Big data enables the promise of personalised medicine
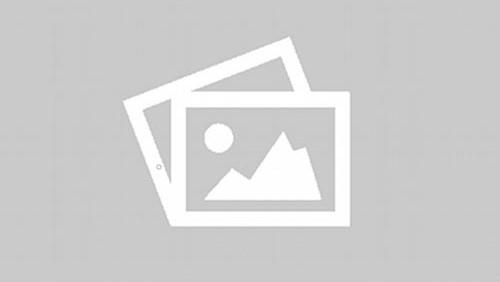
As our personalised medicine month continues, Joel Haspel and Ketan Patel discuss big data and its role in enabling personalised or 'stratified' medicine, as well as the challenges it brings. They also look at how industry leaders can make the most of next-generation diagnostic technology.
Health sciences and big data are inextricably linked. This inherent connection grows more critical by the day and holds the key to healthcare transformation and the realisation of truly personalised medicine. The challenge for health sciences organisations ‒ as data volumes and sources explode within and beyond their enterprises ‒ is to effectively, securely, and cost-efficiently leverage and analyse this information to deliver new levels of actionable insight. We are, at long-last, reaching an important tipping point in this elusive quest.
Data, data, everywhere
The last decade has seen an explosion in health-related data. As individuals, we generate a tremendous data trail in our daily lives through interaction with our personal devices and the world around us. This information can yield important correlations between factors such as our genome, diet, lifestyle, and family history and lead to better prediction, diagnosis, and treatment of diseases. In addition, genetic testing is increasingly common and sequencing of multiple genes or even a person's complete genome is becoming more affordable and, therefore, more viable as a clinical tool.
"As individuals, we generate a tremendous data trail in our daily lives through interaction with our personal devices and the world around us."
The promise of personalised medicine
The promise of personalised medicine is to give the right drug to the right patient at the right time. However, this isn't as easy it sounds. State-of-the-art drugs for many diseases are not effective in up to half of their target population.
There are several factors driving the pursuit of personalised medicine:
1. A clear signal from payers and governments that new treatments must be differentiated and achieve better clinical outcomes than existing treatments. This reality is driving pharmaceutical companies to develop drugs that are population-specific and enable providers to better predict outcomes.
2. Greater accuracy at diagnosing a disease. Genetic tests can more precisely tell us what is going on than signs and symptoms alone, particularly for genetic-linked diseases like cancer. More accurate tests mean less trial and error as well as fewer patient visits.
3. Cost containment with better clinical outcomes. Many health systems are struggling to keep costs under control. Genetic testing aims to reduce the amount of trial and error in diagnosing disease as well as prescribing the right treatments. Comparative effectiveness studies aim to understand which drugs work for which patients, and lower the amount spent on needless or inappropriate treatments.
Information management challenges for clinical genomics
As the cost of DNA sequencing continues to decrease and technological advances make sequencing easier and more robust, the bottleneck in translational research has shifted from data gathering to data analysis. Due to poor informatics infrastructure design, it has been hard for bioinformaticians to build tools that enable researchers to be self sufficient in their standard analyses. This has created an environment in which highly skilled bioinformaticians are required to perform mundane tasks, diverting their time and focus away from challenges and innovation. This ad-hoc analysis paradigm often leads to scattered data and analysis files across multiple storage devices. As a result, it is difficult to reproduce results and transfer knowledge to external collaborators in accordance with regulatory requirements. In the past, some institutions have contracted consultants to build customised solutions. These environments, however, take a long time to build and can become a maintenance challenge due to high-cost and long-term contracts for external expertise. They also create disconnect in knowledge between contractors and in-house resources.
"...the bottleneck in translational research has shifted from data gathering to data analysis."
Most informatics infrastructures today focus on a single omics technology vendor (for example, Illumina or Life Technologies) or a single omics data modality (for example, genome versus transcriptome). While useful, this approach provides a fragmented picture of the underlying biological processes. Overwhelming evidence shows that an integrated approach is the key to identifying the root cause of a disease based on gene structure, expression, and regulation across multiple omics modalities 1-5. For instance, an integrated analysis of cross-modality glioblastoma (GBM) data, including DNA copy number, gene expression, and DNA methylation aberrations, helped dissect genome-wide regulatory mechanisms for further investigation into the identification of candidate biomarkers for GBM tumors and potential therapeutic targets5. A holistic view of cross-vendor and cross-modality data is, thus, critical for the development and delivery of targeted medicine.
