An overview of AI in pharma – Part 1
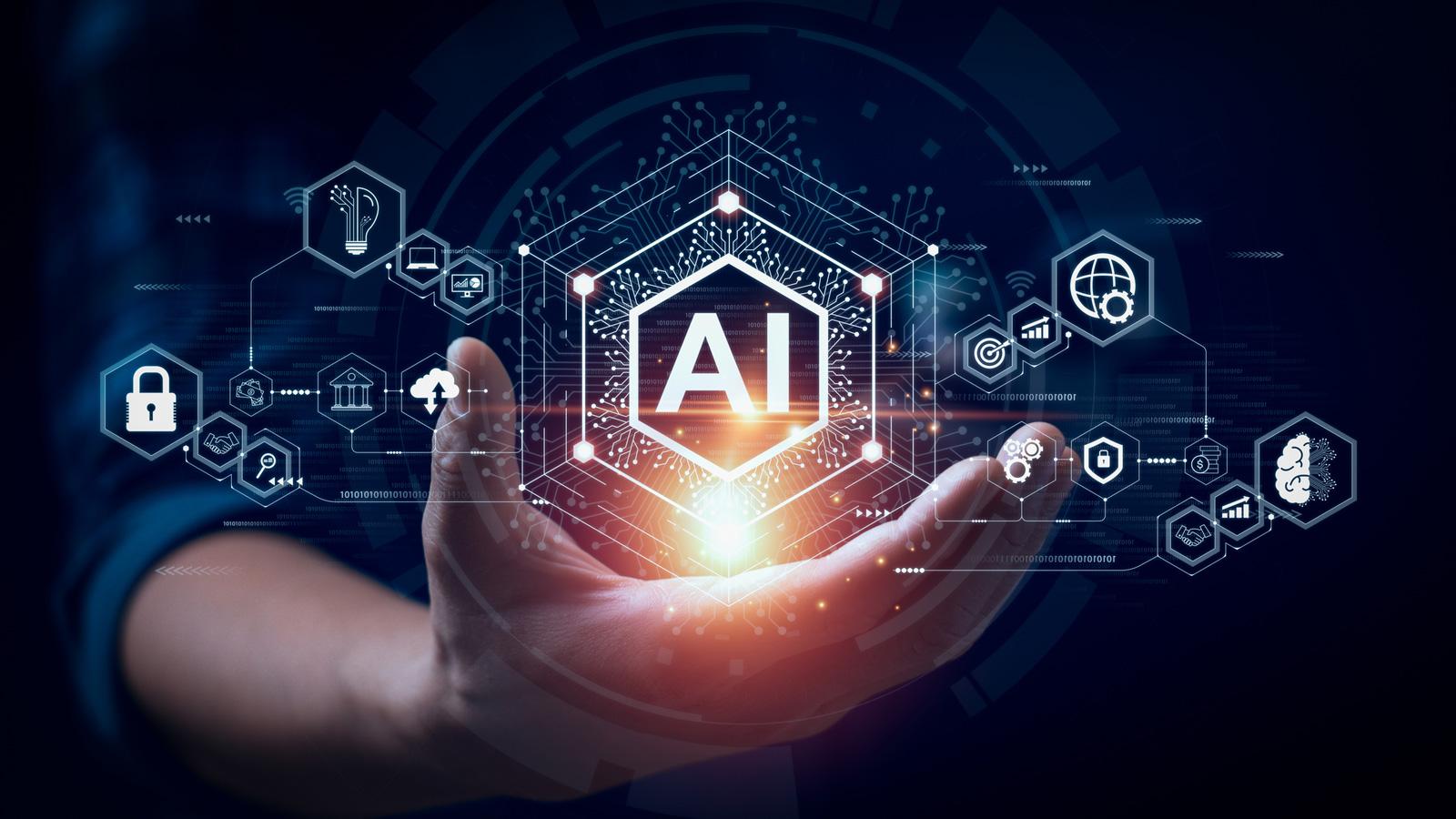
In the dynamic realm of modern pharmaceuticals, the convergence of artificial intelligence (AI) has emerged as a transformative catalyst, propelling companies towards unparalleled competitiveness and resounding success. With an ever-increasing demand for pioneering treatments, personalised medicine, and streamlined drug development, the pharmaceutical industry is awakening to the revolutionary capabilities of AI technologies.
