Experimental AI predicts whether cancer drugs will work

Researchers in the US have developed an artificial intelligence tool that can predict how a patient will respond to cancer treatment, bringing the concept of personalised therapy a step closer to reality.
While plenty of targeted therapies are available against specific cancer mutations, only a minority of patients can benefit from them. The National Cancer Institute (NCI) team and the Sanford Burnham Prebys research organisation hope their computational approach could eventually be used systematically to match patients to the best available treatment.
Their AI platform, called PERCEPTION, focuses on the transcriptome – a collection of all the mRNAs expressed in a cell – and was trained on publicly available data taken from large-scale studies that screened drugs against cancer cell lines.
Current approaches to matching patients to drugs rely on bulk sequencing of tumour DNA and RNA, which takes an average of all the cells in a tumour sample, according to the NCI. However, tumours can have many different cell types, and researchers believe these subpopulations may respond differently to specific drugs.
That could explain why some patients do not respond to certain drugs or develop resistance to them.
To take that into account, the PERCEPTION developers have used a machine learning technique known as transfer learning to train the AI to predict drug responses using bulk RNA sequencing data, but also refined the model using single-cell RNA sequencing data.
“In contrast to bulk sequencing…single-cell RNA sequencing provides much higher resolution data,” according to the NCI. “Using this approach to identify and target individual clones may lead to more lasting drug responses.”
There’s a problem with that, however, as generating single-cell gene expression data is much more expensive and not yet widely available outside of research labs.
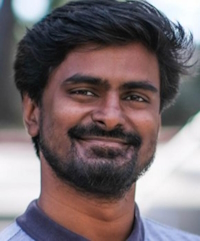
Sanju Sinha of Sanford Burnham Prebys, who is the lead author of a paper on the technique published in Nature Cancer, said that limited access to single-cell data from clinics was the biggest challenge facing the project. However, even the modest amount available was enough to demonstrate the potential of the AI.
“A tumour is a complex and evolving beast [and] PERCEPTION allows for the use of rich information within single-cell omics to understand the clonal architecture of the tumour and monitor the emergence of resistance,” said Sinha.
“The ability to monitor the emergence of resistance is the most exciting part for me,” he added. “It has the potential to allow us to adapt to the evolution of cancer cells and even modify our treatment strategy.”
The AI has been validated by predicting the response to monotherapy and combination treatment in three independent, recently published clinical trials involving patients with multiple myeloma, breast, and lung cancer.
In each case, it was able to correctly distinguish between responders and non-responders based on their transcriptome data. The scientists were also able to show that if just one cell type was resistant to a particular drug, the patient would not benefit from that treatment, even if all the other types responded.
PERCEPTION is not ready for clinical use, but the study shows that single-cell information can be used to guide treatment and could encourage greater use of single-cell RNA sequencing in clinics, which could then be used to train the AI even further.
The team has made the model available via a website to make it easier for other groups to use it with additional datasets.