The trouble with expert judgment in forecasts
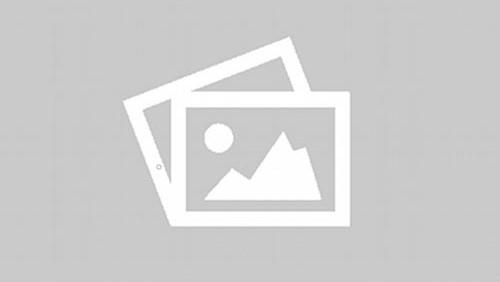
Gary Johnson
Inpharmation
Most pharma “forecasting models” are not actually forecasting models. To work, they require that all the interesting-and-hard to predict numbers (like peak market share, market size, price etc.) be inputted by the user. A model that demands that the user inputs all the interesting-and-hard to predict numbers is not a forecasting model. It is simply a calculator pretending to be a forecasting model. The model simply applies some arithmetic to judgments provided by the user and with great show (often involving clever Excel formatting) comes up with the “forecast”.
So what is the problem? The problem is that these models look so sophisticated (and often cost so much) that people think they have a state-of-the-art forecasting model. The fact that everything hinges on a set of judgments input by the user is obscured. The style of the forecasting model can lend credence to a set of judgments that have no substance. And the judgments usually will have little substance because judgment (even “expert” judgment) has been proven in countless studies to be a fundamentally inferior way to forecast.¹
Inferior to what? Study after study has shown that judgment is inferior to real forecasting models. A real forecasting model is a model that looks at past data and finds patterns in those data. For example, if we look at the market shares achieved by brands that have been launched in the past, and also look at each brand’s entry-order (was it the first to launch in its class, the second, third etc.?), then we will see a clear pattern. The pattern is that, on average, early-to-market brands tend to achieve higher market shares than late-to-market brands. (Yes, we all know about Lipitor! This is forecasting and there are exceptions to every general trend. As the saying goes: “The battle does not always go to the strongest, nor the race to the swiftest, but that’s the way to bet.”)
"Study after study has shown that judgment is inferior to real forecasting models."
A proper forecasting model does not recognise such a pattern in a vague, qualitative way, it quantifies it precisely. In the case of predicting brand market shares from market entry orders, we can use a form of Zipf’s Law. This fascinating law was first discovered in the 1940’s by a linguistics professor at Harvard University, George Kingsley Zipf. Zipf decided to count how often words occurred in the novel Ulysses. To his surprise and delight, he found a simple pattern. The most common word (“the”) occurred about twice as often as the second most common word (“of”), three times as often as the third (“to”), four times as often as the fourth (“and”), five times as often as the fifth (“a”). And so on.
Zipf’s Law has been found to apply to an amazingly large number of phenomena. For example, the size of cities. Hits on web pages. The sizes of earthquakes. And many, many more. Importantly for us, a modified form of Zipf’s Law predicts the relationship between entry order and market share for pharmaceutical brands. Zipf’s Law would say that the first brand to launch ends up with twice the market share of the second brand to launch, three times the market share of the third brand to launch and so on. It turns out that Zipf’s Law is a little too aggressive and punishes late entry brands too much. A small tweak to the maths results in a formula that links entry order to average market share very closely.
Of course, entry order is just one of the factors that determine a brand’s sales. (It would be foolhardy to use an entry-order model on its own unless entry order was the only information you had about a future brand.) Fortunately, there are simple models that can be used to predict all the important determinants of a brand’s sales: proportion of patients treated, therapy class shares, impact of profile and advertising spend on market share, price that payers will tolerate and so on.²
"Fortunately, there are simple models that can be used to predict all the important determinants of a brand’s sales"
So, given that we have effective forecasting models to predict all the key determinants of a brand’s future sales, what role, if any, does that leave for expert judgment? The answer is: very little. A review of the empirical literature shows clearly that judgment works best when there is little change ahead and when we have little objective data available with which to “feed” a forecasting model.² The pharma industry is the best-audited industry on the planet. We almost always have – or can get – plenty of data. Any important forecast almost invariably involves change (often the launch of the product we are forecasting!). So, judgment-based forecasts are rarely “indicated” in pharmaceutical forecasting.
Any yet, there is one very important role for judgment. Although expert judgment is not good at forecasting actual numbers, it is good at identifying the key factors that will affect the numbers. So, say, for example, we are interested in the price that a new, breakthrough pharmaceutical could command. Pricing experts will be poor guides to the price that we could charge. However, if we tell them that this product treats a disease that afflicts a very small number of people, they will be able to tell us that novel, effective pharmaceuticals that target small patient populations command much higher prices than comparable drugs that target much larger populations.³ But, if you ask them “How much higher?” they will not be able to quantify that insight. And that is where a model comes in. A model will “look at” thousands of payer decisions and find the statistical relationship (i.e. the pattern) between patient numbers and price. (An effective price forecasting model will also have to look at other key drivers of price, of course.)
"What role, if any, does that leave for expert judgment? The answer is: very little."
This, then, is the role of expert judgment in forecasting: to suggest the “vital few” factors that drive the price, the market share, the market size etc., leaving it to real forecasting models (not calculators) to quantify the impact of these drivers.
A vast scientific literature attests to the greater accuracy of forecasting models over expert judgment. It is ironic that the pharma industry is supremely science-based when it comes to the conduct of, say, clinical trials. Yet, when it comes to forecasting, relies on finger-in-the-air judgments disguised within calculators-posing-as-forecasting-models. Were the pharma industry to apply the same levels of scientific evidence to its forecasts that it does to its R&,D, the role of judgement in forecasting would largely disappear.
References:
1. Grove WM, Zald D, H., Lebow BS, Snitz BE, Nelson C. Clinical Versus Mechanical Prediction: A Meta-Analysis. Psychological Assessment 2000,12(1):19-30.
2. Johnson G. Sales Forecasting for Pharmaceuticals: An Evidence Based Approach. Henley on Thames: London Scientific Publishing, 2005.
3. Johnson G. Principles of Pharmceutical Pricing: An Evidence Based Approach. Henley on Thames: London Scientific Publishing, 2008.
About the author:
Gary Johnson is author of Sales Forecasting for Pharmaceuticals: An Evidence Based Approach. His company provides modelling software and consultancy to all the world’s leading pharma companies. Gary has been short listed for the MCA Business Book of the Year Awards and has won numerous best paper and speaking awards.
Email: gary@inpharmation.co.uk web : www.inpharmation.co.uk
What influences people to opt for judgment over forecasting models?