US researchers create AI capable of diagnosing depression
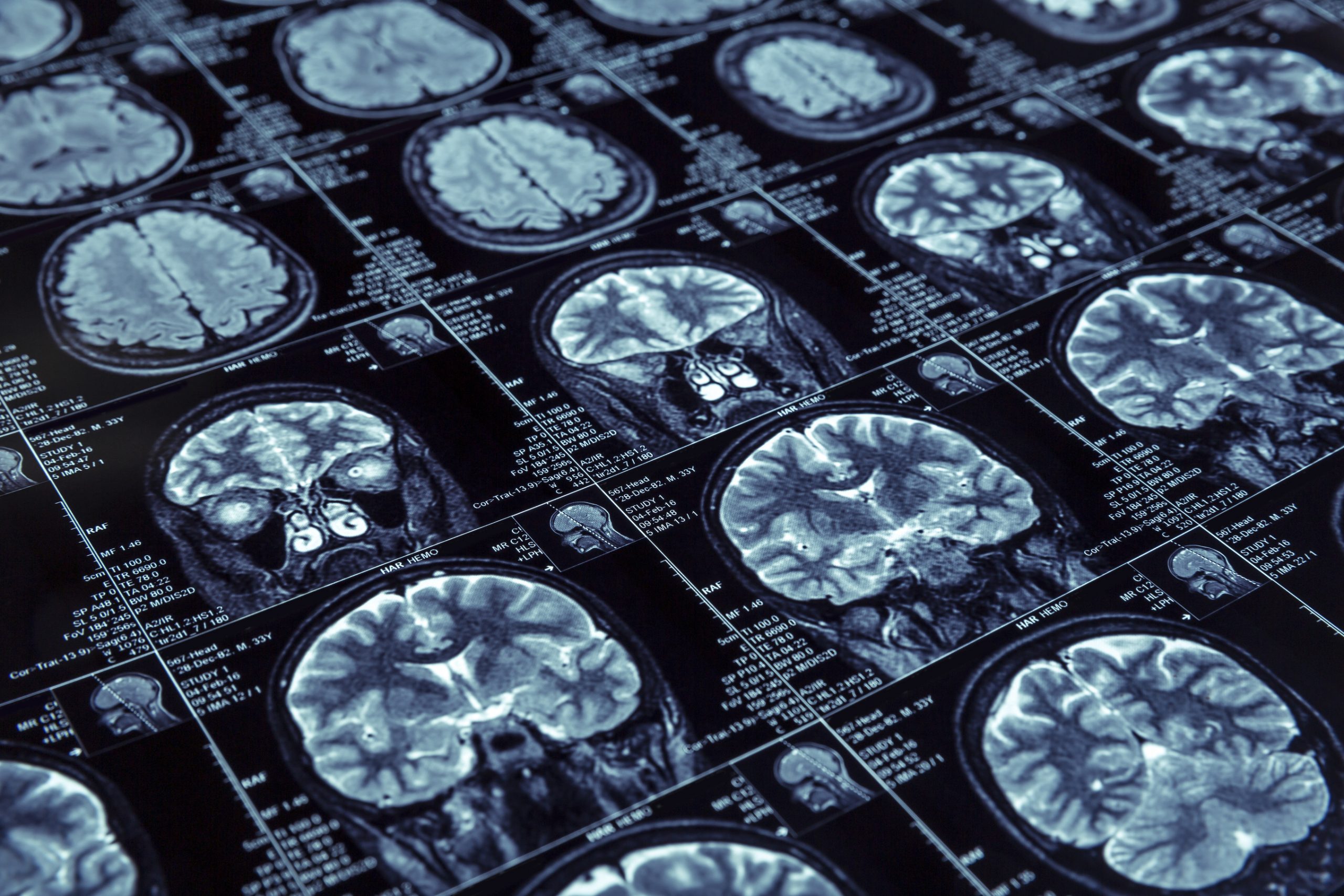
A machine learning algorithm has been trained to detect the risk of depression from brain scan data.
In a study at the University of Texas at Austin, an AI algorithm trained with sample data from previous brain scans, split into those belonging to either healthy or depressed patients, accurately predicted major depressive disorder in 75% of cases.
A total of 52 treatment-seeking participants were included in the study, alongside 45 healthy control participants, all of which received diffusion tensor imaging (DTI) MRI scans.
DTI MRIs tag water molecules to measure the amount of diffusion into the brain over time. Measuring this diffusion helps researchers understand the neural activity of specific parts of the brain.
The scans were analysed by the algorithm and identified as either healthy or depressive, based on their likeness to patterns identified in pre-loaded training scans.
"Not only are were learning that we can classify depressed versus non-depressed people using DTI data, we are also learning something about how depression is represented within the brain," said Christopher Beevers, a professor of psychology and director of the Institute for Mental Health Research at UT Austin. "Rather than trying to find the area that is disrupted in depression, we are learning that alterations across a number of networks contribute to the classification of depression."
With the help of machine learning, the millions of interactions between neural networks and their complex relationships can be automated, making an achievable goal of a previously impossible job for one physician to undertake.
The technology also goes one step further than machine learning algorithms currently in use by companies such as Facebook which analyse language to identify behaviours associated with depression and intervene accordingly, regardless of a medical diagnosis.
Theoretically, this new algorithm could act as a diagnostic allowing for depression to be identified and treated earlier than current methods.
There is still space to improve the algorithm, according to David Schnyer, a cognitive neuroscientist and professor of psychology at UT Austin, who is now looking to integrate data from other sources such as genomics to give a clearer, more holistic application as a clinical metric.
The algorithm will next be tested in a population of several hundred volunteers from the Austin community diagnosed with depression, anxiety or another mental health disorder.