Patentability and predictability in AI-assisted drug discovery
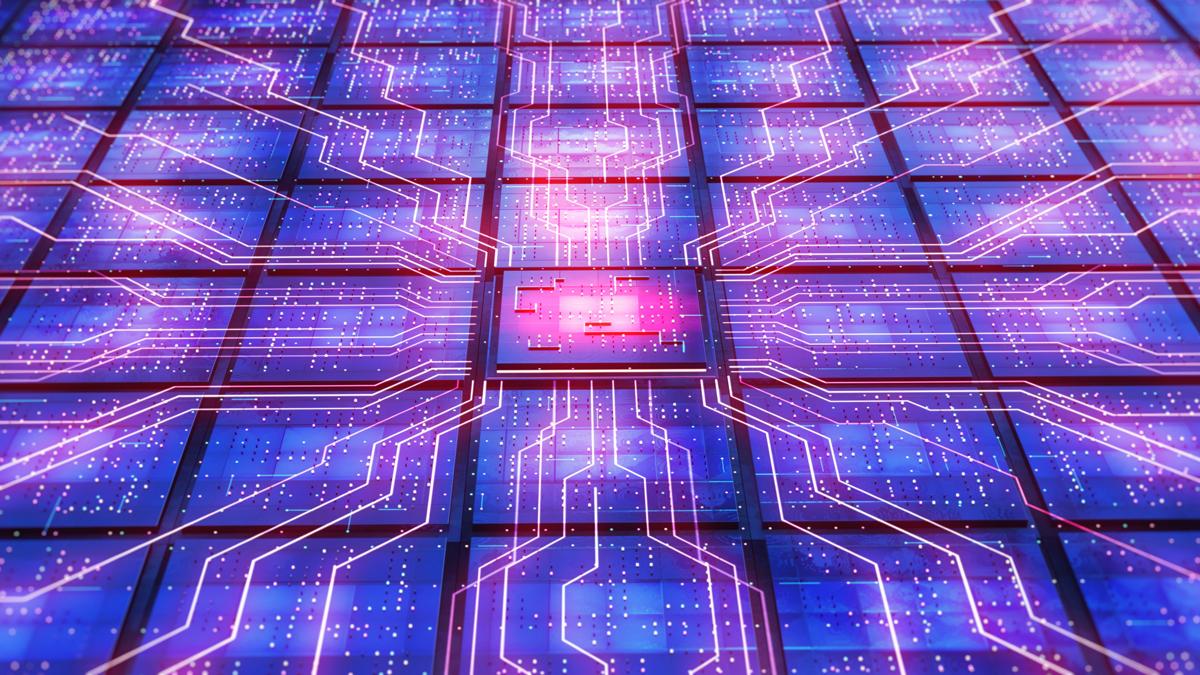
Artificial intelligence (AI) and machine learning (ML) are ushering in a new era in drug discovery.
The AlphaFold2 system developed by Google’s Deepmind was able to predict the 3D structures of over 200 million proteins - with accuracy on par with experimental methods - in just two years. AI tools are being combined into virtual screening platforms that can accelerate multiple steps in the drug discovery process. As pharmaceutical companies focus increasingly on the development of biological molecules for tomorrow’s treatments, these significant advancements in modelling are poised to influence not only how companies develop their therapies, but also how intellectual property protects those innovations.
This article focuses on the evolving state of the art employing sophisticated AI tools and the implications for intellectual property protection covering cutting edge innovations in the life sciences.
Hot off the presses: Patent Office proclaims that AI-assisted inventions may be patentable
The Patent Office determined that AI cannot be an inventor. The Copyright Office determined that AI cannot be an author. So, one of the biggest questions surrounding human-conceived, but AI-assisted invention is whether the results can be patented. On 12th February 2024, the Patent Office began to answer that question, when it released its “Inventorship Guidance for AI-assisted Inventions”. The Guidance explained that using an AI tool, by itself, does not make an invention unpatentable. But that is not the end of the inquiry.
Inventions require “significant contribution” by a human - not simply the rote implementation of an AI-enabled discovery. The Guidance provides several examples that focus on the human’s role in the inventive process. Such roles that might support patentability include prompting a generative model to elicit a particular solution to a problem, training an AI tool to answer a question, or designing an experiment based on an AI output. On the other hand, merely owning or overseeing an AI tool is not enough to qualify as an inventor. The inventorship inquiry is fact-specific and will be decided on a case-by-case basis to determine the presence of “significant” human contribution.
