The steps for successful AI
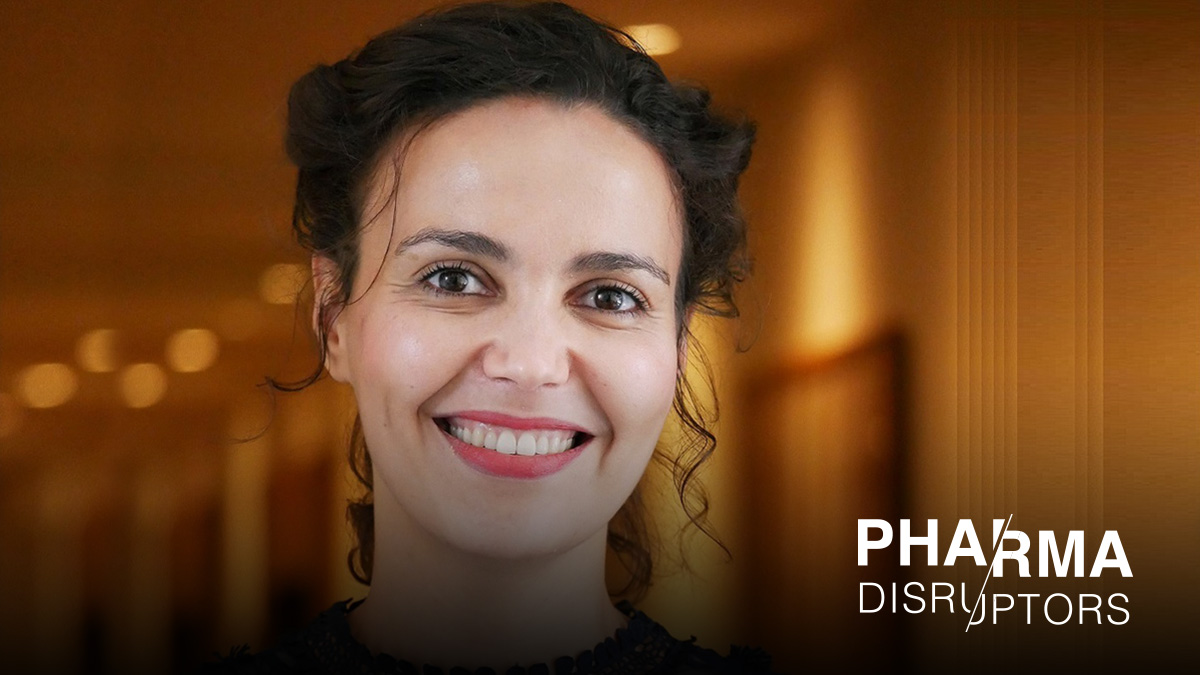
We speak to machine learning expert Loubna Bouarfa about getting life sciences to embrace AI and what makes for a successful use of the technology.
As a machine learning scientist, Loubna Bouarfa has found herself at the forefront of one of the biggest technology shifts in recent memory, with AI poised to leave no industry untouched – life sciences included.
Bouarfa did her PhD in implementing machine learning into operating rooms – through tracking surgeons and detecting anomalies and errors in real time during surgery. Since then she has become CEO of healthcare AI company Okra, and has been named as an Innovator Under 35 for 2017 by MIT and as one of Forbes’ Top 50 Women in Technology.
She says the main drive behind turning to entrepreneurship and applying her expertise to life sciences was her desire to optimise treatment for patients.
“Many patients out there are in need of treatments that they are not getting,” she says. “Many of them are taking pills that are not the best treatments for them because clinical trials only look at the average of the population.
“If we use AI to make sure drugs only get prescribed to the right patient, then we can take precision medicine to a very, very high level. At the moment the research we do doesn't really mean anything for patients. It’s mostly just used to help us get access to a country via a regulator.
“I don't believe that other stakeholders will do this, but life sciences has the scale to achieve it. The industry needs to reshape and take responsibility for driving drugs to the right patients and not wait for healthcare providers to do it.”
At the moment, though, adoption of AI is lacking.
