What Are IDEAs Made Of: Prediction of risk
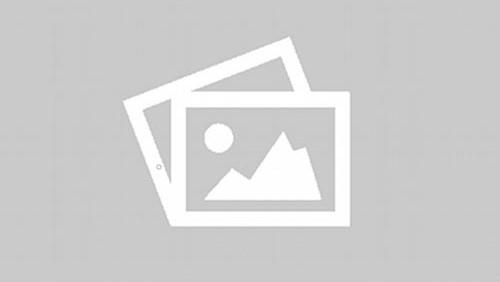
Mike Rea
IDEA Pharma
Hearing that there is a 24% chance of rain tomorrow, would you carry an umbrella or not? How about if it was 24.1%? Rain is reasonably binary (it is raining, or it is not...unless it is that drizzly, 'is it raining?' kind!), and once you're in it, the chance of rain is 100%.
In pharma, the estimation of risks has gone from the finger in the air 'feels like rain' to the 24%, but without the aid of the weather forecasters' supercomputers or the benefit of continually checking the accuracy of those predictions. In one meeting recently, we were told that a submission had a 24.1% chance of succeeding, which brought a smile…Think about it: you won't get a decimal place estimation of risk without a mathematical model. And to get a mathematical model, you have to include all the parameters you think are important in the decision, and work out a formula to produce your final number. But here, a group of people were estimating the chances of another group of people approving or not approving their drug.
What 24.1% says to us is 'more likely than not to not be approved, but we don't have the confidence to say it has zero chance.' (As I know you will be asking, no confidence interval was given - one suspects it would stretch to the 50% mark, at which they're saying 'your guess is as good as mine…'). The '24' is important, because it sounds like a more educated guess than 20% or 30%, while not differing in any meaningful way from either number. The 'point one' is just a step too far, while it sounds incredibly accurate, the reality is that, for the more informed recipient, it means a heavy reliance on a model which is probably wrong.
In fields where forecasting is important, it is well known that there is a paradox - the more complex the model, the more likely it is to be wholly wrong sometimes. Weather forecasting is insignificantly better at one-week predictions than it used to be before supercomputers and billions of research dollars. Stock market prediction is the same. So, here, we have a situation where someone has tried to model the risk of submission failure, included several parameters that are likely to be predictive (not too many though, as that ends up being hard work), asked several people their best/ informed guesses about each of the parameters, taken an average of each of those, and then applied a formula to one decimal place. So, roughly right, possibly...
"In fields where forecasting is important, it is well known that there is a paradox - the more complex the model, the more likely it is to be wholly wrong sometimes."
However, knowing that 24.1% is of no more value than 'unlikely' is not the point. The point is that 'unlikely' may well be more accurate and more reliable, because unless you know that the model is validated, you may well place too much reliance on its accuracy. As a way of summarising a group's opinion, 'unlikely' is probably a more accurate, if less scientific-sounding, outcome. (At this point, it is critical to restate that a group's collective opinion, in the classic Surowiecki sense, is way more valuable than any one individual's). The excellent book, How Risky Is It, Really?: Why Our Fears Don't Always Match the Facts, by David Ropeiki, reminds us of the problems of head or heart assessment, arguing that we take affective views of risk.
So, the open question: is prediction important? Answer: massively. Knowing that a submission is unlikely to succeed forces a team to plan for failure (which was why we were there). Knowing why is also important, as it is critical for that planning.
There are three main areas in pre-launch that require prediction, each of them interdependent/ interrelated. Technical probability of success, regulatory probability of success, and commercial probability of success (this last is conveniently ignored by most companies in the industry). The first two are often underdone and poorly scrutinised. (Let's agree that 'risk' is the inverse of 'probability of success' for ease of conversation.) At one Top 10, they apply industry attrition levels to their phase III programme as part of the calculation of technical risk, despite individually having had 100% pIII attrition for the 5 years to 2009 (whereas another Top 10 had 0% pIII attrition in those same years). Which number is more likely to apply - the industry's rate or the company's own rate?
Technical risk has some good hard inputs - prior science, levels of effect, proofs of concept and principle, statistical powering. But lowering technical risk has the side effect of increasing regulatory and commercial risk. So, technical risk should be done on a set of strategic options, not on a single study. (Recently, we saw a huge 5 year, several thousand patient pIII study about to get a green light, despite having a technical probability of success of 50% - that same couple of hundred million could as happily have been placed on Red in Monte Carlo).
"Regulatory probability of success, despite having to take into account humans and their biases and prejudices, should be easier to predict..."
Regulatory probability of success, despite having to take into account humans and their biases and prejudices, should be easier to predict, but it is important to do this assessment before the lock down of phase III, as those tinkering with the technical risk will make a massive difference here - would you prefer to run a study that succeeds that doesn't achieve registration, or run a study that doesn't hit its primary endpoints?
Predicting probability of commercial success is the industry's new goal: launching drugs that repay their investment and make a contribution. The elephant in the room for the industry, while only 1 drug in 4 repays its investment, commercial success has been the whipping boy of the industry while companies have sought launches at any cost, massaging technical and regulatory risk to lowest levels, producing products with no differentiation, convincing data or place in therapy. So, with an average 25% chance of rain tomorrow (almost 24.1%…), how happy should the industry be to keep telling its investors and shareholders that they should expect rain? (Or, like Alice, be told that it is always jam, tomorrow?)
Here's the thing: it has been said that plans are useless, but that planning is essential. Predicting risk is only useful if something is done about it, if it is used as a tool before setting off. Knowing that you are on a plane that has a 25% chance of reaching its destination would be rather discomforting if you only find out once you've set off.
Pharma is a risk business, but balancing the key risks can produce better outcomes by enabling planning and mitigation.
About the author:
Mike Rea is a Principal with IDEA Pharma, who enjoys taking a look outside the industry to learn how it can think differently. For direct enquiries he can be contacted on mike.rea@ideapharma.com and for more information on IDEA Pharma please see http://www.ideapharma.com/what/default.htm.
The next WAIMO piece will be in a couple of weeks.
Do precise risk calculations serve any real purpose?