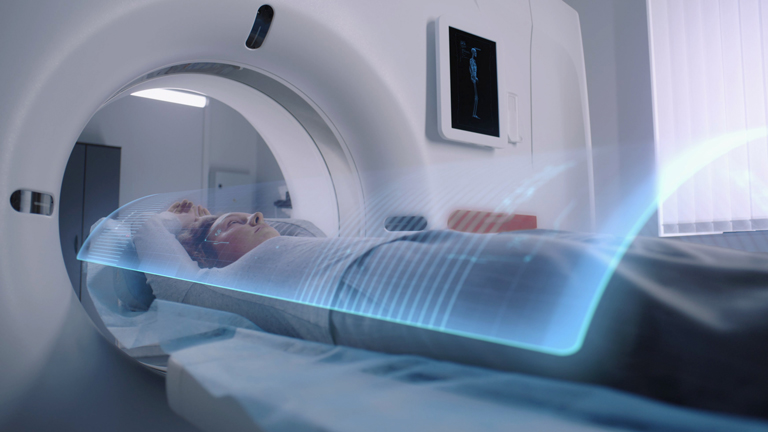
AI and predictive analytics are crucial in oncology due to their ability to manage and address the vast complexity and variability inherent in cancer treatment and tumour biology.
Enhancing diagnostic precision
The enhancement of diagnostic precision is a standout application of AI in oncology. AI-powered machine learning models are adept at parsing complex imaging data, identifying subtle patterns that are beyond human visual detection, and pinpointing early signs of malignancy.
This has notably improved the accuracy of diagnostic procedures, such as mammograms in breast cancer screening, significantly reducing both false positive and false negative results. Early detection facilitated by AI not only increases the likelihood of successful treatment, but also substantially improves the survival rates among cancer patients.
Tailored treatment with predictive insights
One of the primary benefits of AI in oncology is its ability to analyse large volumes of data quickly and with high precision. EPRs, which contain detailed patient histories, treatment outcomes, and genetic information, produce an extensive dataset that, when analysed on its own or through AI algorithms, can reveal patterns and correlations that may not be visible to human analysts. This capacity for deep data analysis helps in understanding the unique progression of cancer in each patient and forecasting their response to different therapeutic strategies.
Beyond diagnostics, AI can help in identifying the most effective treatment plans based on historical data from similar cases. This is especially beneficial in oncology, where the effectiveness of treatments can vary widely among patients due to the genetic differences in tumours. By predicting which treatments are likely to be most effective for a specific patient, AI not only enhances the likelihood of successful outcomes, but also minimises the risk and severity of potential side effects.
Furthermore, predictive analytics can anticipate patient responses to certain medications or treatment regimes, based on their genetic makeup, lifestyle, and previous health records, which allows for adjustments to be made before adverse reactions occur.
Improving operational efficiency
AI also boosts operational efficiency in oncology departments. By optimising scheduling and resource allocation through predictive analytics, hospitals can ensure that their operations run more smoothly, reducing patient wait times and increasing satisfaction. This is particularly key with cancer care, where patients will frequently visit multiple different healthcare settings for their treatment; from oncology management to different chemotherapy and radiotherapy sessions that they need to carry out.
Moreover, AI enables continuous monitoring of oncology patients, providing healthcare professionals with real-time updates on patient conditions. This prompt response capability is particularly critical for oncology patients, who may experience rapid changes in health status.
Learning from clinical trials
Another notable application of AI in oncology involves clinical research and trials. AI models expedite the identification of suitable clinical trial candidates and monitor patient responses during trials, allowing for quicker adaptations and potentially speeding up the approval of new cancer treatments. Additionally, AI systems help manage vast amounts of research data, aiding in the discovery of new oncological treatments and therapies.
Expanding the horizon – The future of AI in oncology
The potential of AI and predictive analytics extends beyond current applications, promising to bring about even more sophisticated tools for diagnosis, treatment planning, and patient management in oncology. These tools are expected to increasingly aid in the detection of early-stage cancers, predict disease progression, and personalise therapy to an unprecedented degree.
The integration of AI and predictive analytics into oncology represents a major leap forward in the quest for precision medicine. These technologies offer the promise of significantly improving how cancer care is delivered by enabling more accurate diagnoses, personalised treatments, and efficient resource management.
As healthcare continues to evolve, the role of AI and predictive analytics will only grow, underscoring the need for ongoing investment in these technologies. In light of these ongoing developments, the future of oncology care looks increasingly positive, promising better outcomes for patients worldwide. Through such advancements, the medical community is set to transform the landscape of cancer treatment, making it more effective, efficient, and patient-centred.
About the author
Rami Riman is director of clinical and business improvements at InterSystems. Prior to joining the company, he gained a decade of clinical experience across multiple hospitals in the US, UK, Lebanon, and UAE.
Riman’s passion remains in increasing patient engagement to improve community’s health and awareness. He holds a BA from the American University of Beirut in Biology, a Doctorate in Medicine from St. George’s University School of Medicine and a Master’s degree in infectious diseases from the University of London. He is also board certified in Internal Medicine and Medical Oncology.
Supercharge your pharma insights: Sign up to pharmaphorum's newsletter for daily updates, weekly roundups, and in-depth analysis across all industry sectors.
Click on either of the images below for more articles from this edition of Deep Dive: Oncology 2024