From the inception of novel drugs to their development through rigorous clinical trials, the indelible footprint of AI is revolutionising diverse facets of this industry, empowering expeditious and astute decision-making, optimising research endeavours, speeding up clinical trials, improving market access, personalising patient and physician engagement, and, above all, augmenting patient outcomes to unprecedented levels of excellence.
Embracing AI in pharma, often referred to as pharma AI, is no longer just an option for pharma companies; it has become an indispensable tool for staying ahead in a fiercely competitive market. The strategic integration of AI in pharma holds the key to unlocking new frontiers of progress and gaining a significant advantage over rivals.
This article delves into the pivotal role of artificial intelligence pharma in the pharmaceutical environment and explores the myriad ways AI is reshaping the industry's landscape.
What is AI?
Artificial intelligence (AI) is a branch of computer science that aims to create machines capable of performing tasks that typically require human intelligence. The overarching goal of AI is to simulate human cognitive abilities, such as learning, reasoning, problem-solving, perception, and decision-making in machines. The field of AI has evolved significantly over the years, encompassing various techniques and approaches to achieve its objectives.
Some examples of types of AI are machine learning (or ML, and many subtypes such as Artificial Neural Networks, Deep Learning, Generative AI, etc.), Natural Language Processing (NLP), computer vision, expert systems, robotics, and automation, among others.
What is Generative AI
Generative AI is getting a lot of press now due to the highly publicised ChatGPT. Generative AI is a branch of artificial intelligence (and a sub-type of Artificial Neural Networks) that focuses on creating machines capable of generating new and original content, such as images, videos, music, text, and more. Unlike traditional AI models that are designed for specific tasks and require extensive human input and labelling of data, generative AI systems can autonomously produce data that resembles human-generated content.
It was really honed in 2014 with the advent of Generative Adversarial Networks (GAN). There are several techniques used in Generative AI, but this was the first and is the most prominent one. GANs consist of two neural networks: a generator and a discriminator. The generator's main task is to create new data instances, while the discriminator's role is to distinguish between real data (e.g., actual images, text) and fake data (e.g., generated images, text).
Examples of Generative AI:
Text Generation
- Language models: AI models like GPT-3 can generate human-like text, including articles, stories, poems, and more.
- Chatbots: Conversational AI systems that can generate responses in natural language, based on input from users.
Image Generation
- Generative Adversarial Networks (GANs): AI systems that consist of a generator and a discriminator working in tandem to produce realistic images from scratch.
- Variational Autoencoders (VAEs): AI models that can learn and generate images by encoding and decoding them in a latent space.
Music composition
AI systems trained on music datasets can compose new pieces of music in various genres and styles.
Art Generation
AI-powered art generators can produce paintings, drawings, and other forms of visual art.
Video Generation
Some AI models can create new videos, either by generating frames sequentially or by modifying existing videos.
Code
Generative AI can be used to automatically create code. However, in testing this we discovered that, while it is great at generating code for existing problems, it has been trained on, it is not good at generating code for unique new challenges… Yet.
3D models and designs
Generative AI can be used to create 3D models and designs for architecture, product design, and more.
Game content
- AI can be used to generate levels, characters, and other content in video games.
Data augmentation
- AI can be used to generate synthetic data for training machine learning models, helping to improve their performance. We have used it to create new data for rare disease patients that allows vast amplification of the data sets to allow unique insights that can then be used by pharma.
Challenges faced by pharma
The transformative power of AI in pharma plays a critical role in developing and delivering life-saving medications and treatments to patients across the globe. Nevertheless, this indispensable sector faces an array of challenges that profoundly impact drug discovery, development, manufacturing, and distribution. These multifaceted challenges stem from myriad factors, encompassing scientific, regulatory, economic, and ethical considerations.
Examples of challenges faced by the pharmaceutical industry:
- Rising R&D costs with uncertain outcomes
- Lengthy development timelines
- Difficulty recruiting patients for clinical trials in a timely manner
- Stakeholder fragmentation
- Supply chain challenges
- Regulatory hurdles
- Drug pricing and reimbursement constraints
- Inflation
- Shift to value-based healthcare
- Pushback on specialty drugs
- Drug safety and adverse event management
- HCP access
- Data security and privacy
- Patent expirations and generic / biosimilar competition
- Upcoming patent cliff size
AI throughout the pharma value chain
AI has been making significant strides throughout the pharmaceutical value chain, transforming various stages of drug discovery, development, manufacturing, and patient care. Its integration has accelerated processes, improved efficiency, and yielded promising results.
