5 pitfalls AI healthcare start-ups need to avoid
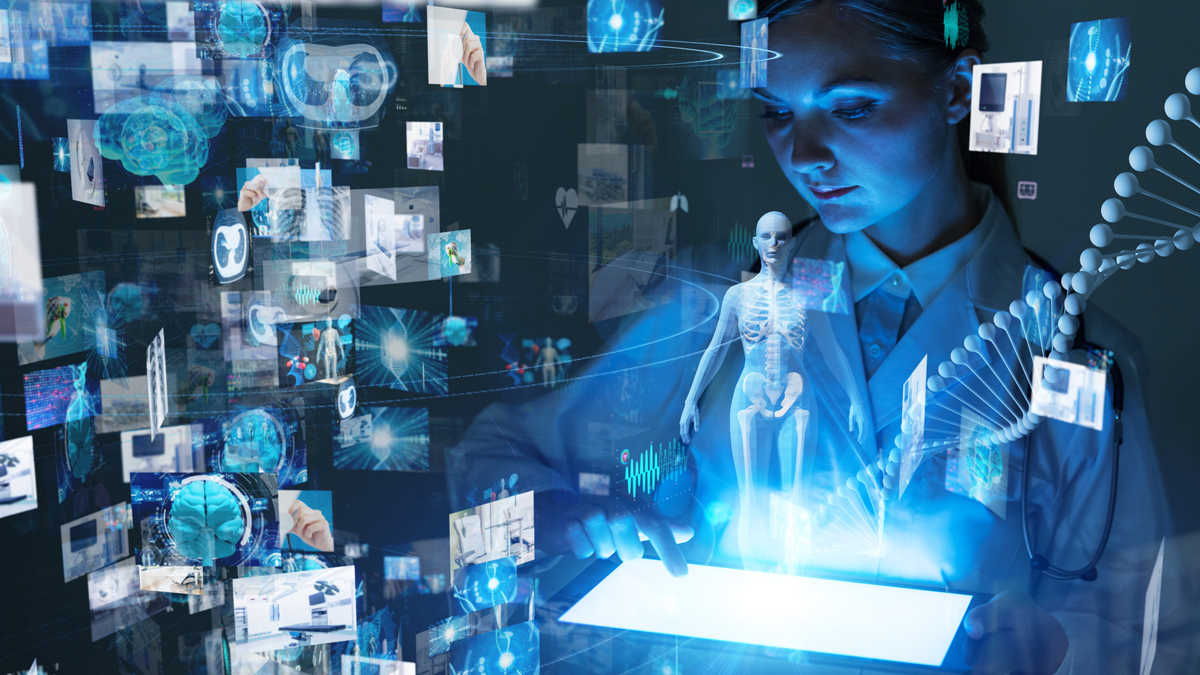
Artificial intelligence (AI) has now moved beyond its initial hype towards becoming a key part of the pharma industry – with many companies looking to partner with AI drug discovery start-ups.
Pharma and healthcare are data-rich industries and AI helps by turning data into actionable insights, allowing us to solve complex, intricate problems. Using machine learning, AI algorithms can generate patterns that will enable us to predict toxicity, find potential combination treatments, identify and predict new drugs and expand usage of current drugs in other diseases.
However, only a handful of companies from the swarm of AI start-ups have successfully gained traction within the pharma industry.
Over the past month, I’ve had conversations with several growing AI drug discovery companies and have analysed some critical strategic shortcomings that can frustrate the upwards journey of these start-ups:
1) Living in a technology bubble and failing to understand the language of pharma
AI cannot be built in isolation without understanding the nuances and the complexity of the business needs it will address. A constant challenge faced by all AI drug discovery companies is ‘sanitisation’ of the unstructured but useful data in life sciences.
Drug discovery is inherently a high-risk endeavour due to poorly understood mechanisms of diseases and lack of negative data (on experiments that do not work) in the public domain.
With high reproducibility issues in life sciences, nuances such as the validation of experimental data, complex networks of interacting proteins, poor quality publications etc., add another layer of difficulty.
Companies will spend much of their time filtering the essential insights from a pile of useless data.
Diversifying the team and having pharma executives on-board – who can understand the relevance of a dataset and ontologies created by the platform – is critical to success.
Because of a lack of thorough understanding, start-ups often fail to attract investors.
The AI drug discovery ecosystem has been only partially successful in addressing this challenge till now. AI depends critically on the quality and standardisation of data used. ‘Garbage in, garbage out’ holds true for AI.
2) Prioritising technology over business strategy
AI alone is not enough to succeed. A start-up at the interface of technology and pharma needs a solid business strategy to thrive.
Often these start-ups focus on how powerful and transformative their platform is and lack an overall business workflow. Most start-ups struggle to find the right positioning in the market – whether that’s providing data analysis as a service, licensing the AI platform, acting as a consultant or developing a new drug/repurposing drugs either alone or in partnership.
Only a handful of these start-ups have internal discovery programmes, while the rest of the companies struggle to find the right business model, often taking a mix of several approaches.
Narrowing down the business strategy while understanding the core strengths early in the journey can address challenges of efficient resource allocation. Likewise, identifying target markets and an effective sales and marketing approach can help clearly define success criteria with defined milestones in the journey.
3) Get stuck in a never ending development loop
AI is very expensive to build and maintain, owing to the need for big data and computational infrastructure. The scarcity of data scientists with domain knowledge in pharma and the large overheads required to hire them adds to the cost.
Many start-ups keep working on improving prediction scores with data and ultimately end up realising that it only adds marginal value for the customer. The benefits of moving to market quickly often outweigh the downsides – with early entry, companies can get immediate and frequent feedback from the end-users of the platform, and they can work with academic and industry end-users early in the journey to validate the platforms and generate the required proof-of-concept.
Rather than staying in a continuous development loop, taking one application to market while you build the rest is a stronger approach.
4) Assuming customers are technologically savvy
Developers should keep the end user in mind when designing the UI/UX of the platform – while still addressing the key technical components.
End users in pharma are often biologists, with minimal exposure to developer tools. While some computational biologists write scripts, most drug discovery scientists are habituated to one-click software. Moreover, there are certain standard ways of data plotting that scientists are habituated to, owing to requirements in international peer reviewed journals.
An easy, graphical results sheet can communicate information better than the strings and scores preferred by software developers.
5) Taking a ‘do-it-all’ approach over finding a niche
Start-ups often get starry eyed about the capabilities of their platforms and want to do everything.
The majority of these algorithms can be repurposed for diverse functions depending on the training data and required outcomes. As the Russian proverb goes, “If you chase two rabbits, you will not catch either one”.
Building new functions requires resources and attention to detail. Start-ups should begin by pioneering one functionality and then building in other directions. Prioritise offerings based on core strengths, get client feedback and revenue flowing and then continue building on other applications.
While a solid foundation has been laid, the AI community will have to be patient and not interpret pharma’s conservatism as a lack of interest. They need to engage continuously with the biopharma leaders and regulatory bodies to build a multi-dimensional strategy involving innovation, privacy, compliance, standardisation, and behavioural change management.
About the author
Amandeep Singh is a life science consultant at MP Advisors.