Leveraging augmented analytics to drive better business decisions
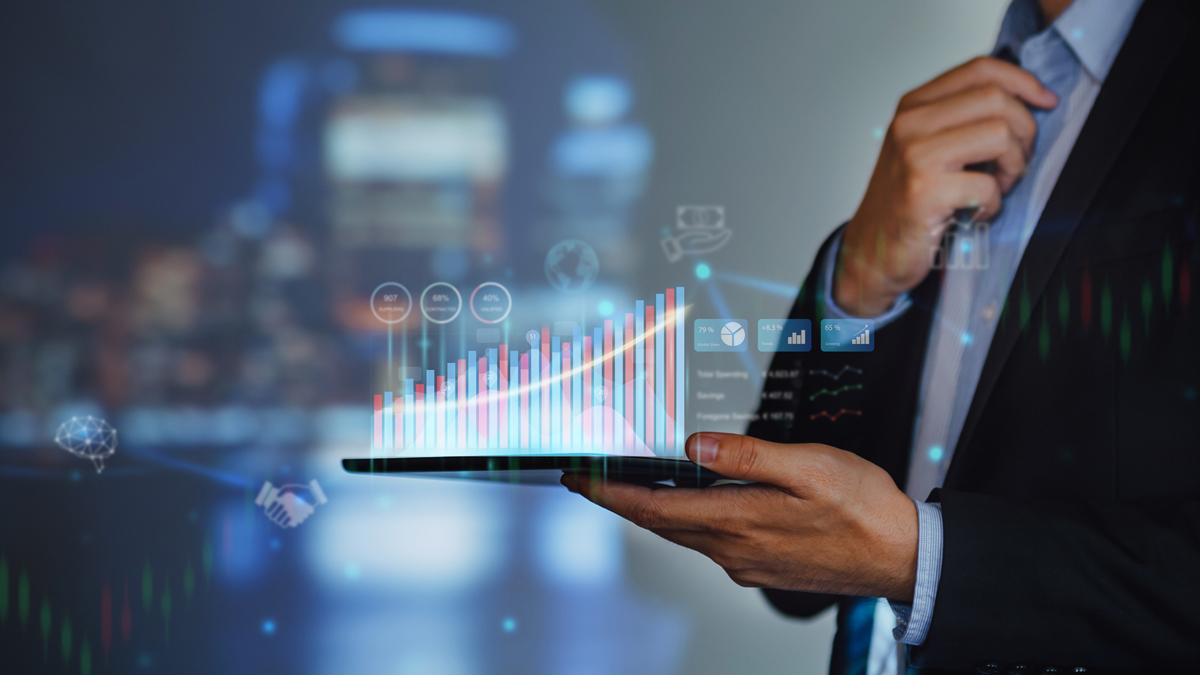
In early 2020, health officials were cautious about forecasting a COVID-19 vaccine timeline to the public, as vaccine discovery was a historically laborious process that took years (if not decades). Yet a successful COVID-19 vaccine gained emergency approval in just 11 months, a blistering pace that likely saved millions of lives. Such a fast turnaround would not have been possible without advanced analytics and the troves of intelligence that scientists had at their disposal.
Despite the success tied to the vaccine rollout, analysing data is still a daily challenge for many life sciences and pharmaceutical companies. There is an abundance of data that is ready and accessible, but without tools and processes to make sense of it all, decision-making becomes inefficient and inaccurate. This is where augmented analytics play a crucial role in supporting the vast amount of data that is available to these companies.
Augmented analytics can address many of these inefficiencies by automating key analytic processes and exploring millions of combinations that a human simply cannot do. Most importantly, they empower business analysts and data analysts to perform analyses without the need for advanced data scientists to be involved, which not only makes the process faster, but increases efficiency around seeing business impact with less skilled resources.
According to McKinsey, advanced analytics enabled by augmented analytics can broadly accelerate business impact, estimating the attainable operating efficiency from scaling these tools to be as high as 15% to 30% of earnings over five years, growing to 45% to 70% over a decade.
This makes tools like augmented analytics essential to helping pharmaceutical and life sciences companies dig deeper into their data. Most significantly, these tools provide the ability to ask the important “why” and “how” questions to obtain better insights on important trends, such as why certain data points change (or don’t change) and the factors contributing to those shifts.
