Why AI equity is the next step for transformational pharma
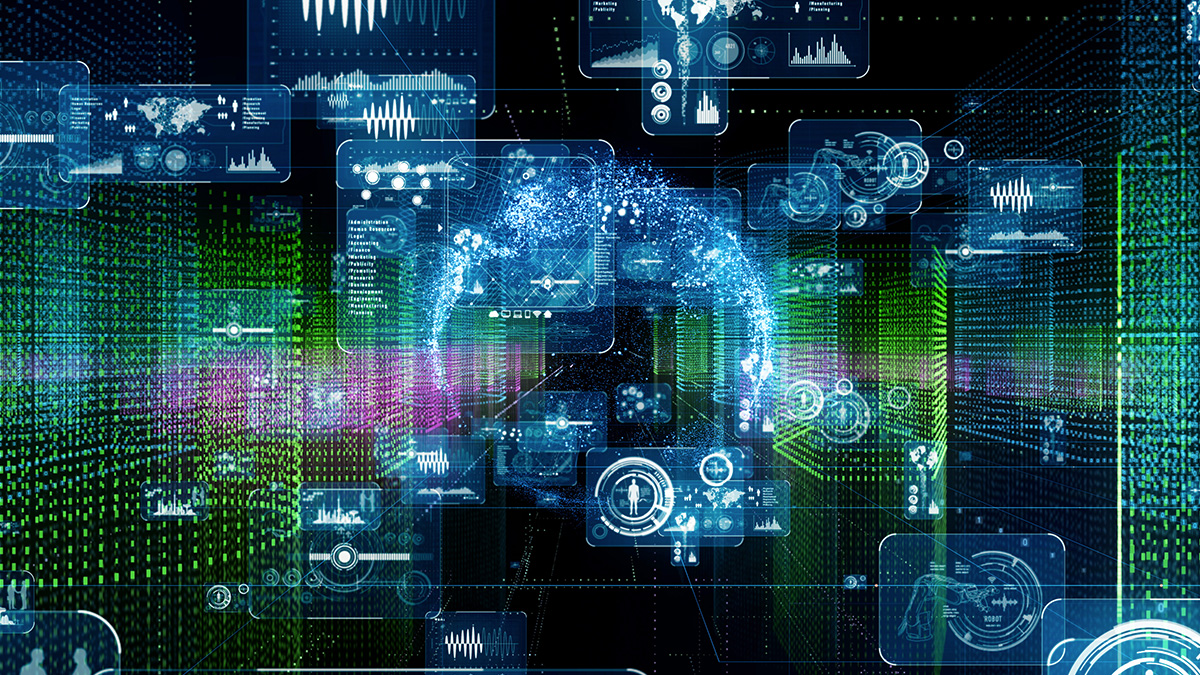
AI can be transformative for all areas of healthcare, but often these systems are built off biased datasets that don’t reflect the true diversity of the general public – leading to approaches that only work for specific populations. Intouch Group’s Abid Rahman explains this concept of AI equity and gives some practical tips for how companies can work towards it.
It might be tempting to think that because AI tools are machines, they are free from the subconscious biases and diversity issues that have long been problematic for clinical research. But in truth these technologies are usually built off datasets that reflect human bias, which can in turn affect how an AI system operates.
Because of this, there are increasing calls for ‘AI Equity’ to be promoted across the industry.
“AI Equity really refers to how effective AI is in a broad range of scenarios with a variety of population groups and demographics,” says Abid Rahman, vice president of innovation at Intouch Group.
Rahman notes that this requires taking into account the biases that often are automatically present in data. He notes, though, that he has never run into a situation where this has been intentional.
“The bias in the data is often exposed when companies run tests using models created from the data. Often AI is simply uncovering what was already there – it’s not necessarily creating a new problem, but it can enhance a problem that already exists.”
These tests might involve identifying the groups of people who will be most affected by an AI system, then finding the right data sources with which to train the algorithm.
“The data itself can often be biased, so it's important to match it up with the demographics who are ultimately going to use the system that you are creating.
“Hospitals or other systems that generate this data have patient populations who are typically wealthy, more connected, and with more access to digital systems. In other words, they are skewed towards populations who already have access – which leads to systems that are skewed in that way as well.”
One case study Rahman uses comes from the US, where some AI systems have shown that African American populations are often healthier than Caucasian populations.
“However, the datasets didn't have enough African-American data to begin with,” he says, “particularly in terms of African-American women, and was therefore severely flawed.”
One example of an approach that seeks to overcome such variances in data comes from researchers at MIT, who have developed a deep learning system that can predict a patient’s breast cancer risk leveraging only the person’s mammograms, with the hope that this means it will work equally effectively regardless of race or ethnicity.
