A look back at 2023: A Benchling biotech breakfast
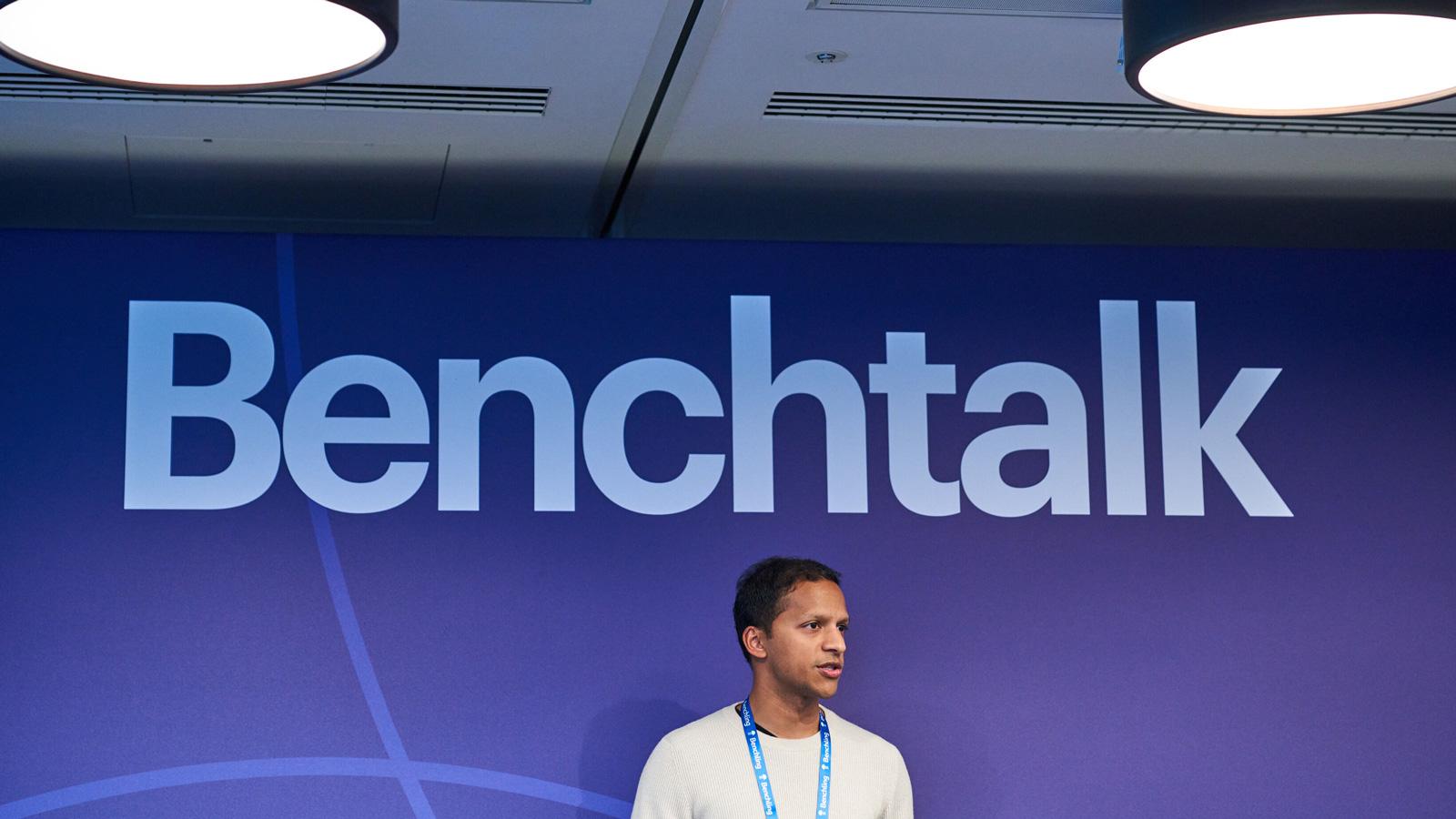
As we enter the New Year, and set forth into the myriad possibilities and plans of 2024, it pays to pause and reflect. And so pharmaphorum here harks back to Benchling’s press roundtable, run as a prelude to its European Benchtalk event in London last October.
A platform for change
Benchling creates software to power the biotechnology industry. The premise of discussions on 24th October was contemplation of the state of tech in biopharma, titularly inspired by Benchling’s inaugural report, finding that biotech is possibly “the greatest platform for change and education since the internet.” Included was also an admission that ‘excitement’ is the word most frequently uttered in conversations surrounding this topic.
Ahead of the press event, pharmaphorum briefly had a chance to speak with Bob Burke, Benchling’s general manager EMEA, about the ‘excitement’ around Benchtalk in London in 2023, the registration target having been exceeded. This, he said, despite the poor economic climate and a conservative year’s experience in terms of investment in digital products.
Summary of findings
The Benchling report on the state of tech in biopharma found that biopharma has shown a widespread adoption of enabling technology, and that that increased adoption has led to a highly complex environment for scientists and R&D IT teams. Such enabling technology includes R&D data platforms, robotics and automation, connected lab instruments and equipment, cloud-based (SaaS) scientific applications, and AI and machine learning (ML).
Indeed, if challenges are addressed, it found that there is then high potential for resultant impact – but those challenges include the fact that, as yet, R&D and IT do not align on where to prioritise investment with that enabling tech.
