How can we reliably discover the biomarkers we need to achieve stratified medicine?
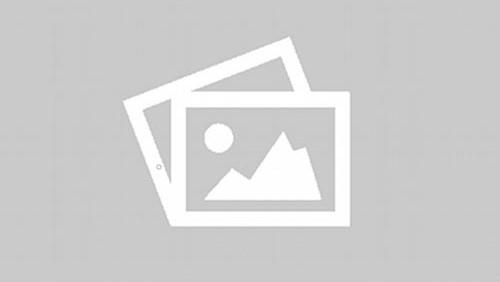
The burgeoning field of stratified / personalised medicine is reliant upon assessing patients using accurate predictive biomarkers – but how can we maximise the likelihood that our research projects will successfully discover biomarkers which can be brought into clinical practice? Ben Chaffey from Biosignatures Ltd. discusses.
Introduction
It's widely recognised that a sea-change is taking place in healthcare. The historical approach of classifying cases of disease within broad categories and following empirical treat-and-observe therapeutic strategies is being replaced by a more targeted form of medical practice, which aims to characterise and treat illnesses on the basis of progressively greater understanding of their underlying biological causes. This is known as 'stratified' or 'personalised' medicine.
Stratified medicine is highly dependent upon biomarkers; signature characteristics of a particular condition which can be used to enhance diagnosis, predict likely clinical progression and guide treatment choices.
The benefits that a stratified approach to healthcare can bring have become particularly apparent in the field of oncology, where the treatment of certain conditions has been transformed by utilising companion pairs of assay and therapeutic. Patients who are most likely to benefit from the use of particular drugs are identified by testing their tumours for the presence of specific genetic biomarkers which predict likely response, before a therapy is commenced.
"The benefits that a stratified approach to healthcare can bring have become particularly apparent in the field of oncology..."
Good examples of this are Zelboraf, a selective inhibitor of the V600E/K mutant form of B Raf kinase found in ~60% of melanomas, which can be assayed for using the Cobas BRAF V600 mutation test (developed by Plexxikon and Genentech / Roche) and the companion pair of Herceptest (Dako / Agilent) and Herceptin (Genentech / Roche) used to identify and treat HER2 receptor +ve breast cancer.
Other clinical areas have also benefitted from personalisation, albeit to a lesser extent to date, with assays such as Trofile (Monogram Biosciences) guiding therapy with Celsentri (Pfizer) for HIV infection and mutation analysis of the HNF1A gene informing treatment of diabetes with sulfonylurea drugs.
These examples demonstrate the benefits which can be provided by reliable biomarker assays that are capable of accurately predicting a patient's response to a therapy.
The task now before the diagnostics and pharmaceutical industries is to maintain and preferably speed up the rate at which novel biomarkers can be discovered, validated, qualified as having clinical value and, ultimately, introduced into routine use. Unfortunately, this process has historically proven to be a significant challenge.
The nature of the biomarker problem
Maintaining the development of stratified medicine requires a pipeline of novel biomarkers which are diagnostic and prognostic of illnesses that cause significant morbidity and mortality, particularly the chronic conditions which have an increasingly large impact upon the aging global population such as cardio-vascular and neuro degenerative disorders.
The research literature is littered with examples of studies that claim to have identified biomarkers for almost every type of disease known to medical science, including many for the conditions mentioned. Despite this, precious few have actually reached clinical practice.
To date, most stratified medicine successes, such as those cited above, have centred upon one particular factor, such as mutation of a specific gene / protein or the tropism of a viral population. Whilst this has certainly not been a trivial undertaking, simpler systems are inevitably easier to work with.
"To date, most stratified medicine successes, such as those cited above, have centred upon one particular factor..."
In order to broaden the range of conditions to which a stratified approach to healthcare can be applied we must tackle the challenge of biomarker discover and development in illnesses which often have a complex and currently incompletely understood underlying pathology; this makes the task very difficult.
In an effort to circumvent gaps in biological knowledge, many biomarker discovery projects, particularly those conducted in conditions which are considered unlikely to have a single underlying cause such as a specific mutation, have employed hypothesis-free 'omics technologies to search for changes in a wide variety of analytes in the expectation that some will be discovered which are highly characteristic of a particular disease.
Whilst an appealing concept, this approach comes with its own particular set of complexities and difficulties which must be fully understood and mitigated against if a project is to stand a chance of success.
Where have we been going wrong?
Discovery, validation and clinical qualification of novel biomarkers using 'omics approaches are a challenging undertaking. Unfortunately, all too often researchers have carried out studies which, with the benefit of hindsight, were compromised from the outset.
Common problems which have historically beset biomarker discovery projects have included:
• Failing to appreciate the degree of natural variability which may be present within a patient population – this can lead to a failure to recruit sufficient participants to adequately statistically power the study.
