Harnessing the digital transformation of the drug development pathway
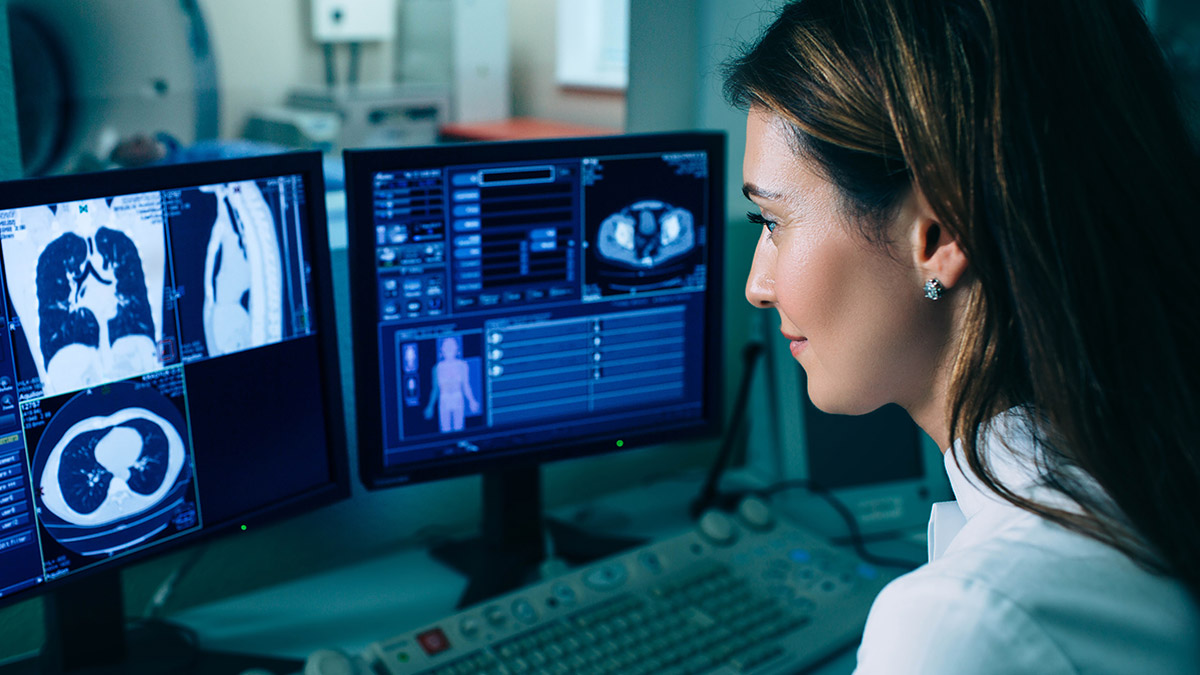
We have the evidence base to support the digital transformation of clinical trials – what we need now is the courage and the regulatory framework.
From virtual monitoring and eConsent to AI-driven image analysis, technology is revolutionising the drug development pathway.
And now that the evidence base is catching up with digital capabilities, drug developers and manufacturers are keen to embrace the opportunity to use emerging innovations to design more efficient, more patient-centred studies.
Kaisa Helminen, CEO at Finland-based Aiforia Technologies, says: “I don’t see the implementation of digitisation being a barrier anymore, because it’s pretty widely used, so I think it’s more about people having the courage to try new technologies.
“In terms of barriers, I think they are now mainly regulatory...I think that’s a part of what has been hindering or slowing the process of adoption”
“The scientific community will often have doubts about using novel technologies and solutions before they have been proven and we have seen the results. But we have that body of evidence now, in scientific journals and through peer-reviewed papers.”
Emergence of regulatory frameworks
The benefits of AI-driven image analysis in drug development are now “pretty obvious”, she says, and regulatory frameworks are starting to catch up with that realisation.
For example, the Food and Drug Administration (FDA) has already approved a number of AI models in radiology applications.
“Things are definitely moving forward,” says Helminen. “There’s also a lot of movement in monitoring patients during the trial and acquiring real world data from patients. That’s very digital today and there are a lot of solutions out there.”
Ongoing regulatory barriers
In Aiforia’s “relatively new” but rapidly growing field, that of digitalised pathological image analysis, there’s still some way to go in terms of regulatory approval.
This is reflected in the fact that the AI-supported digital image analysis technologies have been first implemented in the research pre-clinical development phase.
“It’s extremely useful in the pre-clinical phase, when drug candidates are tested in animal models and there is a huge need for accurate, quantitative information, as well as reproducibility and speed of analysis, from enormous quantities of image data,” says Helminen, whose company provides AI- and deep learning-driven image analysis tools.
“This way we can significantly speed up the testing processes and enable acquisition of novel types of information from image data, to understand better the disease mechanisms, for example.”
It seems natural, then, that this technology is being used in the preclinical stage of drug development, speeding up the process of candidate selection.
But Helminen believes the method holds just as much potential in clinical trials, particularly multicentre studies where the consistent evaluation of patients’ sample images may be difficult to achieve.
“We know that you are really limited with the information you can get from the manual, visual analysis of histology samples, for example. If you are using different centres with different people who might have differing opinions or standards to score, it’s not reproducible. How can you compare apples to oranges?
“By digitalising the images and using automated analysis tools, you get information that is reproducible, quantitative and fast.”
Clinical trials are expensive, complex to organise and hard to recruit for, meaning it’s imperative to get as much information as possible from each valuable tissue sample, she adds.
Asked why the technology hadn’t yet broken through into clinical trials, Helminen says its recent emergence on the scene was only part of the reason.
“In terms of barriers, I think they are now mainly regulatory.
“The pre-clinical phase is not as tightly controlled as studies within humans. When we are talking about studies on animal models, there is less burden on the organisations to approve various new technologies.
“But when we start talking about patient sample analysis, the regulatory question comes into the picture. I think that’s a part of what has been hindering or slowing the process of adoption.”
Joining the data dots
Regulators are keeping up with the pace of innovation in this space, as seen by the FDA’s approval of several AI-based radiology models.
This, Helminen hopes, will “open the aisle” for digital pathology, which she sees as a crucial piece in the medical data jigsaw.
“I think that understanding images better is key to making novel findings from image data. There are many hidden things in images that we have either not been able to see or not been able to understand,” she says, adding that computational pathology had the potential to change this.
At the moment, it’s a matter of one technological step at a time. Yet the possibilities arising from being able to combine the sophisticated datasets currently in development are potentially transformative.
“By looking at things like genomics data, morphological data, medication information and all different kinds of data that are being collected directly from patients, we will be able to understand diseases better and make new medications that reflect that.”