Here are just a few examples of how AI is impacting the pharma industry:
Drug discovery and R&D
AI algorithms can predict the properties and behaviour of molecules, enabling more efficient drug design. For example, for Covid-19, Insilico used AI to generate tens of thousands of novel molecules with the potential to bind a specific SARS-CoV-2 protein and block the virus’s ability to replicate. A deep learning filtering system narrowed down the list to 100 molecules after four days. Applying AI here can reduce development time significantly. Another example is Benevolent AI, who uses AI to analyse biological data to identify drug targets. They have done this successfully for AstraZeneca.
Drug repurposing
AI can screen existing drugs for new therapeutic uses. For example, researchers DearGen in South Korea used AI to predict out of FDA-approved HIV medication that atazanavir was the most likely to bind and block a prominent protein on the outside of SARS-CoV-2. At the same time, Benevolent AI identified six compounds, from which baricitinib looked to be the best of the group for both safety and efficacy against SARS-CoV-2. Interestingly, in both cases the clinical trials confirmed these predictions and what was even more interesting is that, when used in combination, these together provided even more powerful efficacy against COVID.
Clinical trials
AI-powered techniques can vastly improve clinical trials, from designing the pre-clinical experiments, to running them, designing the trial protocols, recruiting for trials, and more. For example, AI is being used to analyse cancer biomarkers in 60 seconds from a drop of blood using an at-home device slightly bigger than an Amazon Echo to optimise oncology drug development with a biomarker monitoring platform and millions of patient datapoints. And of course, automating the writing of the Clinical Study Report (CSR) in less than an hour to be 90% complete.
Supply chain optimisation
AI can optimise pharmaceutical supply chains by predicting demand, monitoring inventory levels, and optimising distribution routes. This improves efficiency and reduces costs. A McKinsey report estimated that AI-driven supply chain optimisation could lead to cost reductions of up to 30% for pharmaceutical companies.
Regulatory
We have used AI to ease the time-consuming human tasks. For example, even when a dossier is complete, the ongoing guidance from authorities such as World Health Organization often means that parts of the dossier needs to be modified or adapted. AI can speed this process up by reading both and highlighting the part in the dossier that needs amending (and why) and even suggest amends.
Medical Affairs
AI-powered NLP techniques can mine vast amounts of scientific literature, patents, and clinical trial data to extract valuable insights. These are found to increase productivity by 1000% and reduce literature monitoring time by between 82-92%.
Drug pricing and market access
AI can analyse real-world data and market dynamics to optimise drug pricing and assess market access opportunities. AI can be used to create a Global Value Dossier (GVD), keep it automatically updated, create value pricing, and even analyse different reimbursement bodies to identify additional factors on top of drug price that are impacting their decisions so these can be included very early on in trial design, as well as in the writing of the submission dossiers.
Drug safety and pharmacovigilance
AI-powered systems can analyse adverse event reports, social media data, and other sources in order to identify potential safety issues with drugs and medical products. These have been found to dramatically reduce human time and costs (15-20% in agent/vendor costs in data entry, quality review, translation, reconciliation, and redaction, then 40-45% reduction in cost for triage, data re-entry, quality checks, MR and submit, and 15-20% in both signal detection and support costs).
Salesforce
Salesforce has so many ways AI can be applied. For example, precision HCP targeting, HCP switch prediction, HCP personality profiling with suggestions on the optimal way to approach each person with language style and content, personalised messaging (both content, and channels and sequence), AI whisper coaches to listen in on calls and offer tips to improve the call impact, and even automating the filling in of CRM fields.
Marketing
Again, in pharma marketing there are so many ways to employ AI, from identifying KOLs with impact rather than simply volume, pre-launch opportunity identification, finding rare disease patients, segmentation (to a segment of one!), omnichannel next best action to have the right message in the right channel at the right time by the individual, and adherence prediction and change - to name but a few.
Patient adherence and remote monitoring
AI-driven apps and wearable devices can monitor patients remotely, enhancing medication adherence and providing valuable data for clinical trials. A study published in JAMA Cardiology found that an AI-powered smartphone app successfully detected atrial fibrillation with a sensitivity of 97.9% and a specificity of 98.5%.