There is more to come in short order. President Biden’s Executive Order on the Safe, Secure, and Trustworthy Development and Use of Artificial Intelligence (the “AI EO”) directed the Patent Office to publish additional guidance on the patent eligibility of AI innovations by 27th July 2024.
The current AI drug development “toolkit”
For ease of discussion, most of today’s common machine learning and AI can be divided broadly into two categories: Predictive AI (“PredAI”) and Generative AI (“GenAI”). PredAI encompasses traditional machine learning and similar tools that use data to create models to help understand patterns and trends. GenAI, on the other hand, learns from the data it is trained on and can generate examples that are probabilistically similar to that training data.
AI tools have become more widespread and available over the past several years, moving from pure research to turnkey solutions. For example, NVIDIA’s BioNemo platform combines several models into a ready-to-use toolkit that can be customised with additional training and fine-tuning. Toolkits like these include models for protein sequence generation, protein folding, molecule generation, and docking, along with models that can help infer effects and optimisations. These platforms are still growing as new models are developed and validated. In the drug discovery context, GenAI is poised to speed up candidate discovery - allowing researchers to generate drug candidates based on desired properties - while PredAI tools can be used to simulate and to optimise those drug candidates.
Workflows incorporating multiple AI tools have led to significant discoveries and clinical trials for drug candidates, including a new class of antibiotics effective against MRSA and VRE. To identify this class of compounds, researchers used multiple PredAI models to work systematically through a library of 12 million compounds. These models first predicted antibiotic activity, then human cell toxicity, and ultimately produced a few thousand hits. The team then further narrowed to include only novel structures to arrive at a handful of compounds for testing. That testing yielded effective compounds that share a structural class. Beyond identifying the compounds, the model provided researchers with explainable results and useful information to help understand the structural class and areas of interest for optimisation. The use of AI tools to enhance drug discovery and predictability has the potential to enhance patent protection covering the resulting inventions.
Patents and the “unpredictable arts”
The ability to protect innovation in the life sciences has been shaped in large part by two related patent law doctrines - the “enablement” and “written description” requirements. Both requirements are analysed from the standpoint of a hypothetical person “having ordinary skill in the art” - the so-called “skilled artisan”. While there is no precise formula for defining the “skilled artisan”, the idea is that patent documents should be assessed from the standpoint of an individual knowledgeable in the field of science pertinent to the invention.
The enablement requirement ensures that the patent document includes sufficient instructions to permit, i.e., “enable”, the skilled artisan to make and use that invention. The threshold for satisfying the enablement requirement is whether a skilled artisan can make and use the invention without “undue experimentation”. A “reasonable amount of experimentation” is permissible, but what is “undue” will depend on the nature of the invention and underlying science. The inquiry draws from questions, such as the number of experiments, the duration of time, the predictability of the science, and the nature of the guidance and examples in the patent description.
The closely related written description requirement obligates inventors to disclose an invention in sufficient detail to demonstrate that they invented what they claimed. Here again, there is not a precise formula - a patent is adequately described when it reasonably conveys to one skilled in the art that the inventor possessed the claimed invention at the time of the patent application filing.
Central to many life sciences patents is the so-called “genus” claim, which covers a group of related “species.” A patent may describe representative examples (“species”) or common features to describe a broader group (a “genus”). The examples must be enough for a person skilled in the art to visualise or to recognise the members of the entire group. While there is no magic number of necessary examples that satisfies written description or enablement, it is a balance of factors, like the maturity of the field and the predictability of the relevant feature.
Patent law jurisprudence over the past 15–20 years reflects that these requirements are more-closely analysed in inventions based upon chemistry and biology, which courts regard as the “unpredictable arts.”
Enablement: Can Generative AI tools convert “undue” experimentation into “reasonable” experimentation?
Given the improvement in, for example, predicting protein structures provided by AI models, has yesterday’s undue experimentation become today’s reasonable experimentation?
The availability of AI toolkits and uptake throughout the industry suggests that today’s “ordinary skill in the art” either includes the use of AI tools or will very soon. This may already be the case for small molecules. Chemists have at their fingertips today, AI toolkits that approach experimental accuracy. These tools have already produced drug candidates that have advanced to clinical trials.
The current tools for antibody-based drugs and other biologics are not as mature - perhaps reflecting the more complex nature of such drug development. That said, the potential of AI tools to predict interactions and to identify candidates seems promising. For example, there are developing models that predict antibody-antigen interactions based on sequence information. In principle, such models may be able to predict antibody-based drugs with particular functional features - a capability that may be the difference between a sufficient and insufficient patent disclosure based on current case law. These sorts of predictive tools may reduce the need for extensive screening required by the traditional wet-lab methods in favour of targeted candidate generation followed by in silico (i.e., experimentation performed by computer) screening. From a legal perspective, this technology may facilitate broader patent coverage by converting what otherwise would have been “undue experimentation” to something more predictable.