There are many use cases of augmented analytics in the pharmaceutical and life sciences space, but three that are directly impacting decision-making strategies include analysing prescriber behaviour and trends, discovering patterns in data, and gaining better visibility into supply chain performance.
Analyse prescriber behaviour and pinpoint underperforming healthcare professionals
By analysing prescription data across multiple sources, augmented analytics can look at the medical conditions, patient demographics, and specialty areas for which drugs are most widely prescribed.
One example of this use case is a pharmaceutical company that recently gained FDA approval for a rare cancer treatment. The treatment was only eligible to patients once they had undergone three to four rounds of other treatment. Spotting eligible patients – or rather, spotting healthcare professionals (HCPs) who would consider prescribing patients this new drug – was a needle-in-a-haystack proposition. The pharmaceutical company’s limited salesforce couldn’t afford to indiscriminately cold call HCPs, and they needed a targeted approach.
This company used augmented analytics to merge internal CRM data with third-party patient claims data to find similar classes of oncology drugs. They used that data to build and train a machine learning-based HCP targeting model to predict which accounts were most likely to become prescribers. This in turn provided them the focus they needed to conduct their sales outreach. Significantly, the work was completed by business analysts on their existing data teams – not by advanced data scientists – and helped the firm identify 30% additional new opportunities, all without adding sales staff.
Analysis frameworks provided by augmented analytics can help companies protect market share gains by adjusting for gaps in coverage. In use cases like the one above, companies can help grow market share by overlaying HCPs against sales coverage, which can be used to target underperforming HCPs.
Discover patient patterns to improve care
Augmented analytics allows pharmaceutical and life sciences companies to understand the “why” behind certain outcomes. It also uncovers the primary factors impacting patient satisfaction to identify gaps in patient care.
As Deloitte points out, augmented analytics can be used on large population studies; geographic, demographic, and medical condition information can generate profiles of community health patterns and target the demand for prescriptions more accurately.
It can also be used by a market access team at a large pharmaceutical company to understand how a particular treatment impacts outcomes, and how a CPT code tied to the company’s testing associates with fewer number visits. For example, whether there’s a reduction in additional diagnostic testing because of the use of a particular CPT code, or whether diagnosis codes are associated with more successful payment.
By using machine learning insights that automatically evaluate every combination of data points across a firm’s internal and external data sources, these firms can spot key trend drivers and hidden relationships between variables.
Increase supply chain performance visibility
Pharmaceutical and life science firms are turning to augmented analytics to help them increase equipment reliability, prevent manufacturing losses, and spot underutilised plant capacity. They then adjust accordingly and improve yield, quality, and cycle time.
Operations are optimised through AI-driven analytics, which increase visibility into the supply chain’s performance. Asset performance management tools create efficiencies in the supply chain, while predictive systems can analyse failure patterns and warn of imminent equipment failures. This reduces manufacturing costs and presents savings opportunities across all stages of the product life cycle. As a result, capital expenditures (CAPEX) are reduced.
Augmented analytics is imperative to commercial success
In today’s increasingly digital world, pharmaceutical and life sciences companies will only continue to find themselves awash in more and more data. How they leverage and scale analytics to parse that data will inform the quality of their decision-making and their ability to keep up with industry peers.
To maintain pace, they must employ a modern tech stack that allows for easy and quick analysis of all the intelligence they generate and capture. By leveraging augmented analytics, pharmaceutical and life science firms can not only upskill existing data analyst teams to uncover relevant business insights.
This relieves data scientists so they can focus on more advanced analysis and model building and also helps them to quickly get the type of “how” and “why” insights that companies in this space need in order to make sharper decisions that drive business performance.
About the author
Ajay Khanna is CEO and founder of Tellius, a company disrupting business analytics with Search and AI, is a tech entrepreneur who has a passion for building disruptive enterprise products with an awesome user experience.
Prior to starting Tellius, Khanna was CTO & founding member of Celcite, a fast-growing telecom analytics and solutions company that was acquired by Amdocs. Khanna has over 25 years of extensive experience working in various technical, business. and consulting roles. He holds degree in Electronics and Communications Engineering.