AI chatbots struggle to interpret patient descriptions
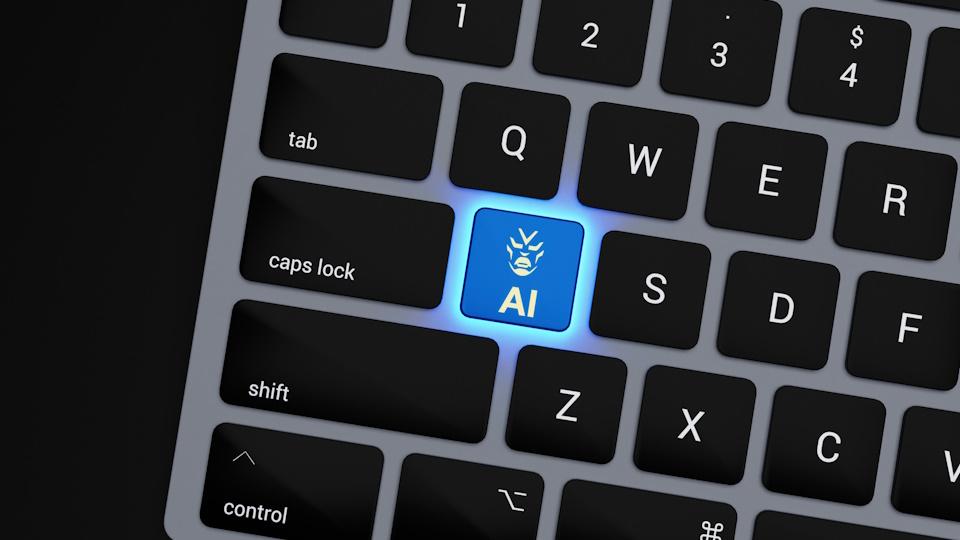
Artificial intelligence tools based on large language models (LLMs) find it hard to identify genetic conditions based on patient-written descriptions, suggesting there is some way to go before they should be applied generally to clinical settings.
That's the finding of a new study from National Institutes of Health (NIH) researchers in the US, who looked at a range of LLMs – including the latest versions of OpenAI's ChatGPT and Google's Bard – to see if they could identify genetic conditions from questions using medical terminology or based on the everyday wording that might be used by patients.
LLMs are machine learning models that can comprehend and generate human language text and are trained on massive amounts of text-based data. For some time now there has been interest in using them in clinical practice to analyse and respond to patient questions about their health and assist in decision-making by healthcare professionals.
Drawing from medical textbooks and other reference materials, the researchers designed questions about 63 different genetic conditions, ranging from well-known conditions like sickle cell disease, cystic fibrosis, and Marfan syndrome, to disorders that are much rarer and obscure.
They selected three to five symptoms for each condition and generated questions phrased in a standard format: "I have X, Y, and Z symptoms. What's the most likely genetic condition?"
They found that the LLMs ranged widely in their ability to point to the correct genetic diagnosis when medical textbook terms were used, with initial accuracies between 21% and 90%, and with the success rate tracking with the size amount of data on which they had been trained. The best-performing model was GPT-4, one of the latest versions of ChatGPT.
Replacing medical terms with layperson's language – for example "macrocephaly" with "a big head" – had a dramatic impact on their performance, however, with no LLM showing accuracy above 21% and some scoring as low as 1%.
That said, 7 out of 10 of the models were still more accurate than Google searches when using common language, according to the researchers, and rewriting the patient responses in a standardised format improved the accuracy.
The results expose the limitations of LLMs, and the continued need for human oversight when AI is applied in healthcare, but also point to the ways in which they could become useful in future, according to the scientists.
"These technologies are already rolling out in clinical settings [and] the biggest questions are no longer about whether clinicians will use AI, but where and how clinicians should use AI, and where should we not use AI to take the best possible care of our patients," said Ben Solomon, senior author of the study and clinical director at the NIH's National Human Genome Research Institute (NHGRI).
"For these models to be clinically useful in the future, we need more data, and [that needs] to reflect the diversity of patients," he added. "Large language models have been a huge leap forward for AI, and being able to analyse words in a clinically useful way could be incredibly transformational."
The research is published in the American Journal of Human Genetics.
Earlier this year, a health-focused LLM developed by Google called AMIE trained specifically to carry out patient consultations was shown to be more accurate than primary-care doctors in diagnosing some illnesses and scored higher on empathy.
Photo by BoliviaInteligente on Unsplash