Four steps to building an innovation culture in pharma
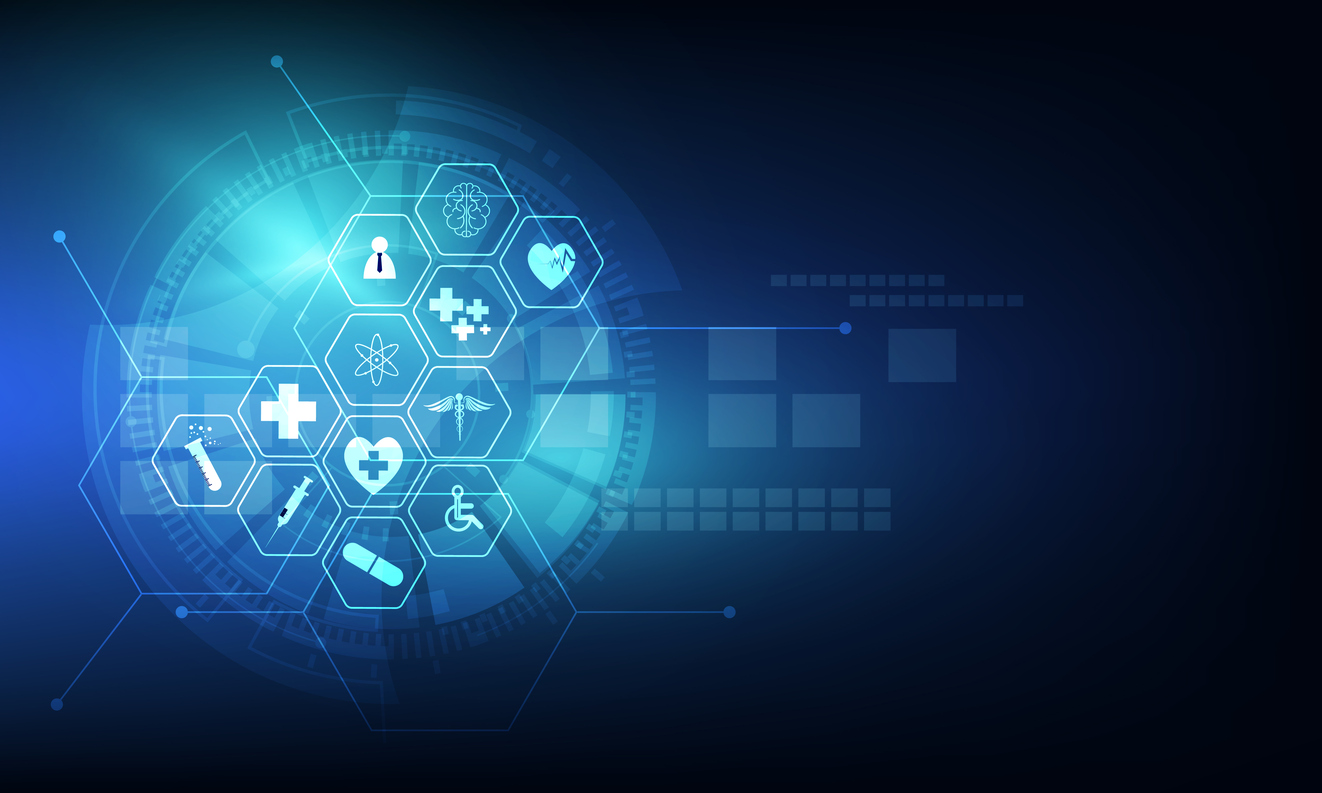
The quest for innovation is a major driving force in pharma. From the discovery of therapeutic biologicals in the 1920s, such as Salvarsan and insulin, to the blockbuster drugs birthed in the 1990s, such as Lipitor and Humira, pharma has always been on a quest for novel, groundbreaking drug development.
However, advancements in technology are constantly transforming how industries and thought leaders operate. Even though the desire for innovation is still at the core of pharma, the industry is still struggling with slower and less efficient traditional R&D models - and is consequently lagging behind in optimizing the benefits of new models of innovation. In order to remain competitive, pharmaceutical companies must learn to foster a culture of innovation at every level of their organization and at every stage of drug development.
Here are four things pharma companies can do to build a dynamic culture of innovation.
1) Be honest about failure
Most researchers want to learn from failure, but only a few are publicly transparent about failure. When a clinical trial fails to achieve the intended results, newer studies can benefit from the lessons learned in that failure. However, the researchers involved in the failed trial are not usually incentivised to share their mistakes or the lessons that they learned in the process.
Model predictions and studies such as 2016’s ‘Learning from successes and failures in pharmaceutical R&D’ in the Journal of Evolutionary Economics have clearly demonstrated that both successes and failures can contribute to pharmaceutical R&D department investment decisions. Consequently, there should be no shame in being honest about failure. In fact, the faster that pharma companies learn from their failures, the more successful they will be in bringing innovative drugs to market.
On average, it takes 12 years for drugs to go from pre-clinical testing to FDA approval, and it takes seven years for medical devices to go through the process. The journey to approval is too long and the increasing complexity of traditional clinical trials has contributed to the high cost of failure in drug development. Too much time passes before researchers have an opportunity to learn from failure, and the stakes are high.
In an article by Chorus, the authors contrast the traditional drug development model with an alternative model they call ‘quick win, fast fail’ and which places a greater focus on reaching proof-of-concept faster. Consequently, there are more chances to be successful, even when there are many failures along the way. In other words, failing fast (and more often) is a big catalyst for success.
