Artificial intelligence in drug discovery and diagnosis
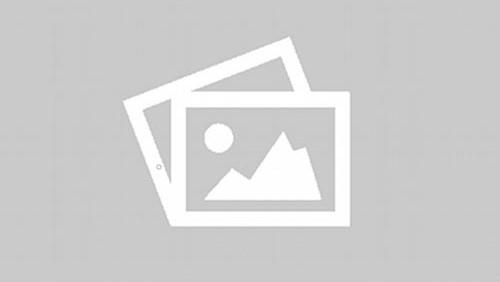
Machine learning is widely predicted to make drug discovery and patient diagnosis quicker, cheaper and more effective in the future, and signs of this can already be seen.
Nearly 70 years ago, artificial intelligence researchers at New Hampshire’s Dartmouth College discussed building machines that could sense, reason and think like people — a concept known as ‘general AI’. But their plans were destined to remain in the land of science fiction for quite some time.
However, in the last decade the rapid growth in computer-processing power, the availability of large data sets and the development of advanced algorithms have driven major improvements in machine learning.
AI researcher Ben Goertzel brought to light ‘narrow AI’ in 2010. It focuses on complicated, but specific tasks with no genuine intelligence or self-awareness. These techniques have become ubiquitous and are widespread in our daily lives, examples being applications such as computer vision, voice analysis and even route selection in our mobile devices.
Turning the lens to healthcare, this technological progress has also triggered a surge of start-ups that are using artificial intelligence and machine learning technology for drug discovery and diagnosis, with many of them exploiting its massive potential to identify patterns hidden in large volumes of data.
Casting an eye across the AI healthcare landscape shows disease identification and diagnosis of illnesses to be the current forerunners of machine learning research in medicine.
Industry data shows that more than 1,100 medicines and vaccines to treat cancer alone are currently in trials. While this wealth of development could mean substantial positive change for cancer patient outcomes, it also presents the challenge of finding solutions to make the most of the large volumes of resulting data. That is where biologists and researchers need to closely cooperate with information scientists to explore the potential of enhancing insight, development and learning through AI and machine learning.
To give a sense of the potential this technology holds for human health, we take a look at four recent AI healthcare developments which are laying the foundations for the future of machine learning in medicine.
IBM examines cancer gene alternations
It’s no surprise that technology leaders were some of the first to jump on the bandwagon, particularly in high-need areas like cancer identification and treatment. IBM Watson Health launched a genomic sequencing service in partnership with Quest Diagnostics, which aims to make advances in precision medicine by integrating cognitive computing and genomic tumour sequencing to quickly uncover highly personalised cancer treatment, including options that previously may have remained unidentified.
As a core part of this project, US hospital Memorial Sloan Kettering (MSK) Cancer Center combined its patient data with Watson’s scientific information into
Paul Sabbatini, deputy physician-in-chief for clinical research at MSK Cancer Center, said: “We now know that genetic alterations are responsible for many cancers, but it remains challenging for most clinicians to deliver on the promise of precision medicine, since it requires specialized expertise and a time-consuming interpretation of massive amounts of data.”
“Through this collaboration, oncologists will have access to MSK’s expertly curated information about the effects and treatment implications of specific cancer gene alterations. This has the power to scale expertise and help improve patient care.”
The rocky road started with suggestions last year from Jefferies that IBM was being “outgunned in the war for AI talent and will likely see increasing competition”. The equity researchers reported the company may also not be able to drive a profit, despite its extensive healthcare investments.
Just a year later IBM has laid off a substantial amount of Watson’s Health workforce, with reports suggesting the June 2018 job cuts affected 50-70% of employees in that division.
A spokesperson for the tech giant said the layoffs were part of its restructuring plans and that IBM would continue to invest in high value segments of the healthcare industry like data management, analytics and AI.
However, more recently the efficacy of its oncology offering was dealt a blow, after a Stat report on internal IBM briefings showed the ‘supercomputer’ would often suggest unsafe or incorrect treatments.
Google looks deep into your eye
Another example of a tech-giant making advances into healthcare through AI is Google’s DeepMind Health, in this case, working in partnership with Moorfields Eye Hospital NHS Foundation Trust in London, developing technology to address macular degeneration in aging eyes.
The results, recently published in Nature Medicine’s journal, detail the process in which AI technology analysed thousands of historic retinal scans to identify signs of eye diseases — such as glaucoma, macular degeneration and diabetic retinopathy.
Dr Pearse Keane, consultant ophthalmologist at Moorfields Eye and National Institute for Health Research (NIHR) Clinician Scientist at the UCL Institute of Ophthalmology, said: “The AI technology we’re developing is designed to prioritise patients who need to be seen and treated urgently by a doctor or eye care professional.”