Nonetheless, Rahman says that these kinds of AI inequities are still a widespread problem.
“There are thousands of pilot projects ongoing all over the world, and the more pilot systems that are created, the more widespread the problem becomes.”
Stringent regulations in healthcare mean that the sector often fares better in data bias compared to what Rahman terms the ‘Wild West’ of AI in more general settings – but there is also more potential for harm if healthcare gets this wrong.
Rahman notes, for example, that during the COVID-19 pandemic, models created in one specific environment or country are often used to create policy in another country (often due to lack of resources) without consideration for how populations might provide different data.
“Imagine a new AI-generated COVID test that is 99% accurate with a white Caucasian population and only accurate 40% of the time with South East Asian populations. Millions could potentially become very ill.
“Likewise, if a drug is developed using genetic data from a certain group of people, and it does not work with the same level of efficacy for other populations, that would be very problematic and potentially even harmful for those other groups.”
An additional effect would be reduced trust in both AI and healthcare systems in general.
“If a model is proven to be wrong, it's going to be hard to get people to trust the next model that comes along,” says Rahman.
Making AI equitable from the ground up
To implement AI in an equitable way, Rahman says, companies need to have the right experts on their teams.
“Often what happens, especially in pilot projects, is that companies have experts who know a lot about the technology but not enough about the demographics of the end users and their needs. It's important to have domain experts involved in the project from the beginning.”
Another challenge is having access to the right data in the first place.
“There’s lots of data out there, but just having large volumes of data doesn't mean that the issue of bias is already taken care of,” Rahman notes.
“Likewise, it can be tempting to use certain algorithms simply because they are easy to implement – but, again, it's important to look at a variety of options and the pros and cons of each one to find the right AI algorithm for you. Then when you validate those algorithms, you need to do so with the right sets of test cases.”
From there, it’s important to think about who will have access to the project pilot.
“It’s easy to release to a pilot population who already have access to such technology and are primed in other ways to benefit from the technology – but companies need to make sure that the pilot group also represents the variety within that population who will ultimately use it,” Rahman says.
All of this can be more time- and resource-intensive than companies might have expected, which can be a barrier – but Rahman notes that if companies don’t start an AI project in the right way, they will end up with built-in issues in the system.
“They need to focus on the ultimate benefit rather than the immediate return on investment (ROI). Being impatient with ROI can lead to suboptimal systems in the long term.”
Benefits for humanity
But despite these teething issues, Rahman is generally positive about how AI is shaping the future of pharma and healthcare.
“Electronic health record (EHR) systems have started to use AI-based platforms that can recommend the right treatment pathway for a patient depending on their profile. Meanwhile, AI is aiding drug discovery by screening data to predict the properties of a potential compound, generate ideas for novel compounds, and save time for researchers.”
He notes that most successful tech companies, e.g., Google and Facebook, are really AI companies – and that this is a shift pharma is likely to see too.
Ultimately, Rahman says that AI equity is important because AI is “here to stay”.
“It really is going to become embedded in every facet of our lives. The transformative value it can create will be comparable to the difference between doing research pre-Google and post-Google, or communication pre-internet and post-internet. We’ll see massive benefits of AI for humanity as it becomes easier to create treatments for specific populations based on their needs, their behaviours, and their genetic makeup.
“For us to be able to realise those benefits, there has to be a recognition that equity of access is a real challenge. Luckily, many companies are already cognizant of that, and we’re seeing more and more top-down approaches to creating equitable environments within AI and drug development.”
About the interviewee
Abid has over 19 years of experience in software engineering and over 16 years in pharmaceutical marketing and technology. As an innovation leader, Abid’s primary role involves making technology relevant in healthcare by designing and collaborating on solutions for patients, caregivers and healthcare providers. His technology expertise is in architecture and design of enterprise solutions with emphasis on practical implementation of Artificial Intelligence. Abid leads the development of Cognitive Core, an AI platform created for the life sciences industry. In addition, Abid is also focused on leading up innovation labs, R&D, proof-of-concepts, new product development and open innovation partnerships.
About Intouch Group
Intouch Group is a privately held full-service agency network, providing creative and media services, enterprise solutions and data analytics globally through seven affiliates in eight offices, including Intouch Solutions, Intouch Proto, Intouch Seven, Intouch International, Intouch Media, Intouch B2D and Intouch Analytics. Collectively, Intouch Group employs more than 1,000 people. With a dedication to the life sciences, Intouch Group operates with the belief that there is no challenge too big to cure. Contact Intouch Group at info@intouchg.com or visit them on the Web at intouchg.com.