Expanding data sources: faster drug development?
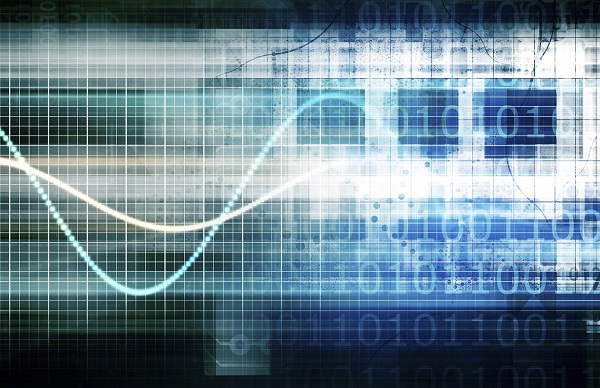
Using advanced technology and the skills of the data scientist for data source management from diverse sources can bring many benefits to the speed and quality of clinical trials, says Jim Streeter.
It’s logical to think that expanding data sources in clinical trials can lead to more therapies, but does this additional data speed up or slow down a clinical trial?
In the past, clinical trials used only structured, clinically-sourced data, which was relatively easy to organise and mine, but today they are more complex and utilise data from a plethora of sources, such as mHealth devices for remote monitoring of trial patients, mobile health applications, biomarker data and clinically-sourced data.
If the end goal is getting more therapies to market, and currently only 20-30% of drugs make it that far, can expanding data sources and improving the aggregation and management of both unstructured and structured data help improve the likelihood of regulatory approval and getting safe and effective drugs into the hands of patients? The answer to this question is ‘yes’, using a combination of advanced technology and the skills of the data scientist.
The technology
If larger and more comprehensive datasets are made available to pharmaceutical companies, and their clinical researchers, questions about what data to collect and how to use it are replaced by more meaningful ones like: ‘What new theory can I prove regarding the population of this trial?’; ‘Should my study continue based on new insights I’ve received?’ or ‘What new patterns can I uncover to lead me to a new hypothesis?’
The answers lie in technology – technology with advanced metadata management capabilities that can offer the flexibility and scalability needed to handle all real-world data in the format, size, and frequency required as clinical trials evolve.
Today, the amount of data from various domains is exploding, giving a more comprehensive picture of clinical studies that can, in turn, improve decision making. There are challenges in aggregating, storing and preparing the data for high-speed analysis because it is no longer just structured data. It is both structured and unstructured data, from a growing number of systems, devices and publications, such as electronic medical records (EMRs), medical devices and documented research. This influx, while valuable, is making it difficult to capture and analyse at different intervals during a study.
For example, consider a biopharmaceutical company focused on cancer drug development. To improve its study and time to market, the study teams want to be able to quickly combine and analyse data collected through the clinical trial, along with genomics data, to understand whether the patients in the trial are the right ones, based on their genes and likelihood of responding to the treatment. Armed with this information early on in the process, they can determine if they are on the right track or if they need to course correct with more precise patients. This correlation between the study and genomics helps identify the exact type of patient who has a better chance of responding positively to the drug. It is a big step towards precision medicine.
The prospect of combining multiple data types for better outcomes may seem time consuming, daunting and not worth the additional effort, as it could slow down the time to market, but combining these invaluable data types and making that information available throughout a study increases the probability of a drug reaching the market faster.
Technological advances and data scientists together are providing new levels of analysis and the ability to predict outcomes in near real-time. Machine learning and artificial intelligence are bringing new algorithmic techniques that can assist in identifying patterns that support enhanced and automated decision making along the drug development path. For example, historical data about a clinical trial’s ability to recruit suitable patients can predict the probability of future recruitment success. The combination of qualitative and descriptive data means researchers can identify similar groups of patients who are best suited to a new trial. This not only shortens the time taken in site selection and patient recruitment, but increases the likelihood of positive results.
Historically, the gap between combining structured and unstructured data for clinical trial decision making has caused errors and delays in setting up and completing successful trials. However, the new aggregation, storage and analysis solutions, combined with artificial intelligence and machine learning, means trends and negative signals can be highlighted much earlier in clinical trials. Not only does this shorten the path to drug development and improve safety, but it helps create a more robust pipeline of disease-treating therapies at a faster pace than ever before.
The human touch
However, the human touch is still critical and the role of the data scientist, or data revolutionist, has emerged. While clinical researchers focus on creating successful trials, the data scientist focuses on directing overall clinical data quality and management activities to support the progression of the drug development pipeline. This role has responsibility for connecting the dots across the organisation to ensure the right data reaches the right hands at the right time. While the role varies, based on each company’s needs, ultimately it means being a leader in both science and complex data management, quality and visualisation, from source to submission.
Blending technological innovations and data scientists’ expertise, and applying them to the clinical world, presents a huge opportunity for positive impact. Not only does the insight from more data from different sources enhance the questions asked of clinical trials and fill a much-needed pipeline of new drugs, it also uncovers hidden relationships that can precipitate new hypotheses and provoke new, potentially life-saving, questions that we never thought to ask before. With the right technology and people, new sources of data promise to speed, not slow, clinical trials allowing biopharmaceuticals to bring more life-saving drugs to market faster.
About the author:
Jim Streeter is global vice president Life Sciences Strategy for Oracle Health Sciences. He has over 25 years of data acquisition and analysis experience using computerised systems, and has focused on eClinical systems and processes for trials for the last 15 years.
He has implemented end-to-end eClinical solutions and processes across all therapeutic areas and all phases of studies and his early experience was gained at Pfizer, where he was senior director of Global Clinical Data Services.