On the clinical front, many translational research projects disproportionately invest in molecular profiling technologies compared to the clinical information collection systems, which is commonly done through a project-specific case report form. Without heavy and consistent post-processing, clinical data reuse and comparisons across projects are impossible. As translational research moves toward the bedside, the sources of clinical data will continue to expand beyond case-report forms, and will include electronic health records, state or national registries, and records from various departments, such as accounting, pharmacy, and pathology. From an informatics perspective, translational research tools need to support the integration and standardisation of clinical data from disparate sources, with the help of consistent terminology and units of measure. Once this data is standardised, it will not only benefit translational research but also the analysis of other metrics, such as cost effectiveness and real-world evidence of clinical outcomes. This capability will greatly benefit pharmaceutical companies that would like to connect controlled clinical trial data to real-world data on drug performance.
Industry leaders make the most of next-generation diagnostic technology
Major academic medical centres are exploring how to integrate whole genome-based testing into their clinical practices. Most have begun with the move toward electronic medical records. These systems are now a part of a clinician's daily workflow and represent a rich source of clinical phenotypic data.
As use of gene sequencing grows, forward-looking organisations are beginning to complement sequencing technology with ancillary omics environments to securely store molecular genomic data and allow analysis of genotype-phenotype relationships. These analytical platforms are extremely useful for research and will allow physicians to use this rich source of data to improve clinical care.
A central aim of these robust analytics initiatives is to provide an evidence base for reimbursement. Sometimes a patient is given the wrong treatment or is over treated. Companies like Genomic Health have built a large evidence base around a genetic test for a specific disease, which enables them to persuade payers to reimburse for these tests. A key goal of analytics projects is to determine whether or not genetic testing can actually save money for the entire healthcare system.
"Major academic medical centres are exploring how to integrate whole genome-based testing into their clinical practices."
For diagnostic companies, it is important to know how many patients should be eligible to receive the test and how many actually got the test and why. Integrating a rich source of clinical and genomic data can also be used to help clinicians develop a treatment protocol for individual patients. For example, a clinician can search the database for patients similar to a specific case and then quickly drill down to the most effective treatments for previous patients with the same clinical and genomic characteristics. While these systems cannot yet provide the physician with guidance on which is the best treatment for a specific patient, they provide an additional tool to deal with difficult cases and help physicians decide whether to enroll the patient in a new clinical trial for an experimental medicine.
Conclusion
As organisations move to advance translational research to achieve personalised medicine, they require fully integrated, robust, and easy-to-use informatics solutions that let researchers and clinicians aggregate, store, and analyse clinical and omics data from diverse internal and external sources, including public consortiums. We are seeing the emergence of these solutions today, which allow researchers to stratify patients and clinicians to evaluate treatment response for similar patients in a self-sufficient manner. This type of solution will play an important role in accelerating the biomarker development cycle and advancing personalised medicine.
References
1. Integrated genomic analyses of ovarian carcinoma. Nature, 2011. 474(7353): p. 609–615.
2. Masica, D.L. and R. Karchin, Correlation of Somatic Mutation and Expression Identifies Genes Important in Human Glioblastoma Progression and Survival. Cancer Research, 2011.
3. Sumazin, P., et al., An Extensive MicroRNA-Mediated Network of RNA-RNA Interactions Regulates Established Oncogenic Pathways in Glioblastoma. Cell, 2011. 147(2): p. 370–381.
4. Verhaak, R.G.W., et al., Integrated Genomic Analysis Identifies Clinically Relevant Subtypes of Glioblastoma Characterized by Abnormalities in PDGFRA, IDH1, EGFR, and NF1. Cancer cell, 2010. 17(1): p. 98–110.
5. Comprehensive genomic characterization defines human glioblastoma genes and core pathways. Nature, 2008. 455(7216): p. 1061–1068.
About the authors:
Joel Haspel currently leads the EMEA Healthcare Strategy for Oracle Health Sciences. Leveraging his 25 years of consulting and IT experience, of which 14 have been exclusively focused on healthcare, Joel works closely with the Oracle Health Sciences Network, Enterprise Health Analytics, Translational Research Center and Health Information Exchange product strategy teams. Prior to joining Oracle he served as CEO of Sentient Health, an innovative Healthcare supply chain and analytics software provider, and was a Senior Manager at Deloitte Consulting.
Dr. Ketan Patel is currently a Healthcare Solutions Consultant with Oracle Health Sciences working on Translational Medicine solutions. Prior to this post, he led multiple teams and projects both at Pfizer and at Lilly working on Translational Bioinformatics in the fields of Oncology, Diabetes and Inflammation over a 9 year period. Dr. Patel holds a PhD in Bioinformatics from the University Of Oxford and an MSc in Artificial Intelligence from the University of Edinburgh.
How can pharma make the most of next-generation diagnostic technology?