Draper aims to improve AI lung cancer detection systems
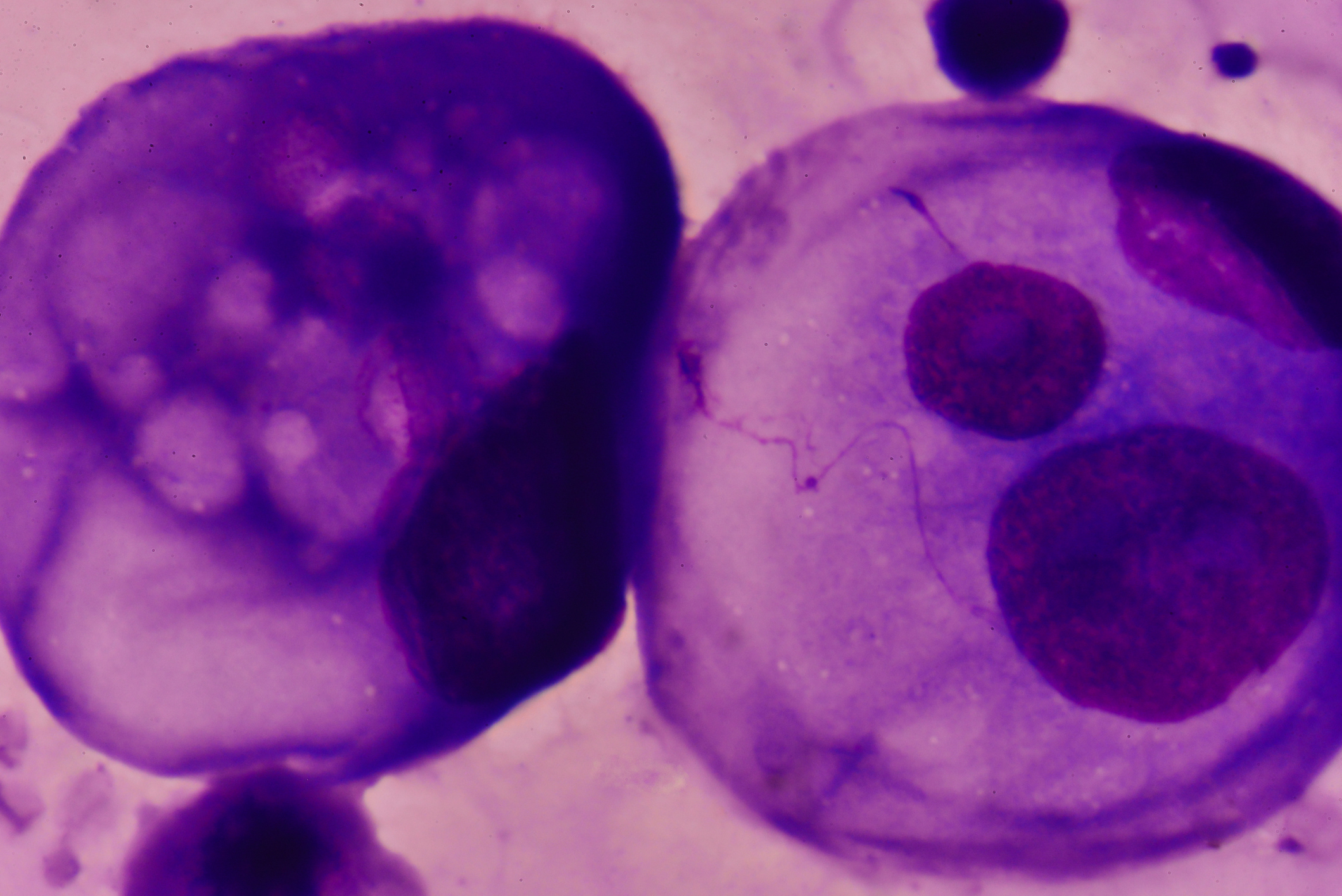
US firm Draper has announced it is aiming to make artificial intelligence (AI) systems more adept at detecting lung cancer warning signs in scans.
The high mortality rate associated with lung cancer is in part because symptoms become apparent only after the cancer is at an advanced stage.
A team from Massachusetts-based Draper is looking at ways to identify high-priority cases from scans.
The group is looking at an area of data science that uses artificial intelligence and machine learning to improve clinical decision-making in healthcare and other fields.
They hope to find ways to help radiologists who already use AI software to look through hundreds of 2D images within a single CT scan to spot cancer.
But without a built-in way to identify high priority cases and suspicious regions in the scans, there is no confidence estimate that can guide radiologists towards the most troublesome-looking images.
Technician Andrew Berlin said: “While today’s AI software is often able to identify suspicious findings on images, systems that provide a confidence estimate in their conclusions are very rare.
“This leaves the physicians uncertain when to trust the software - is it certain that a lesion is suspicious, or is it unclear and the software is just making its best guess?”
With his Draper colleagues, Berlin designed a new computer-aided detection and diagnosis system for lung cancer screening with low-dose CT scans that not only detects suspicious regions, but also provides the physician with a confidence level in those detection results.
The system operates by using a 3D convolutional neural network (CNN) that has been modified to not only classify which regions of a scan look suspicious, but also to calculate how confident the neural network is in its conclusions.
In a research paper by Berlin and Draper machine learning scientist Rebecca Russell, the two authors said the next stage of development is to improve datasets to reduce the number of false negatives.
Authors wrote: “Although our system demonstrates that 3D CNN models can be effectively used to analyse lung CT scans, the performance is still bounded by the limitations of the datasets used to train our models.”