Why intelligent automation is the future for regulatory
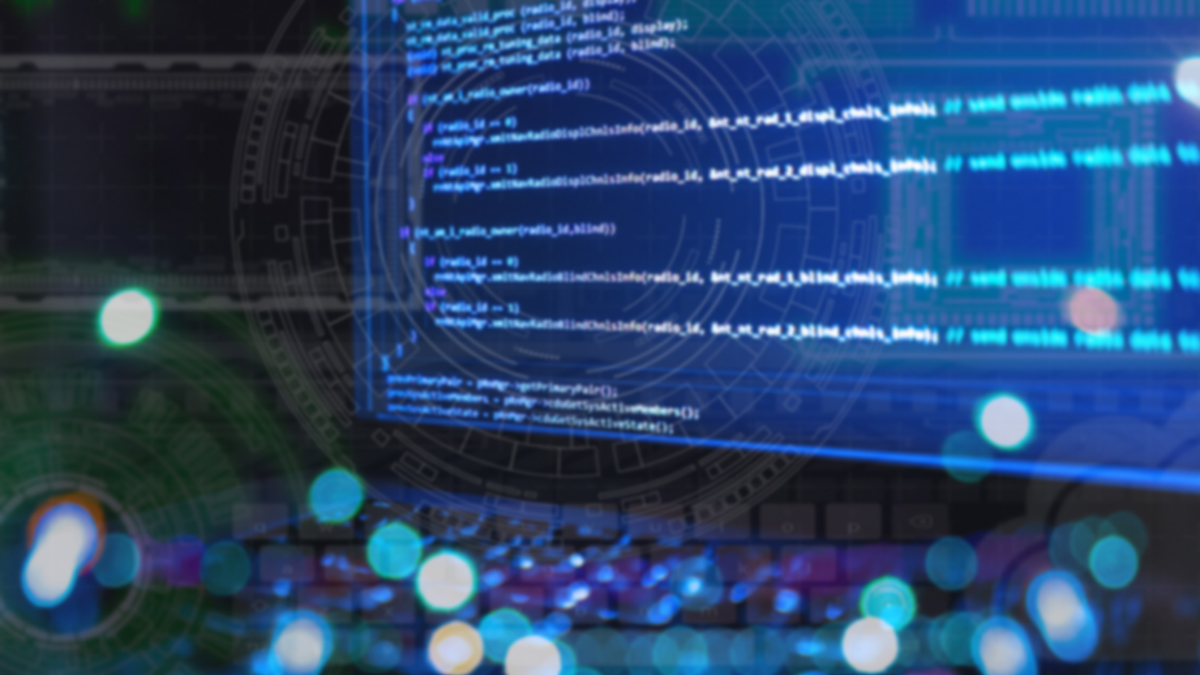
There are a host of new, previously unimaginable tools and techniques – from analytics to robotic process automation (RPA) and artificial intelligence (AI) – available to help speed up processes and increase data accuracy. But for many life sciences organisations, these tools are either not yet fully adopted or are not being put to good use within their regulatory functions.
In Accenture’s Intelligent Life Sciences: Redefining Regulatory through Intelligent Automation report, we underscore how automation can help regulatory professionals in life science organisations bring products to patients faster.
Adopting intelligent automation could help organisations realise disruptive benefits that are above and beyond the tangible gains of cost, quality and productivity improvements. By applying intelligent automation, companies could realistically expect to enable: the seamless distribution of product information in a variety of multimedia channels to all internal and external stakeholders; rapid and accelerated implementation of product advances that could propel continuous improvement; and advanced prediction of risks to mitigate against resource capacity constraints – which have been highlighted during COVID-19.
For many companies, it could be realistic to automate as much as half of the manual maintenance tasks currently performed, resulting in significantly different future operating models where blended roles will prevail with strategic product oversight.
But where to begin?
Where do you begin this transformation? Start with the data. Companies need to change the way people collect, curate, interpret and apply data for regulatory submissions and improve confidence in that data. According to Accenture research, most organisations are still struggling to understand the basic ‘truth’ of the data they use and exchange with others. The Accenture Technology Vision survey found just one-third of 103 life sciences executives have high confidence in their data and validate it extensively. To improve data confidence regulatory executives should take a four-step approach to change how they:
- Collect Data: Life sciences organisations should utilise cloud-based solutions with global access that facilitates one repository with a single source of truth and eliminates the use of local file sharing and servers. By integrating applications across the end-to-end value chain, they eradicate data entry duplication.
- Curate Data: Regulatory specialists need to apply data standardisation and master data management to define the right granular level of data for storage. This needs to be done up front. By implementing robust data governance and management, they can maintain data quality and integrity. By ensuring traceability of data evolution, as well as end-to-end transparency of submission status and its components, they help promote confidence in the data itself.
- Interpret Data: Executives need to make more use of readily available data to drive business decisions and optimise operations. They should be applying analytics to past submission data to recommend future submission content plans and pre-empt and mitigate health authority (HA) questions.
