Enhancing clinical trials: The case for algorithmic prespecification in subgroup analysis
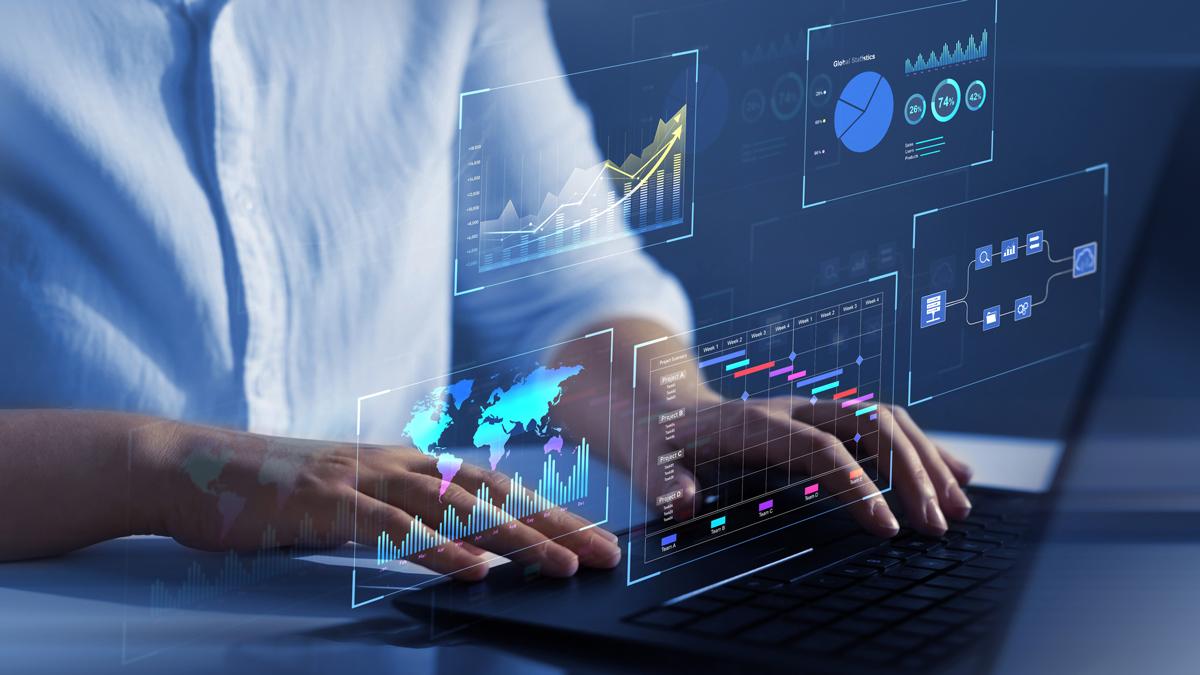
In the dynamic world of clinical trials, innovative methods are vital to improving the efficiency and reliability of medical research. One area that has garnered both interest and scepticism is subgroup analysis.
Subgroup analysis involves breaking down a study population into smaller populations according to specific characteristics such as age, gender, genetic markers, or severity of a condition, and analysing the data of each group separately. This can provide important insights into potential differences in treatment effects or outcomes within specific populations.
While this practice is widespread in clinical trials, the lack of a standardised approach raises concerns about the credibility and reproducibility of results. The need for a paradigm shift is evident, and in this piece we will explore the potential of algorithmic predefinition in subgroup analysis as a means to address these challenges.
“Always Do Subgroup Analysis, But Never Believe Them" - Sir Richard Peto
Indeed, it's common to encounter scepticism when discussing subgroup analysis. The prevalence of this scepticism is captured by a memorable peer-reviewed paper published in The Lancet that humorously explored sub analyses according to zodiac signs, highlighting the inherent risk of finding false associations when searching through vast datasets.
The criticism of subgroup analysis often stems from the perception that it can be manipulated to yield favourable outcomes, leading to a lack of trust in the reliability of the findings.
A call for change: Bridging adaptive trials and subgroup analysis
The clinical trial landscape is gradually undergoing a shift from traditional, fixed protocols to more flexible, adaptive designs. Adaptive trials allow modifications to the trial after its initiation without undermining its validity and integrity.
While the shift to adaptive clinical trials may also draw potential scepticism, the creation of well-defined guidance and a process of algorithm pre-specification has helped overcome this challenge.
This guidance, published in 2019 by the US Food and Drug Administration (FDA), outlines key principles for adaptive design, simulations and changes, regulatory considerations, and maintaining trial integrity.
Drawing inspiration from this trend, the industry would be wise to consider a similar evolution and guiding framework for subgroup analysis.
Consider the following:
- Hope for the best, prepare for the worst
In the world of clinical trials, failure isn’t the exception, it's the norm. It is often difficult to prepare for less than successful results, but ignoring the possibility of failure is not the solution. Ironically, it is only by preparing for the possibility of potential negative outcomes that it is possible to increase the chance of success. - Overcoming scepticism through transparency
The scepticism surrounding subgroup analysis often arises from concerns about post hoc exploration and selective reporting. Algorithmic predefinition addresses these concerns by introducing transparency and predefined logic into the process. If the decision-making criteria are established before the trial commences, the likelihood of false associations and discoveries diminishes significantly. This not only enhances the credibility of the findings, but also instils confidence in the scientific community and regulatory bodies. - Connecting adaptive trials with subgroup analysis
The combination of adaptive trials and subgroup analysis is a logical progression that can produce substantial benefits. Adaptive trials allow for dynamic modifications in response to accumulating data, enhancing a trial's overall efficiency. Similarly, by predefining algorithms for subgroup analysis, researchers can establish a structured framework for decision-making within the trial. This framework can be examined and discussed before the trial begins and its operating characteristics can be better understood. This synergy enables a more cohesive and harmonised approach to clinical research, bridging the gap between trial design and subgroup exploration. - Embracing novel approaches
Combining the above points, we must recognise the unique opportunities that algorithmic predefinition brings to subgroup analysis. By creating algorithms that dictate decision-making during a trial, we empower researchers to leverage data without inflating the risk of Type 1 errors. Algorithmic predefinition encourages a shift in mindset, promoting the use of stronger tools for more meaningful insights.
Towards a logical algorithm: Defining the future of subgroup analysis
In essence, the proposition is to define a logical algorithm before initiating a trial, outlining how subgroup analysis decisions will be made throughout the study. For instance, the algorithm could dictate that, if a result surpasses a certain threshold, a specific decision or action will follow. By establishing this predetermined logic (taking multiple-hypothesis testing into account), researchers can navigate the complexities of subgroup analysis with a clear roadmap, mitigating the risk of unwarranted findings.
This predefinition naturally extends to the validation process itself - how will the validation be conducted? What tests should be run? What data should be left as a holdout, and more.
Additionally, moving towards pre-defined subgroup analysis - leaning heavily on the adaptive design framework and guidance - can allow for subgroup detection during the trial itself (using adaptive enrichment design). This can enable more strategic identification and exploration of subgroups during the trial, avoiding the need to first “fail”.
In conclusion, the integration of algorithmic predefinition into subgroup analysis represents a paradigm shift that aligns with the dynamic nature of modern clinical trials. Embracing this approach not only allows for the exploration of novel and meaningful discoveries, but also addresses the scepticism surrounding subgroup analysis.
By fostering transparency, connecting with adaptive trial methodologies, and leveraging the power of predefined algorithms, we pave the way for a more efficient trial process. In today's era of clinical research and innovation, evolving our methodologies is crucial to ensure the integrity and credibility of our efforts in delivering essential therapies globally.