When conventional methods are not enough
The roundtable discussion included Saji Wickramasekara, co-founder and CEO of Benchling, together with James Field, CEO of LabGenius; Uzma Choudry, tech and biotech investor at Octopus Ventures; Emmanouil Metzakopian, VP of research and development at bit.bio; and Pierre Salvy, head of engineering at Cambrium. The participants began with a run-through of their various approaches in the biotech arena.
Lab Genius’ James Field explained that synthetic biology, macroscopic engineering - none of it had worked. So, he took an alternative approach with ML molecules, permitting the engineering of novel therapeutic antibodies. These, he said, wouldn’t have been found with conventional methods.
Emmanuel Metzakopian, of bit.bio ran through the cell coding company’s work in genetic bio and cell engineering, and how it is bottleneck solving and unlocking the scalability of manufacturing. Berlin-based Cambrium’s Pierre Salvy then unpacked how designing in a computer reduces the experiment cycle in terms of time and cost. He should know: Cambrium is the fastest EU company to launch an AI product, in less than two years.
The engineering investment trend
Octopus Ventures’ Uzma Choudry, meanwhile, explained how the company had been built out of the bio-investment vertical. A bio scientist herself, she talked journalists through advances in the molecular toolkit, as well as probing tools like next-gen sequencing to tackle the huge amount of ‘knowledge’, of data. Choudry believes AI and ML are key in solving the complexity of datasets. It is for this reason, she said, that there has been an uptick in investment in companies looking at building new tools – the revolution in proteomics, for instance. Indeed, her one-sentence summary of the current investment thesis was, “Scaling biology using engineering.”
Benchling’s own Saji Wickramasekara, not a scientist, but a software engineer, told how it had struck him the first time he’d stepped into a biology lab that it was “like being transported back in time 20 years,” with an “archaic set up with notebooks for high-stakes work.” Development of the R&D Cloud, however, is overcoming this.
Real use cases
LabGenius’ Field noted his company’s interest in what can’t be adequately solved, including the problem of creating new therapeutic models excellent at differentiating between healthy and diseased cells. Looking at the existing targeted therapeutic antibodies, he said, there are nasty side effects, known from those who have been or are being treated for diseases like cancer. The question is how the diseased cell can be specifically targeted. Conventional methods limit the number of designs one can test, but, through ML active learning processes, this can be expanded.
From an investment standpoint, Octopus’ Choudry mentioned that there are a number of companies using AI given datasets on the drug discovery side. Ori Biotech, for instance, is using ML to speed up development in cell and gene therapies, monitoring cell growth in bioreactors for a closed loop system, changing the fate and destination of those cells.
In terms of a breakthrough, bit.bio’s Metzakopian, brought up the problem of transcription factors to drive reprogramming of pluripotent stem cells (iPSCs) – without knowing the codes, some 2,000 transcription factors would have to be translated, but if you take those and predict, then only one to six factors are needed for cell identity. This, he said, is “massive,” with “anything from hundreds of thousands to millions of possibilities.” And it is AI that helps with this: taking the transcription factor space in combination with the omics space for a targeted focus.
Time-saving, acceleration to market, and the talent gap
Benchling’s Wickramasekara explained that the “long, hard road” of bringing medicines to market (which can take ten years) could be ameliorated, enabling technology to reduce timelines and also labour: “There is a shocking amount of toil in sciences,” he said. However, 95% of biotechs in the world today are not modern or digitally native from the ground up, and so “science has an opportunity to improve points at every [step] of the process,” he said. It would certainly circumvent the famous example of a request to read and comment on “one million pages of data.”
For Cambrium’s Salvy, though, there is yet the issue that “biology is about twenty years behind modern tech.” He commented: “People come out of uni who can’t do data. Teams with legacy data and notebooks don’t know how to transform. So, tech is coming up to transform that – the talent gap is not so much a talent gap as a cultural gap […] Data collaboration between people is a foundational solution – [in the] next five years this could be solved with strong intent and direction.”
LabGenius’ Field agreed, reflecting that it will take a close collaboration between data scientists and biologists, aka the “unicorn of the talent gap”. To this end, Octopus’ Choudry stated that early points in education systems and syllabuses can promote the cross-pollination of engineering and biology, instead of keeping them separate systems.
Benchling: Half software, half scientists
Wickramasekara believes that the cultural challenges are tougher for those organisations who have been around for a while and have operated one way. However, he said he is cautiously optimistic: the best thing from ChatGPT and being in vogue is that it has gotten “curmudgeonly folks” to consider the possibilities. There is now an awareness, which is, he said, “an incredible motivator.”
In consideration of overall impact, it was noted that a survey had looked at the highest areas of impact with tech. Unsurprisingly, AI/ML were lowest in terms of expected impact. For LabGenius, it’s all dependent on the end form of the molecule, though. Field thinks there’s a nuance to the way in which the scientific process is changing, and ML is imbuing it every single step of the way: “There’s not one big problem that needs to be solved. They’re hammers, and every single problem requires a hammer suitable to it. Perhaps ML is the hammer, but it might not be,” he said.
Future excitement
Cambrium’s ‘excitement’, meanwhile, is over the natural energy between proteins and natural language processing (NLP). Proteins, Salvy explained, are a string of letters and all progress through the NLP vertical can be mapped into ML. At the time of the roundtable, Cambrium were working with NVIDIA on a programme language model: “Out of a mathematical equation, you get proteins. Out of nowhere, [they] will materialise. It is absolutely Sci-Fi, but it’s actually working,” he said.
On the subject of hype when it comes to these technologies, Field cautioned that “there is clearly a bubble in generative AI,” but that the onus is on them to “temper this with reality.”