“Only 25% of the companies in Europe are in the uptake stage for AI,” says Bouarfa. “The rest may be talking about it, but they are not doing it. Despite the hype surrounding machine learning, only a few companies are using it. In life sciences, that percentage is even lower.
Bouarfa says that the hype surrounding AI can actually be detrimental to adoption.
“Often the real benefits of machine learning and AI are lost amongst the hype and are not reaching life sciences.
“Meanwhile, people get worried about the risks of AI because they hear about all the scandals that happen around technology failures and data problems. This is the other side of the hype, which can be quite scary for companies. They’d rather see someone else do it first before they do it.”
Introducing ethical guidelines around AI could help prevent these problems from arising and increase confidence in the technology.
Bouarfa is a member of the high-level expert group assigned by the European Commission to deliver ethical guidelines for AI.
“The European Commission wanted to increase trust and identify the areas where we need to put boundaries on AI,” she explains.
“We have delivered two documents so far, one of which showed how AI can be implemented ethically. Now we are in the piloting stage with companies to identify what can be regulated and what can be implemented as best practices.
“The guidelines are helping more wary customers to understand AI and what they need to do to make it ethical and safe – e.g. transparency of algorithms, explainability of the results, and bias control.”
That said, she notes that in life sciences there is already a good foundation for this kind of governance, with the industry already being adept at things like anonymisation of patient data and handling bias.
Tackling misconceptions
Another barrier to wider adoption are the misconceptions about what AI can actually do and how it should be used.
“The first misconception is the view of AI as a one-off – the idea that it's simply an advanced methodology that researchers can use in one study and not use again after they publish,” Bouarfa says. “That's not AI. AI is a system that is deployed in an organisation and is used continuously.”
She adds that the second common misconception is that these technologies are going to take people’s jobs.
“To ensure that AI reaches its full potential, we do need to realise that there are some aspects of jobs that will need to be automated. But AI will not replace humans; it will work more as a decision support system for healthcare.
“Whether you are someone in R&D using AI system to summarise the literature for you, a medical rep using a system to support you in talking to healthcare providers, or a doctor using AI to make diagnoses, you need to embrace the technology and shift your mindset from ‘this is taking my job’ to ‘this is supporting me’.”
Bouarfa says that the third misconception is that rule-based systems alone constitute AI.
“A system making decisions based on just a few parameters is not AI. That's an IT system, the kind of thing that’s been used since the 80s. AI combines these systems with an artificial process system that learns from the data.”
She adds that the best way to stop misconceptions spreading is by simply “telling the truth” about what AI can do.
Successful AI
Bouarfa says there are three important ingredients for “successful AI”.
“First of all, we need to define outcomes and have a problem for the programme to solve,” she says. “In healthcare, as I said, this is often about patients taking drugs that aren’t best for them.
“Secondly, we need to know the right AI right tool to use for each situation. We need to learn from the past, to predict the best strategy or the best outcome for each patient. Sometimes we don't even need to use AI – you could use a simple statistical model that you don't need to automate.
“The third thing is that AI needs to empower the system for the people working with it. Therefore you need to know the person you're empowering. Is it a health researcher? Is it a medical lab? Is it a doctor?”
Despite low adoption rates, Bouarfa says she has seen “great shifts” in the use of AI to clinically validate drugs.
“We’ve seen that it can reduce the importance of clinical trials by providing a great tool to get the right evidence from the real-world environment. I think that shift will be huge for the industry. It's almost a new paradigm of evidence-based medicine, moving from the obsession with clinical trials to a data-driven way of validating treatment.”
Though we haven’t yet reached a point where drugs developed with the help of AI systems are widely available, Loubna notes that this era is definitely on its way.
“It will take time but I see it happening. The drugs that they are coming are not standard drugs – they are targeting very, very specific patients. We can only do that using tools that are much more complex like machine learning. It needs to happen, but we need to be open to it and ready to collaborate for it to happen quickly.
“I hope that the needed mindset change will happen and we can move from talking about it to doing it – to really adopting AI and empowering the workforce to better direct healthcare to the right patient, and stop thinking about the fancy ideas that are not helping anyone.”