Putting a critical eye on early clinical trial design decisions
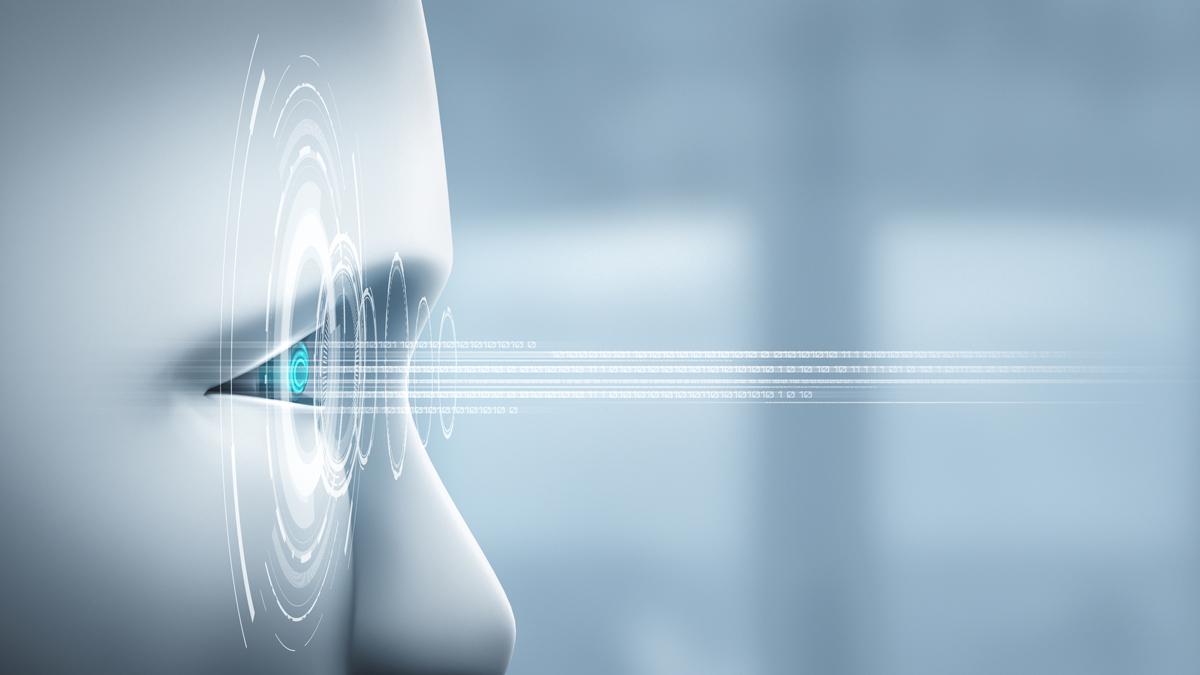
Drug development industry stakeholders recognise there is no one-size-fits-all approach to the long-standing challenge of patient recruitment and engagement. Ensuring you are reaching the right patient populations and engaging them effectively must be addressed from multiple angles and solutions, especially in today’s complex research and development landscape.
Because trials and target patient populations vary in so many ways, clinical trial sponsors see the value in proactively identifying ways to enhance protocols early in study design to reduce amendments and related hiccups and make patient participation better. Though they understand the value, it may be difficult for sponsors to map out approaches that will maximise their efforts.
Whether it is thorough evaluations of the market, relevant treatment patterns, patient perspectives, or other issues, there is a transformative shift in how tech-enabled solutions like artificial intelligence and machine learning modelling and advanced data analytics can help leverage an extensive breadth of available data to guide more informed decisions about trial protocols. As a detailed example, we take a deeper dive into how strategic, evidence-based study design, with patient centricity top of mind, can make a positive impact on actionable patient recruitment and engagement downstream.
Variables impacting today’s protocol design
We know there are several key factors that impact the effectiveness of a trial’s protocol in today’s R&D environment.
For one, with growing scientific discoveries and a push toward personalised medicines, trials are becoming increasingly complex, which may negatively affect protocol amendments and patient recruitment and dropout rates. From 2005 to 2020, the number of required study procedures increased by 139% in Phase III trials, with the number of endpoints increasing by 214%. Also, though there are multiple data sources to access for study design, the data may be fragmented. It is difficult to know what evidence is available and what may still be needed with siloed sources, which leads to gaps in information.
