Machine learning puts virtual drug screening into high gear
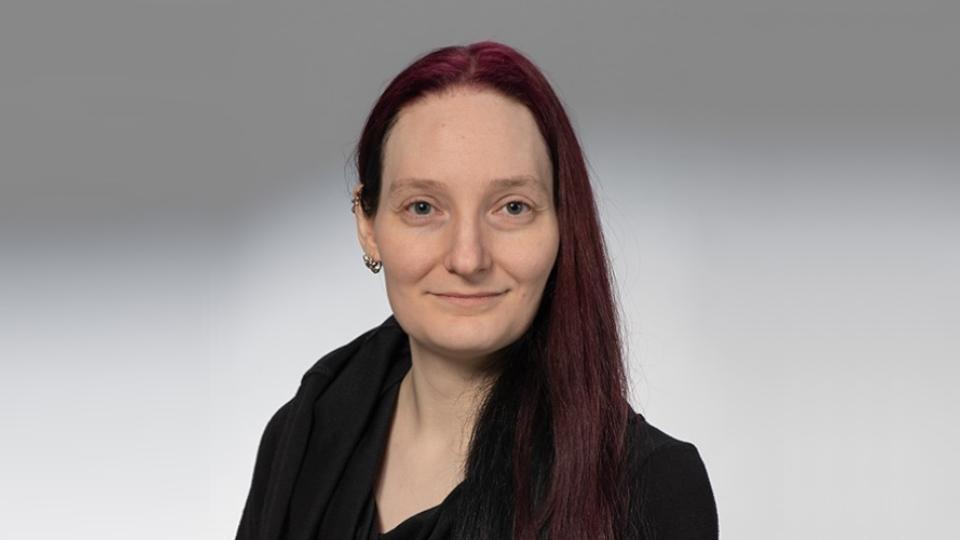
Dr Ina Pöhner of the University of East Finland
Researchers in Finland have combined virtual drug screening with machine learning (ML) – carried out on supercomputers – to dramatically shorten the time taken to identify candidate molecules.
Virtual screening of large compound libraries is already deployed routinely in the pharma industry to seek out potential drug candidates, but a massive increase in the number of compounds to be screened in recent years has made that a process that can take months or even years.
Now, a team led by scientists at the University of Eastern Finland (UAF) say they have achieved a 10-fold reduction in the time taken to screen 1.56 billion drug-like molecules, finding 90% of the best candidates that could ‘dock’ with two pharmacological targets in less than 10 days.
Using conventional approaches, even with supercomputers, the docking screening process would have taken more than six months, according to the researchers, led by Dr Ina Pöhner (pictured above) of the UEF’s School of Pharmacy.
The work was supported by Orion Pharma and CSC - IT Center for Science, which hosts the supercomputers – called Mahti and Puhti – that were used in the project.
Orion developed the artificial intelligence-powered HASTEN tool, used to accelerate the virtual screening process and predict which compounds would bind to the two experimental targets, a bacterial chaperone protein and a viral kinase.
The scientists, who have published a paper on their work in the Journal of Chemical Information and Modeling, say it is the first rigorous study to compare an ML-boosted docking screen with a conventional ‘brute force’ docking approach that would run the simulation against every drug candidate in the library.
“With HASTEN, we observed robust recall of 90% of the true 1,000 top-scoring virtual hits in both targets when docking only 1% of the entire library,” write the authors in the paper.
According to Orion’s Dr Tuomo Kalliokoski, who led the development of HASTEN, the tool uses ML to learn the properties of molecules and how those properties affect how well the compounds score, and could dramatically speed up drug discovery.
“When presented with enough examples drawn from conventional docking, the machine-learning model can predict docking scores for other compounds in the library much faster than the brute-force docking approach,” he said.
The team is releasing the datasets generated as part of the study into the public domain, along with a ready-to-use screening library and the entire 1.56 billion compound-docking results for the two targets, which can be used as benchmarking data by other researchers.
“This data will encourage the future development of tools to save time and resources and will ultimately advance the field of computational drug discovery,” they said.