Despite such promising advancement, AI-assisted drug development is not without challenges. These AI tools are quite new, and questions remain about the predictive accuracy and the resulting drug candidate quality. Models are only as good as the data they are trained on - those developed using insufficient or poor-quality data might drive inaccuracy. Even for models with proven accuracy, it remains to be seen how much effort those models actually save in the lab.
There is a legal nuance that should be pointed out, which is that questions of enablement are answered based on the “skill in the art” at the time of the invention. So, the development of new AI-assisted tools cannot “save” existing patent disclosures that otherwise may not be sufficient. Similarly, better AI tomorrow cannot be used to argue that a patent filed today would not require undue experimentation.
Written description: Can inventors use AI tools to claim more broadly from less disclosure?
As discussed above, written description requires that a patent informs a person skilled in the art to recognise that the inventor invented what was claimed. The level of detail required to comply with the written description requirement in an AI-assisted world is unclear - but, like enablement, appears on the brink of change. Available AI toolkits should make it easier for skilled artisans to derive more from less, as it relates to patent disclosures. In more legal terms, the AI-equipped skilled artisan may be able to visualise or to recognise the members of the broader genus from a smaller given subset of species.
For drug candidates, this may strengthen patents through greater predictability. For example, with a PredAI that can model antibody-antigen interactions based on functional information, e.g., where it binds, so that a skilled artisan is able to recognise the relationship between members of the larger group. Or AI tools could lessen the number of examples that need to be disclosed in a patent, allowing a company to move faster on obtaining protection. Consider a scenario where a GenAI can reliably create similar small molecules based solely on how the examples dock with a target protein - other members of the class could be envisioned reliably from only a handful of specific examples.
Ultimately, these tools change the state of the art and will impact how the law is applied, even though questions remain about the extent of that impact.
Trade secrets may fill gaps in patent protection
It is important to note that not all matters are appropriate for patenting - regardless of the presence of AI tools. Trade secrets may be a business’ “secret sauce” and cover things like customer lists, custom algorithms, the New York Times’s methodology for its Best-Seller List, and the Coca-Cola recipe. Protectable trade secrets generally include information that is valuable because it’s kept secret from others.
At some point in the innovation process, a company must decide whether its proprietary work is more appropriately protected as a trade secret, and therefore kept private, or with a patent, and thereafter recited in the public domain. With uncertainty in the ability to patent AI-enabled innovation, companies may devote more attention to the viability of trade secrets.
In AI-assisted drug development, trade secrets may cover many aspects throughout the development cycle. But, early on, innovators must decide whether to file a patent application. The decision to file a patent will often require the disclosure of this proprietary information and will therefore extinguish the trade secret right. As described above, the “enablement” and “written description” requirements ensure the fulsome disclosure of patent applicants to provide the public with sufficient information to justify the government grant of a limited monopoly.
For example, consider an AI model providing explainable rationales for its candidate generation or optimisation targets - i.e., why the model is making decisions. This information is valuable to further research and development - assuming that the AI is correct. On the patent path, it may be necessary to reveal that valuable information in a patent application. Given the context-sensitive multi-factor analyses discussed above, the choice between trade secret and patent protection requires careful consideration based on the invention, its value, and the state of the art.
Conclusion
The use of AI is already changing how drugs are discovered and developed. The legal system has yet to catch up with the implications. With expected additional guidance from the Patent Office and continued AI-enhanced drug development, these issues will come to a head in the near future. Even though many of these questions do not have firm answers, their consideration can lead to a more-informed process for life sciences companies to develop and to safeguard their valuable innovations and intellectual property.
About the authors
Michael Kahn is a partner in Akin's IP practice in New York and focuses on intellectual property litigation in district courts nationwide. He represents innovative companies in various sectors and advises investment professionals on IP‑related matters.
Ryan Dowell is a counsel in Akin’s IP practice in New York and represents clients in IP litigation in federal district court and Section 337 Investigations at the ITC. Dowell’s practice spans technological fields ranging from medical devices to AI and facial recognition.
Svetlana Pavlovic is a counsel in Akin’s IP practice in New York and represents clients in a broad range of sectors, with a particular focus on biotechnology and the life sciences. She advises pharmaceutical companies in intellectual property matters related to complex patent litigation in the life sciences.