Deep learning model ‘detects 90% of lymphatic cancer cases’
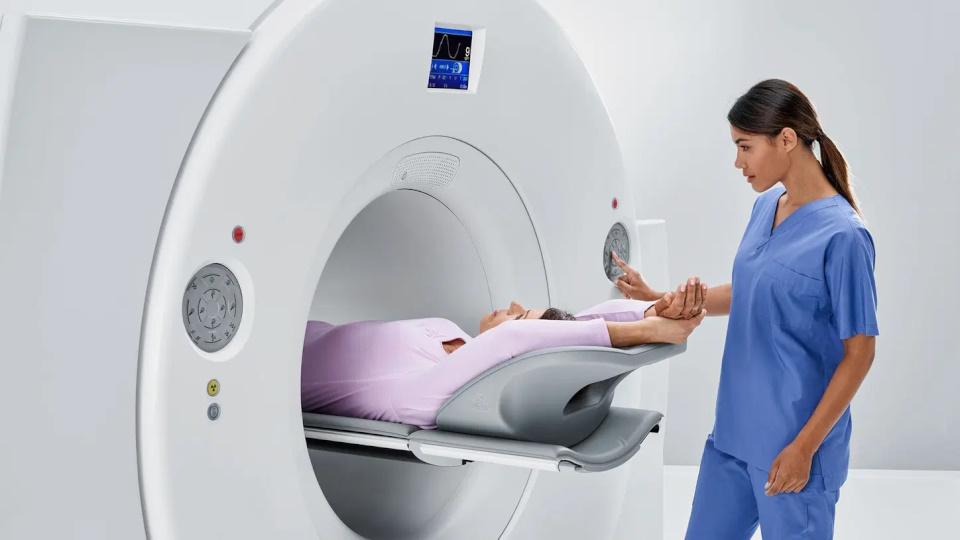
Researchers have developed an artificial intelligence algorithm to assist in the diagnosis of lymph node cancer from medical images that has an accuracy of around 90%.
The team carried out a retrospective study on the deep learning model, using PET-CT scans from more than 5,000 patients treated for lymphoma at Memorial Sloan Kettering Cancer Center in the US and the Medical University of Vienna in Austria.
The Lymphoma Artificial Reader System (LARS) algorithm was trained on 80% of the images, and tested for accuracy on the remaining 20%, comparing the results with the patient’s final diagnosis, according to a paper published in The Lancet Digital Health.
The study is thought to be the largest ever to apply deep learning to PET-CT scans and showed a balanced accuracy in detecting whether or not an image had a lymphoma of 87% to 91%. There was also remarkable consistency in the results, despite the use of different equipment and scan procedures by the US and Austrian clinical teams, according to the authors.
The scientists say LARS could reduce the workload for radiologists, by giving a second opinion or ranking which patients need treatment the fastest, pointing out that a massive rise in demand for PET has made it hard for some health systems to provide timely scans.
Figures from the Organisation for Economic Co-operation and Development (OECD) show a 33·5% increase in PET scans between 2015 and 2020, and the number of diagnostic imaging specialists has struggled to keep pace, particularly in low- and middle-income countries.
“An AI-based computer system for interpreting medical images […] contributes to increased equality in healthcare by giving patients access to the same expertise and being able to have their images reviewed within a reasonable time, regardless of which hospital they are in,” said lead author Ida Häggström, associate professor in electrical engineering at Chalmers University in Sweden.
She added that LARS was developed using an approach known as supervised training, which means they "haven’t programmed predetermined instructions in the model about what information in the image it should look at, but let it teach itself which image patterns are important in order to get the best predictions possible.”
Häggström acknowledged, however, that there is still a great deal of work to be done to validate the deep learning model and allow it to be used in clinical practice.
“We have made the computer code available now, so that other researchers can continue to work on the basis of our computer model, but the clinical tests that need to be done are extensive,” said Häggström.