Currently, ophthalmologists around the world use optical coherence tomography (OCT) scans as part of the diagnosis process to detect signs of eye disease, but analysing complex images is time consuming and can slow down the speed of diagnosis.
“The number of eye scans we’re performing is growing at a pace much faster than human experts are able to interpret. There is a risk that this may cause delays in the diagnosis and treatment of sight-threatening diseases, which can be devastating for patients,” added Keane.
The artificial intelligence technology trialled in the London hospital was able to quickly identify ten features of eye disease from standard OCT scans. The system was then able to suggest the diagnosis, including its explanation and treatment recommendation to a doctor to review.
Google is going to take this technology to further human trials and is currently exploring the use of the same machine learning idea in other disease areas, including cancer treatment.
DeepMind Health came under fire in June 2018 for its data security. The Information Commissioner’s Office (ICO) in the UK investigated the NHS data sharing deal after a complaint from the public.
Mustafa Suleyman, co-founder and head of Applied AI at DeepMind Health said: “Given the sensitivity of health data, we’ve always believed that we should aim to be as innovative with governance as we are with the technology itself.”
“We’ve already invited additional oversight into DeepMind Health by appointing a panel of unpaid Independent Reviewers who are charged with scrutinising our healthcare work, commissioning audits, and publishing an annual report with their findings.”
The review conducted by the panel said the company must provide more transparency to gain public trust and recommended minor amendments to the information processing agreement signed with the NHS.
Berg researches automated therapies
Boston-based biopharma company Berg is using AI to research and develop diagnostics and therapeutic treatments in multiple areas, including oncology, by applying an algorithm and probability-based artificial intelligence to analyse large numbers of patients' genotype, phenotype and other characteristics.
The aim of Berg’s approach is to identify potential treatments on the basis of the precise biological causes of disease.
“We are turning the drug-discovery paradigm upside down by using patient-driven biology and data to derive more predictive hypotheses, rather than the traditional trial-and-error approach,” said Niven Narain, Berg’s co-founder and CEO.
Using this approach, Berg’s team identified the importance of certain naturally occurring molecules in alterations in cellular metabolism pathways. This led the group to discover how its own AI assisted cancer drug BPM31510 works and indicated some possible therapeutic uses.
The drug, BPM31510, is currently in a phase II clinical trial, having already received an orphan-drug designation in the US by The Food and Drug Administration (FDA) in February this year in people with advanced pancreatic cancer. The company is also using this AI system to look for drug targets and therapies for other conditions, including diabetes and Parkinson’s disease.
BenevolentAI’s system can suggest medication
London-based start-up firm BenevolentAI developed its own artificial intelligence platform, fed with data from research papers, patents, clinical trials and patient records.
This forms a representation of more than one billion known and inferred relationships between biological entities such as genes, symptoms, diseases, proteins, tissues, species and candidate drugs.
The database can be queried just like a search engine, to produce knowledge graphs of a medical condition and the genes that are associated with it, or the compounds that have been shown to affect it.
Most of the data that the platform is working with are not annotated, so it uses natural-language processing to recognise entities, understand their links to other records and easily extract information such as medical condition and the genes that are associated with it, or the compounds that have been shown to affect it.
“AI can put all this data in context and surface the most salient information for drug-discovery scientists,” says Jackie Hunter, chief executive of BenevolentBio.
In the article published in Nature the biotech described an experiment in which researchers asked the system to suggest new ways to treat amyotrophic lateral sclerosis. It returned around 100 existing potential compounds.
Scientists selected five to undergo tests using patient-derived cells. The research found that four of these compounds delivered promising results, and one was shown to delay neurological symptoms.
Bright future for machine learning
McKinsey estimates that big data and machine learning in pharma and medicine could generate up to $100bn annually, based on better decision-making, optimised innovation, improved efficiency of research, clinical trials, and new tool creation for all stakeholders.
Increasing AI investments in drug discovery by big pharma companies suggests the industry has not only woken up to but is actively embracing the benefits of machine learning to identify and screen drugs, more accurately predict drug candidates and cut R&D costs and time.
Pharmaceutical giant Merck & Co is working with Atomwise on a confidential project which uses deep learning technology for the discovery of novel small molecules. GSK entered a $33m drug discovery collaboration with AI-driven start-up Exscientia and US-based Cloud Pharmaceuticals; Pfizer has started a collaboration with IBM Watson for immuno-oncology drug discovery research and the list goes on.
Narain, co-founder and CEO of Berg thinks the technology will drive major advances: “Over the next five years or so, the truth will come out in the data,” he said. “If by then we are creating better drugs, and doing it faster and cheaper, then AI will really take off.”