Future of generative AI in pharma
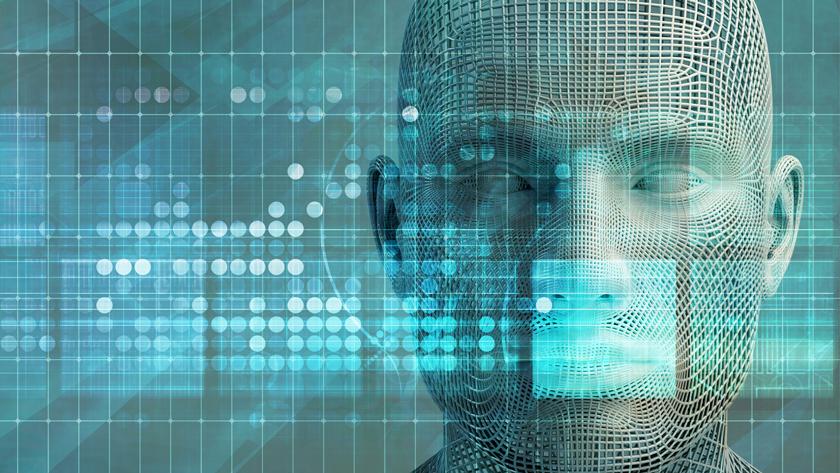
Across all industries, generative AI has become the latest buzz word. Talk of implementing automation and AI solutions to enhance human capacity continues to dominate conversations. In fact, venture capitalists have increased investment in generative AI by 425% since 2020 to $2.1 billion.
In the life sciences industry in particular, leading pharma companies are already deploying this technology across their own operations to spur productivity and efficiency for mundane, time consuming tasks. The possible applications of generative AI seem to be endless and over the past few months, new tools like ChatGPT, DALL-E, and Stable Diffusion are providing amazing capabilities that are taking the AI world by storm. However, inherent inaccuracies and errors have proven to be problematic for pharma companies looking to automate content generation.
Generative AI uses Large Language Models (LLMs) and advanced machine learning to create human-like text or imagery, pulling from existing data and experiences to generate new content. This cutting-edge technology has the potential to enhance how humans traditionally perform daily tasks and work across industries. It’s imperative that pharma companies employing AI-powered tools go beyond what existing probabilistic models are capable of. As pharma companies show more interest in leveraging components of LLMs into their workflows, it’s critical they collaborate with a vendor that takes a data and human-centric approach to implementation.
How to leverage AI automation
In order for those within the pharma industry to utilise generative AI to its fullest potential, the industry must embrace the vastness of LLMs with data that is proprietary and only available confidentially. There are a number of ways generative AI can spur innovation, productivity, and efficiency within the pharma industry, when applied correctly. Implementing AI solutions allow organisations to churn out large sums of data at an accelerated pace and at scale. Such solutions empower data scientists, medical writers, and analysts to focus on more strategic initiatives. Additional benefits to leveraging AI include:
- Acceleration of drug development: New drug application approval processes depend on the pace of development. Pharmaceutical companies often face obstacles, such as bringing drugs to market at a rapid pace, especially before their competitors. AI quickens the process of manufacturing drugs. Additionally, reporting paired with this process can be extremely time consuming and instead of taking up large amounts of an employee's time, an AI solution can alleviate that task and collect the data and information much quicker and seamlessly.
- AI solutions successfully identify disease patterns in larger datasets: Humans are not wired to process large amounts at a rapid pace and especially not error-free. Automation allows organisations to eliminate human inconsistencies, while providing exact and valued work.
- AI assisting medical writers to create fast, accurate reports: Mundane and time-consuming tasks often create low-morale, causing many to feel unmotivated to complete these parts of the job. By allowing employees to hand off tasks such as data collection and reports, they are then able to focus on other more enjoyable or strategic components to the job. Empowering employees is essential in ensuring no one feels burnt out.
Implementing generative AI is essential for pharma
Generative AI is revolutionising content automation for all industries, but has the potential to significantly disrupt the way pharma companies have approached traditional processes. While there’s great potential for pharma, automation in such a highly regulated document landscape is complex and should be approached with caution. As more pharma companies begin to leverage components of LLMs into their workflows, it is critical they collaborate with a vendor that takes a data and human-centric approach to implementation. Training models using LLMs and symbolic AI is essential to ensure reliable and credible results. The key is to consider many fundamental customer needs, such as data isolation and security, transparency and explicability of AI models, bias and fairness, and the auditability of results. Since medical writers work with confidential, sensitive patient data, a secure and reliable option is needed.
According to Brian Burke, Research VP for Technology Innovation at Gartner, it’s anticipated that over 30% - up from zero today - of new drugs and materials could be discovered using generative AI solutions by 2025. Only time will tell what’s to come with generative AI, but one thing which is certain is that it harnesses the power to significantly impact pharma and additional highly regulated industries.
About the author
Emmanuel Walckenaer is the CEO of Yseop, an AI software company using Natural Language Processing (NLP) to automate content generation for highly-regulated industries. Walckenaer brings to Yseop over 25 years of international experience in high-tech service and business development.