In addition to failing fast, pharma firms need to find more efficient ways to create failure through experiments. According to Amy Edmondson, the Novartis professor of leadership and management at the Harvard Business School, one of the skills that company leaders should have is the ability to create failure through experiments.
But how can the pharma industry as a whole learn from these failures? It starts with companies being willing to incentivise the open sharing of lessons learned from failure. When a drug fails in research or in clinical trials, companies should incentivise researchers to publicise the failure so that others can learn from it. Individual researchers within the same company should not be territorial about their research but should share lessons learned from failure with one another. In an ideal world, this sharing of failures should also take place between different companies, though they would have to make a concerted effort to embrace this level of transparency.
2) Build data analysis as a core competency
In the past, the core competencies of pharmaceutical companies were research and marketing. Recently, data analysis has become a forerunner on the list of core competencies.
In order to be increasingly innovative in the discovery of novel drugs, pharma companies must be empowered to elevate their data analysis capacities. They need to exercise control over their own data analytics and not merely rely on an external organisation to help them leverage their own data. That’s why we don’t simply sell a product or service - we sell an ecosystem that empowers in-house analytics teams to work faster and smarter.
In-house data science teams with advanced analytics capabilities are becoming increasingly common in pharma. As with any new competency, learning quickly will support the effectiveness of these capabilities. Pharma will need to learn to reduce the time-lag in data analysis, emulating other industries that work with real time data and insights.
Companies must become competent with not just extracting the data points and sets available to them, but also with explaining the relationships and connections between disparate data points and indicating smart ways to use the data. This is no easy task, but there is a recent emergence of tools, such as our iPlexus platform, that empower data analytics within a company to find these relationships and relevant insights.
Pharma companies must also become adept at network analysis. We are now in the age of the algorithm, precision medicine and data-driven analysis. Pharma firms need to take advantage of these new technologies. They must learn to parse data in new ways. For instance, the use of ‘low-code’ (designing and developing new software by relying on libraries, APIs and third-party infrastructure) can help pharma companies meet their needs faster. With low-code, they would be able to create something new without coding from scratch. One of the benefits low-code can give pharma companies is ensuring that they bring drugs to the market faster.
Additionally, to catalyse innovation, pharma firms need to use tools such as AI and machine learning to make sense of complex data and inform decision making. Clinical researchers know that accuracy, repeatability, and reproducibility are important in bringing a drug to market, however pharma companies still rely heavily on human input to achieve high-volume tasks. Low-code can be used together with robotic process automation (RPA) and artificial intelligence (AI) to leverage data and create innovative products.
3) Encourage cross-functional collaboration
Data silos and lack of open data are big challenges in pharma. Pharma companies should incentivise researchers to share insights with one another and to collaborate on projects.
They need to engage more in new open innovation models that encourage sharing of data without the risk of losing intellectual property. Examples of open innovation models are open sourcing, crowdsourcing, public-private partnerships (PPPs), collaborations with academic centers and outsourcing to virtual R&Ds.
Let’s briefly identify the roles each of these models play in achieving innovation in pharma:
- Open sourcing allows pharma to gain knowledge from the public, especially in drug development for neglected diseases
- Crowdsourcing is similar to open sourcing, but instead of getting ideas from the general public, crowdsourcing solicits the skills of experts in the field
- PPPs allows companies to combine resources and share risks
- Recruiting experts from academic centers can help solve complex problems
- Virtualisation of R&Ds helps companies keep their focus on core technologies while partnering with virtual firms that help them pursue under-utilised tasks.
Due to these open innovation models, traditional R&D models are progressing from internally-driven to externally-driven prototypes. Although this transition has caused major pharma companies such as Merck, AstraZeneca and Pfizer to downsize their internal R&D departments, open innovation has produced measurable benefits for pharma in terms of innovation. In a recent Bain & Company survey, 91% of surveyed executives recognised that they need to increase their company’s capacity for innovation because it is “critical to creating future competitive advantage and earning profits”.
4) Automate lower-level cognitive tasks
To focus on creativity and innovation, researchers in pharma need to spend less time performing lower-level cognitive tasks, such as administrative work and collecting, cleaning, and organising data. Performing these tasks limits thinking outside the box. Researchers need a larger brain bandwidth to achieve ‘Aha!’ moments.
Research by McKinsey and Company shows that about 45% of tasks people are paid for can be automated using technology. Examples of automation technologies within pharma and healthcare include IBM’s Watson and Innoplexus’ iPlexus platform.
Using automated systems allows researchers and pharma companies to focus on higher-end creative tasks. It can yield more meaningful work and, in turn, can catalyse innovation.
By taking steps towards incentivising the sharing of failure, building data as a core competency, encouraging cross-functional collaboration, and automating lower-level tasks, pharma companies can build the culture of innovation that they need to remain competitive in this dynamic field.
About the Author:
[caption id="attachment_42968" align="alignleft" width="106"] Lawrence Ganti, Innoplexus[/caption]
Lawrence Ganti is CEO|Americas of Innoplexus an artificial intelligence based data analytics firm specializing in the lifesciences. Prior to joining Innoplexus, Lawrence spent 15 years leading teams across functions and geographies in the Pharmaceutical industry. He also worked as a research associate for McKinsey & Company and holds an MBA from IMD in Switzerland and a Bachelors from Babson College in the United States.