Responsible integration of AI in pharmaceutical labs
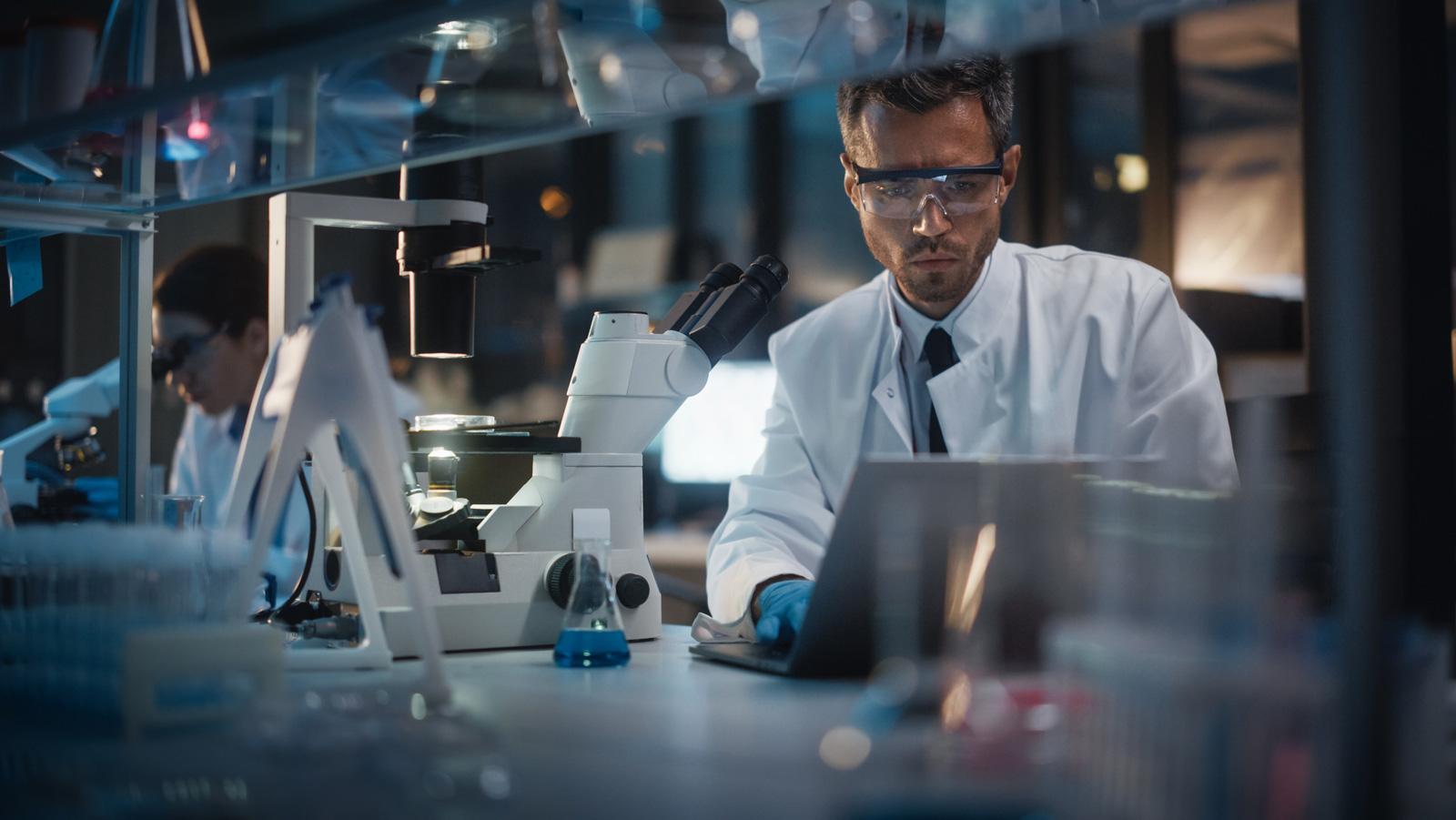
As applications of generative AI (GenAI), and AI in general, extend to every industry, the dialogue surrounding its responsible integration remains front and centre. President Joe Biden's new Executive Order on Safe, Secure, and Trustworthy Artificial Intelligence breaks down a long list of concerns as it relates to security, privacy, equity, consumer rights, labour, and more. A call for clearer guidelines is also at the industry level, and especially strong in pharma and biotech.
AI and the pharmaceutical market are an ideal match. Drug discovery and development companies are burdened with intense competitive and financial pressure to shorten timelines and accelerate innovation. One drug can take 10-15 years and up to $12 billion in cost to develop and get to market, if it successfully beats the 90+% failure rate. AI has the potential to shave years off timelines and save billions of dollars, all while increasing the rate of success. And AI providers have responded. In fact, Precedence Research shows that AI in pharma was valued at $905 million in 2021 and is estimated to surpass $9.24 billion by 2030. AI has already transformed the industry to the point that the technology is now essential to be and stay competitive.