- Apply Data: Regulatory employees should be using stored data to intelligently create submission documents. By limiting documents full of free text fields and subjectivity companies can adopt a more digitised approach, where document templates can be compiled automatically from available data. Making real-time data accessible to the consumers of the information when and where it’s needed increases the likelihood of buy-in to the system’s value-add. In this way, manufacturing scheduling can be optimised, and batch release decisions more informed. This, in turn, enables healthcare practitioners to get the most up-to-date product information at their fingertips.
Focusing on the business outcomes of how data can be interpreted and applied, through implementation of analytics, RPA and AI, will help strengthen your data vision, enhance your data stewardship culture and provide financial justification for investing in improved data management. This is crucial because all signs point to a future world order of increasing volumes and complexity of new products coming to market, and digital support is a must have – not a nice to have – to handle this workload.
What intelligent automation to consider?
As companies make the move into the realms of intelligent automation, there are 3 key considerations that need to be considered from the offset.
- Set the North Star vision: Discuss the company strategy and pipeline considerations for the next 3-5 years and what the business objectives to achieve this strategy are. Is the driver for intelligent automation waste reduction and cost saving, or the speed at which innovative products are being brought to patients? Define the priority focus areas for your intelligent automation roadmap and evaluate the balance of ‘quick wins’ versus longer term strategy initiatives.
- Create the Operational Blueprint: Outline what the future processes look like with the inclusion of intelligent automation. What activities remain and from where should they be performed? Consider how job descriptions and roles will evolve to account for the transformed future working environment.
- Prototype, automate, evaluate, repeat: Establish the infrastructure to prototype at speed and fail fast. Consider how to implement in an agile, modular manner that gradually combines into an end-to-end solution. Carefully evaluate benefits realised.
Here are some example use cases showing how Intelligent Automation is changing the game:
Regulatory Requirements & Content Plans
- Business Challenge: Maintaining data on submission requirements is a constant challenge. As a result, market requirements gathering is often repeated for each submission, generating longer lead-times. Additionally, insights gained from HA feedback are not incorporated into submissions, reducing first time submission approval accuracy.
- Solution: Analytics can compare new submission properties against past submissions to suggest a content plan based on the closest match, and most recent, approved submission.
Health Authority Correspondence Processing
- Business Challenge: Timely recording of submission approval dates or tracking of HA questions can be challenging when information received by affiliates needs deciphering and translating before being entered into regulatory systems.
- Solution: AI tools can translate and decipher letters without the need for local affiliate intervention and automatically enter information in Regulatory Information Management systems for stakeholders to act upon.
Label Authoring and Tracking
- Business Challenge: Managing and providing traceability of the roll out of global label updates is onerous based on language nuances, implementation considerations and replicated data terms across multiple documents which can lead to a high risk of product label inconsistencies.
- Solution: AI tools can take the complexity out of mapping global-to-local terms and automatically suggest what updates are needed where, as well as provide end-to-end traceability of the update progress.
As companies make the move to adopt intelligent automation, they need to make sure their projects are: business-outcome oriented (rather than simply automating a task or function); human-centred (so they don’t just eliminate repetitive tasks but rather free up people to focus on higher-value analysis, decision making and innovation); and technology rich (i.e. integrated into the broader architecture of data sources and applications).
How do you ensure success?
And there are pitfalls to avoid. One of the most common mistakes companies (across all industries) make is to allow business units to carry automation initiatives in silos. You should drive home the notion of ‘one company’ and keep an eagle-eye out for cowboys building their own rogue programmes. Similarly, make sure everyone is on the same page – if there is a disconnect between IT and the business, what’s built may not serve what’s needed. And make sure your HR, training and communications teams are looped in, informed and armed with the right information and tools to encourage and support adoption.
Lack of communication on how the workforce will be re-purposed post automation implementation can lead to internal unrest and possible attrition. There will need to be training and change control in place, and that takes planning and management. Finally, from the onset and throughout there needs to be a team tasked with ensuring responsible automation is in place. This needs to be owned by the C-Suite and at every turn communicated to employees, so it is part of the DNA of the transformation: consider the ethical and legal implications when data is handled, and tasks are automated. Know who is accountable and responsible for outcomes and ensure those teams own that responsibility.
The process of adopting intelligent automation isn’t easy but it’s also unavoidable. Adoption of processes and procedures to handle complex data is essential in this day and age – the companies that fail to build a platform that is adaptable to change are at risk of being left behind; those that do adopt new solutions are not only poised to succeed – they are the industry leaders of the future.
About the author
Kim Brownrigg is a senior principal at Accenture and leads the Regulatory Domain in Europe. Her responsibilities include interlinking Consulting, Technology and Operations services to optimise client value and defining next generation service offerings. Kim is currently leading Accenture’s Regulatory digital transformation programme; helping clients define their digital strategy and roadmap within Regulatory, designing applied intelligence solutions and prototypes and delivering pilots and scaled implementation to transform and streamline the industry.