• Comparing samples taken from patients with severe cases of the condition of interest with samples taken from healthy individuals – leading to the discovery of biomarkers of 'unwellness' rather than a specific disease.
• Failing to ensure samples have been both obtained from study participants and stored in a uniform and highly-controlled manner – studies have claimed to discover disease biomarkers but actually discovered markers of sample degradation which unfortunately correlated with different research sites, at which either predominantly cases or controls had been sampled.
• Failing to carry out adequate validation of initial results – discovery projects which are highly reliant on multifactorial analysis techniques can be very prone to 'overlearning' the training data set. This leads to an apparently high level of assay performance which cannot be subsequently achieved when analysing new data. The true performance of an assay can only really be known when it is assessed using samples which have never previously been analysed by this system.
So, how can we improve?
Significant amounts of time have been spent analysing the biomarker discovery, validation and qualification process in order to develop strategies and procedures designed to maximise the chances of a project reaching a successful outcome.
"One strategy is to take an engineering approach to biomarker discovery."
One strategy is to take an engineering approach to biomarker discovery. This essentially means breaking the problem down into discrete manageable chunks which can be worked upon using standardised approaches.
This brings with it the additional benefit that 'modular' activities can easily be combined with a minimal degree of modification, greatly speeding up study design and start-up processes.
It's 'industrialising' the research process to bring about the sustained, predictable generation of new clinical biomarkers.
Biomarker study recommendations
Stratified / personalised medicine won't happen without the development and implementation of new biomarker assays. This means that much more biomarker research – crucially, research which yields successful results – will be required in the near future.
"Stratified / personalised medicine won't happen without the development and implementation of new biomarker assays."
To maximise the chances of achieving a successful project outcome, ensure the following activities are all carried out:
• Characterise the performance of the chosen measurement platform: Carry out extensive characterisation – when the success of the entire project rests on the analytical values generated by an instrument, you have to be able to trust it behaves consistently, run-after-run-after-run. Statistical Process Control (SPC) methodologies are particularly helpful here and we have recently published two articles(2,3) discussing the application of SPC to research proteomics.
• Standardise all sampling and experimental procedures: Humans are extremely variable research subjects and unless the condition you are investigating has a single characteristic change (BRAFV600E, for example) the signal-to-noise ratio in samples will likely be low. Adding sample-handling variation onto this could severely compromise the ability of the study to generate meaningful results at all. Ensure suitable SOPs are in place and emphasise the importance of adhering to them when training staff!
• Understand the clinical population of interest: This sounds obvious, but you have to really understand the population to design an effective study. Candidate biomarkers discovered in samples taken from patients who are not representative of the final target group are much less likely to have the desired high performance in final validation and qualification trials. This may well prove terminal to the project.
• Utilise a pragmatic and flexible study design: There are many factors, such as actual recruitment rate and intra-cohort variability, which have significant impact upon the viability of a study but are difficult to accurately predict up-front. Designing studies which incorporate features such as interim assessment points and flexible overall recruitment targets will allow adjustments to be made in light of relevant observations made on the actual cohort of interest, such as updating statistical power calculations based on the true sample variation observed.
• Evaluate true assay performance using blind tests: By designing projects to include both discovery and evaluation phases, it is possible to perform a blind assessment of assay performance immediately after the discovery stage. This allows a more informed decision to be made by the research team themselves regarding further development and also demonstrates to third parties that the assay performance figures reported are reliable.
Concluding thoughts
Whilst biomarker research is undoubtedly challenging, trying to understand the nature of the problems it presents more completely and thereby designing and carrying out the best studies that we are able to will maximise the number of projects which successfully achieve their aims.
References
1) BiopSave Clinical Validation Trial: (http://www.controlled-trials.com/ISRCTN52361806)
2) Bramwell DI (2013). An introduction to statistical process control in research proteomics. J. Prot. http://dx.doi.org/10.1016/j.jprot.2013.06.010
3) Jackson D & Bramwell DI (2013). Application of clinical assay quality control (QC) to multivariate proteomics data: A workflow exemplified by 2-DE QC. J. Prot. http://dx.doi.org/10.1016/j.jprot.2013.07.025
About the author:
Ben Chaffey, Ph.D., is Clinical Operations & Business Development manager for Biosignatures Ltd. Since being awarded his Ph.D. in Applied Immunobiology by The University of Newcastle, UK, he has accumulated a decade of experience in both academic and industrial life-science research, development, sales, marketing and management.
When he's not running clinical research projects Ben spends his time building water rockets with his sons and exploring the countryside of North East England.
He Tweets @BenC_Biosigs and can be contacted by emails sent to ben.chaffey@biosignatures.com
How can we reliably discover the biomarkers we need to achieve stratified medicine?