However, as we’ve all now learned, while mind-blowing in its capabilities, AI and especially generative AI, has its downsides. And when it comes to the health and well-being of real humans, there is very little margin for error. Before AI in all its forms can truly benefit the pharma and biotech industries, and ultimately patients, the risks and ethical challenges that come with the technology must be addressed. Below are three guiding principles to keep in mind when considering integrating AI into your R&D organisation.
Principle #1: Protect your intellectual property (IP)
A pharma company’s IP and lifeblood is its scientific data. Your data holds the secrets to novel discoveries, key findings, critical decisions, and proprietary innovations. Keeping R&D data secure and private should be the first and foremost priority when integrating AI. This need is even more pronounced with the recent rise of generative AI.
Generative AI models are especially data hungry and require massive amounts of learning data. Every prompt from the now 180.5 million users of ChatGPT has been used to further train the model since its entry into the world just a year ago. Over time and relatively quickly, OpenAI and others introduced “secure” enterprise versions, but what is going on under the hood remains a bit opaque.
When considering the many AI options, you must be diligent and ask two main questions: ‘Is my (IP) data being used to train models outside my company?’; and ‘Who owns the output if I use these tools?’ Regulations and standards around copyright, trademark, and other legally binding protections are complex and evolving, and the answers to these questions remain unclear. A full understanding of AI’s implications around IP protection will require further adaptation and testing to truly understand the nuances – something scientists, especially, can appreciate. In the meantime, it is important to work to consensus internally on what confidence level is acceptable and what standards to follow within your own organisation while we all await official policy and regulations from governing bodies all over the world.
Principle #2: Mitigate biases and harmful impacts
Before the risks around AI can be mitigated, they must first be understood. Scientists and R&D leaders need to keep some key hazards top of mind so they can combat them effectively, if and when they arise. Two primary hazards, that can both discount the benefits of using AI as well as derail authentic innovation, are model bias and automation bias.
Model bias is when the model’s algorithms systematically produce biased results due to biased inputs and assumptions. For example, any generative AI tool that pulls data from the internet (written content, images, etc.), a forum that is inherently fraught with biases, is immediately biased in its results. Just seven months ago, when we prompted “scientist”, Midjourney returned only older, Caucasian males. Model bias can be resolved, however, as long as you are sensitive to bias potential in the first place and then balance with additional inputs and proactive training.
Automation bias is the tendency for people to trust the output of a computer more than a person, ignoring conflicting information. Again, balance is required. Automation bias can contribute to dangerous mistakes, especially when AI models are not transparent or explainable. Similar to new drugs, AI systems should only be used after being thoroughly researched, vetted, and tested, with humans always involved in the process. Design checkpoints into workflows and build a QC process around technology in your organisation.
Principle #3: Champion a future-proofed workplace
We typically see three levels of individual buy-in when it comes to AI, often at mixed levels within one R&D team. The first are the sceptics who do not believe AI lives up to the hype and are unsure about its true capabilities. The second believes in the power of AI, but does not know how or where to get started for their lab. The third are fully bought in and already started with some AI tools, and may or may not be finding success with them. Regardless of what level of buy-in exists within a company, an R&D organisation, or a lab, there is shared acknowledgement that AI is forever changing pharmaceutical research. And scientists need to be prepared.
This fundamental shift in the industry requires organisations to ensure their workforce - their domain experts - are properly trained to be users of AI and also to have a heightened understanding of the associated risks. AI is changing the role of the scientist in the lab, but humans will always be needed to guide and monitor it. The more knowledge your team has about AI use cases, biases, and risks, the more confident they will be to work alongside it, as well as know when to raise doubts and concerns about certain systems or results. Only then will they be enabled to truly leverage AI to answer new research questions and solve the healthcare problems of the future.
Conclusion
These three principles provide a strong foundation for safe and responsible AI deployment for pharmaceutical and biotech companies. Protecting the IP that lives in your data, mitigating model and automations biases, and continuing to lean into how AI and humans can work together are strong starting points when developing your approach, but it really is just the beginning. Integrating AI into a lab is already complex, and even more so when compounded with the challenges around its responsible deployment.
The most important step is to keep moving forwards on the path of integrating AI into your technology solution set. Advancements are only accelerating and stalling action will further widen the gap between market winners and losers. Begin the process of getting internal stakeholders aligned on expectations and a balanced approach, and involve scientists from the beginning. An experienced external partner who understands the technology behind safe and secure implementation of AI, combined with a deep understanding of the complexities of pharmaceutical research, is especially valuable at this stage. Though AI is currently outpacing policy and regulation, it is absolutely feasible and realistic to responsibly integrate it into today’s labs and bring new medicines to market faster than ever before.