Digital health round up: UK government invests millions in AI
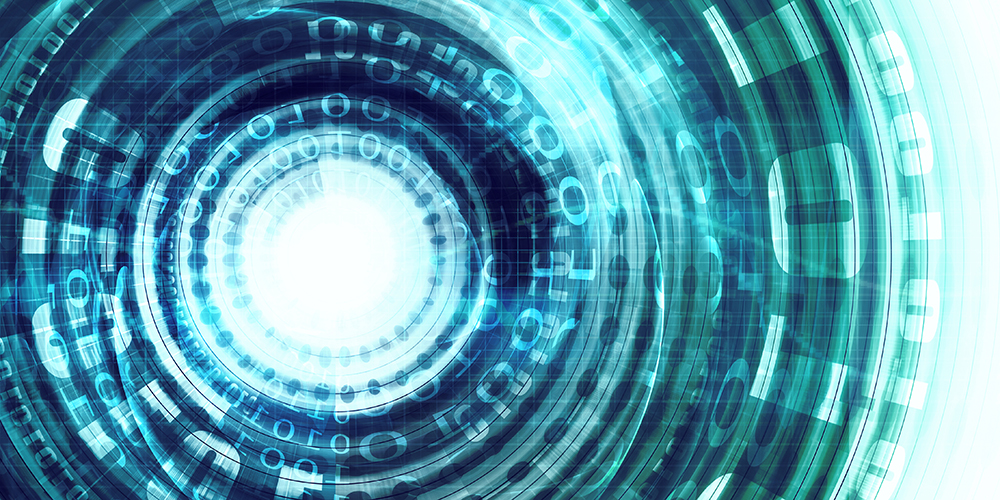
This week saw the announcement of millions of pounds of investment from the UK government into artificial intelligence (AI) health projects, with five centres across the country focusing on pathology, diagnosis and imaging.
Backed by £50m in funding, the new centres will bring together doctors, businesses and academics to develop products using AI to improve early diagnosis of diseases including cancer.
Products developed at the new centres aim to offer more personalised treatment for patients, while freeing up doctors to spend more time on caring for patients.
According to the announcement from the government departments for health and business, the investment in large-scale genomics and image analysis will drive new understanding of how complex diseases develop, in a proactive step to ensure people get the right treatment at the right time.
Centres will be based in Leeds, Oxford, Coventry, Glasgow and London.
The centres will be funded through the Industrial Strategy Challenge Fund, the government’s investment programme that focuses on addressing the opportunities and challenges of the future, which is managed by UK Research and Innovation.
Centres will be spearheaded by medical companies including GE Healthcare, Siemens, Philips, Leica, Canon and Roche Diagnostics.
The centres are:
London Medical Imaging and Artificial Intelligence Centre for Value-Based Healthcare, which will use artificial intelligence in medical imaging and related clinical data for faster and earlier diagnosis, automating expensive and time-consuming manual reporting.
Glasgow’s iCAIRD (Industrial Centre for AI Research in Digital Diagnostics) will bring together clinicians, health planners, and industry to work with innovative SMEs to answer clinical questions, and solve healthcare challenges more quickly and efficiently.
NCIMI (National Consortium of Intelligent Medical Imaging) in Oxford will consider the role clinical imaging plays in the delivery of more personalised care and earlier diagnosis to support disease prevention and treatment.
Based in Coventry, the Pathology image data Lake for Analytics, Knowledge and Education (PathLAKE) will use NHS pathology data to drive economic growth in health-related AI.
As already reported by pharmaphorum, The Northern Pathology Imaging Collaborative (NPIC) located in Leeds will boost the city’s capacity in digital pathology research.
Public health officials commission healthy living app
Public Health England is calling on tech firms to develop an app that encourages people to live healthier lifestyles.
In a contract notice issued late last month, the agency responsible for the nation’s health and wellbeing said it has a budget of £2.2 million earmarked for the proof of concept project.
According to the notice the project will be delivered by mid-2019, and will assess one or more ways to support people to adopt healthier behaviours.
Public Health England said it has built a team of national and regional experts in areas such as health economics and behavioural science to support the winning bid.
Applicants must have experience of using mobile apps to support people adopting healthier behaviours within the last three years.
They must also have demonstrable experience of working with commercial or public health partners in the delivery of digital intervention projects, and running proof of concept projects.
Work can be performed from any location but is likely to be coordinated by staff in Manchester, London and other areas in the UK.
Those applying are being asked to propose how they would devise a proof of concept that could be used in a region such as the North West or London, and the project will be based overall in the North West of England.
Applications close tomorrow, and PHE has said it already has 20 completed applications from 18 small and medium-sized companies and two large companies.
There were a further 53 incomplete applications from 44 SMEs and nine large companies, according to PHE’s contract notice.
Tool could make trials more efficient by reducing patients' stress
Tech firm Cambridge Consultants has designed a digital health tool that aims to make clinical trials more efficient using machine learning to help prevent patients becoming stressed and dropping out.
The tool, known as Verum, remotely monitors patients and provides machine learning-driven predictions around conditions or disease states.
Verum’s initial test application focuses on clinical trials, where the company thinks huge efficiencies can be achieved by identifying and mitigating the effect of patient stress on trial outcomes.
Stress is an underlying cause of behavioural and disease states and yet it is poorly understood, leading to badly controlled clinical trials with average drop-out rates at 30%.
For proof of concept, Cambridge Consultants designed a system to measure and monitor a participant’s stress levels during trials.
Developed in consultation with clinical psychiatrists and neuroscientists, Verum uses the power of biometric data, primarily voice and electromyography (EMG), and machine learning to better understand outcomes and increase the likelihood of clinical success.
It said the machine learning-driven system can provide deeper insight on participants during trials through real-time triggers and alerts, enabling clinicians, nurses and trial co-ordinators to mitigate the effect of stress on compliance, investigate stress as a confounding factor and inform better adaptive trial design through continuous data streams.
With the average cost of a phase 2 clinical trial ranging from $7 million to $19.6 million and 80% of all clinical trials failing to reach completion, the company noted that increased efficiency has the potential to deliver very significant savings.
Verum comprises sensors integrated into a wearable, a data collection app and a widget for healthcare dashboards that augments existing data with patient-specific predictions and alerts, for unprecedented clinical insight.
The tool aims to help clinicians, nurses and trial co-ordinators to access biometric and behavioural data from continuously monitored patients, gaining valuable context around a specific condition.
This is achieved by applying a machine learning algorithm to measure patient data, producing a single numerical estimator of a patient’s stress levels at any stage of the trial.