AI used to accurately predict risk of patient death
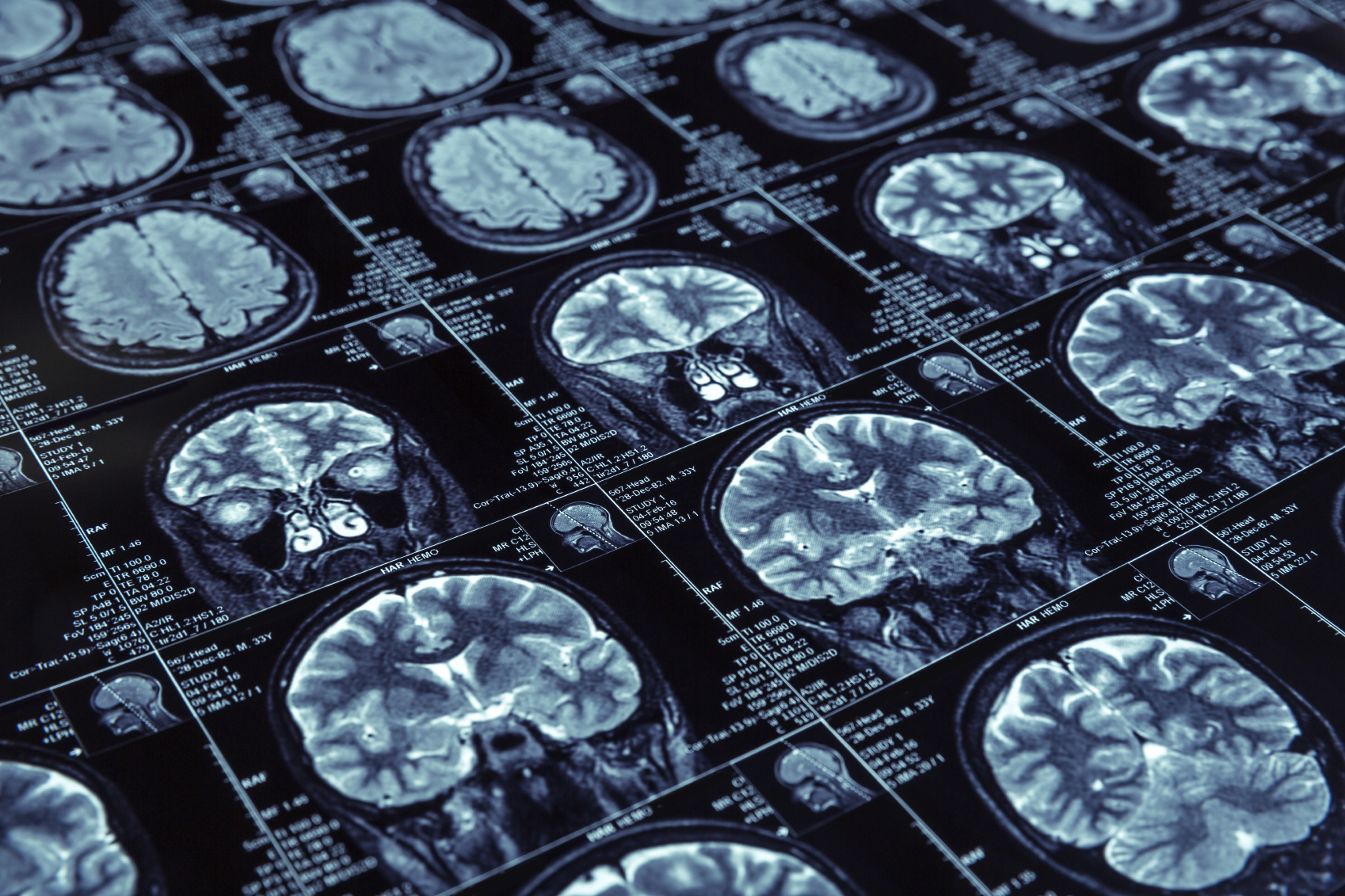
Researchers in Australia are using AI to predict patient death to a high level of accuracy.
Created at the University of Adelaide, the software predicted which of 48 patients would die within five years with close to 70% accuracy.
The technology uses deep learning principles to search CT scans for signs of disease.
It does so by analysing brightness levels of pixels within each scan and assigning a value to them. The software then searches for a pattern within these values.
In this study, the AI's most accurate predictions were for patients with chronic conditions like emphysema and congestive heart failure.
“There is only a certain amount of information humans can see when looking at scans but research suggests there is more we can see using computers,” said Dr Luke Oakden-Rayner, lead author of the paper. “Using deep learning we are able to understand and analyse organs in a way doctors can’t do at the moment.”
Future health
By letting doctors look at medical outcomes rather than diagnoses, it can predict a patient's future health, he said.
The study is the first of its kind in terms of entirely relying on clinical imagery to calculate longevity.
Other studies include factors such as age and gender to help guide these predictions, and such studies tend to achieve accuracy levels of around 60-70%.
In this case, these factors were excluded. However, coupling this data with the new AI could lead to significantly more accurate success in predicting death.
The study has strong implications for personalising treatment. It could aid in predicting how a disease will progress as well as evaluating efforts to halt progression.
The system could also be used to gauge surgery response or chances of injury, according to Oakden-Rayder.
AIs are proving to be particularly effective in predicting future health outcomes. In research by the Cincinnati Children’s Hospital Medical Center, an AI identified suicidal people from written responses with 95% accuracy. It could differentiate between suicidal participants and those that were mentally ill but not suicidal to an 85% level of accuracy.