Knowing these factors, how can sponsors successfully address trial complexities and better connect multiple data sources to provide useful insights to direct trial design and related patient recruitment efforts?
Minimising design unknowns via data iterations
Creating an ecosystem of data resources (e.g., disease communities, real-world data, regulators, etc.) and applying analytics early in study design can support the creation of viable design elements like endpoints, eligibility criteria, or treatment comparators.
“What if” scenarios
When leveraging advanced analytics, real-world data, and the right mix of expertise (e.g., design analytics experts, AI/ML engineers, clinical trial experts, patient recruitment specialists, etc.), “what if” scenario generation can help sponsors review different eligibility criteria and parameters to determine what may best help meet recruitment goals and quantify the impact of each option. For example, in a type 2 diabetes study for which the sponsor wants to increase the patient pool, analytical modelling leveraging real-world data can create several scenarios for what likely variables will help meet that goal and to what degree. Analysis may show that decreasing the lower limit of the required HbA1c range even by 0.5% and allowing metformin as a concomitant therapy can double the percentage of eligible patients. Variations of that modelling can help sponsors determine the ideal criteria to maximise enrolment potential.
Real-world treatment patterns
Evaluating real-world treatment patterns can help inform decisions for treatment comparators and identify available patients within a defined population. In some cases, analytics can help quantify how these patterns vary from industry guidelines. When reviewing real-world data for an oncology trial, understanding the treatment path an actual patient population takes versus what is published as recommended care in industry guidelines can be worthwhile. If the sponsor wants to widen the patient pool, analytics can help highlight exclusion insights by specifics. For example, if an oncology study requires participants to have received and progressed on a specific immunotherapy, prescription and disease data will indicate the number of patients treated with that therapy as a first-line treatment. If that number is low, it can significantly reduce the number of eligible patients to consider, so sponsors may want to add other immunotherapies to their inclusion criteria to expand the patient pool.
Market landscape view
Drug developers will always need to keep a keen eye on their market, but how far down into the data can they go to direct their individual trial design efforts? Using applied analytics, it is possible to gauge trial feasibility in specific ways. Identifying sites that treat patients within specific indications of interest and how they are delivering that treatment can be helpful to understand potential patient populations for those locations.
Additionally, by analysing all available historical data, stakeholders can examine previous endpoint performance to determine potential primary and/or secondary endpoints to consider. For example, for a diabetic peripheral neuropathy trial protocol, the sponsor wanted the primary endpoint to be numbness and tingling. However, cross referencing with historical trial data may show that previous trials conducted by other companies alongside regulatory guidelines may have pain as the primary endpoint. However, in layering in recent literature, there may be a case for numbness and tingling. Though it will take further evaluation given the findings, having the ability to leverage this level of data via multiple resources will help stakeholders make more informed decisions earlier in the process.
The key benefit of the strategic analytical applications discussed above is the opportunity to secure results to gain initial insights early in the trial design process. These insights can be further iterated upon to support evidence-driven decision-making, thereby offering a chance to see how the layers of changes may impact protocols.
Study design through the eyes of the patient
By moving towards developing drugs with patients, not for them, the industry is showing that it collectively views patients as partners in the trial design process. From understanding nuances in patient burdens to analysing their real-world experiences, sponsors and clinical research organisations are committed to integrating their voice into trial design. According to research findings from the Tufts Center for the Study of Drug Development, engaging patients in protocol design improves clinical outcomes, including reducing substantial protocol deviations and needs for amendments, which enhances trial timelines and operational efficiencies, and ultimately helps speed up development for patients in need.
Sponsors can better pinpoint patients’ pain points, preferences, and experiences as early as possible in the design development process by applying data science and analytics methodologies. Through a host of data sources (e.g., real-world data, customised patient surveys, scientific literature, social listening findings, insights from patient advocacy groups, focus groups, and providers, etc.), sponsors can secure customised patient-focused data assessments to further explore nuances in design.
Within a matter of weeks or a few months, design analytics applications alongside patient market research, recruitment insights, and more can help answer specific questions about the trial at hand, such as:
- How do this trial’s design elements compare in terms of patient burden to studies with similar phases and therapeutic area?
- Which design elements will resonate with target patients?
- What does the typical patient look like (e.g., behavioural patterns, skills, goals, etc.)?
- Which clinical outcomes assessments are used to support label claims for this indication, and which COAs have been used by similar trials recently?
- Is it possible (or necessary) to integrate decentralised trial solutions?
- What is needed to optimise the patient-reported outcomes/COA strategy?
- What tailored materials and messaging will resonate best with target patients and their families?
Further, it is possible to map scenarios with advanced analytics of patient insights and related data to understand and better address potential patient burdens. For example, sponsors can compare trial burden scores based on design elements at different rates as seen in this chart. Gauging scenarios can indicate preferred length and/or frequency of visits. Having these discussions early in planning can also help to avoid costly pivoting later in development.
Driving actionable recruitment through modelling
The practice of iterated evidence-driven analysis early in trial design would be pointless if there was no actionable follow-through to leverage these insights within the patient recruitment and engagement strategy and related marketing outreach.
AI-based modelling can evaluate inclusion/exclusion criteria to help narrow potential trial participants, sifting through millions of data points (e.g., claims data, pharmacy data, etc.) to identify those who may meet protocol requirements. With a core list of deidentified individuals, sponsors and CRO partners can tap into a larger consumer database of demographics, lifestyle variables, purchasing habits, and other useful insights to understand areas of commonality. Refined insights can be as specific as:
- Residing in a household with or without children or an elderly parent.
- Purchasing habits, such as ordering products via mail.
- Level of access to internet.
- Hobbies, such as boating, hunting, fishing, etc.
- Music or TV preferences.
- Household philanthropy or lack thereof.
By layering these data insights, the modelling can also rank patients from most likely to least likely to meet protocol requirements. Breaking down insights from the modelling further and further can help create an intersection between the points that matter most to the sponsor, such as matching protocol requirements to patients’ geographical locations and willingness to participate in trials.
Additionally, and as importantly, sponsors and CROs committed to improving inclusion in clinical trials, especially from those most affected by the disease, can proactively overlay diversity data on top of this modelling to reach patients from traditionally underserved communities.
Taking these steps early in trial design helps shape the strategy for tailored recruitment and engagement. Sponsors can create a multi-pronged marketing approach, including customising advertising content according to what will resonate with individual audiences of interest, what communication platforms may work best, what imagery to use, etc.
Informed decisions through the protocol development continuum
Unlocking the potential of accessible data from multiple sources to guide informed trial design decisions is possible through thoughtful planning and use of strategic analytic methodologies. Deep dives into data insights and increased patient engagement in the protocol design process allows sponsors to apply that collective knowledge to the bigger picture and inform better decisions to steer trials in the right direction. In reducing protocol deviations and need for amendments, these advanced data-driven approaches are being shown to improve clinical outcomes and accelerate R&D efforts in a complicated landscape.
About the authors
Gail Long is director and head of marketing patient recruitment and enablement at IQVIA. She brings more than 10 years of experience in patient recruitment and three decades of marketing experience to her current role, in which she responsible for connecting potential patients to clinical trials and ensuring her team effectively incorporates their needs into all aspects of marketing outreach.
In her current role as director of clinical planning and design analytics at IQVIA, Tammie Nguyen develops data-driven analytics packages for clinical trial sponsors. A pharmacist by training, with a background in biochemistry and cell biology, Nguyen has more than a decade of clinical drug development experience. She has pressure-tested over 150 protocol assessments using historical and real-world data to identify areas for protocol optimisation prior to execution.
Working at IQVIA since 2015, Ivan Petrov – associate director of clinical planning and design analytics – helps clinical trial sponsors optimise their study design with transformative design analytics. In his work, Petrov is focused on the patient perspective, ensuring the patient voice is heard and integrated into end-to-end clinical development. Prior to joining IQVIA, he was a part of the Nielsen consulting team, leading the business for Bulgaria and advising fast-moving consumer goods companies by creating tailor-made solutions to meet client goals.
Jane Rheaume, associate director of marketing at IQVIA, has specialised in clinical trial patient recruitment for more than eight years, with an additional three decades of direct marketing experience. In her current role at IQVIA, she ensures clinical trial-related marketing is strategically prepared at study start-up and manages traditional channel outreach to match patients to appropriate clinical trials.