How data is critical to commercialising drugs for rare diseases
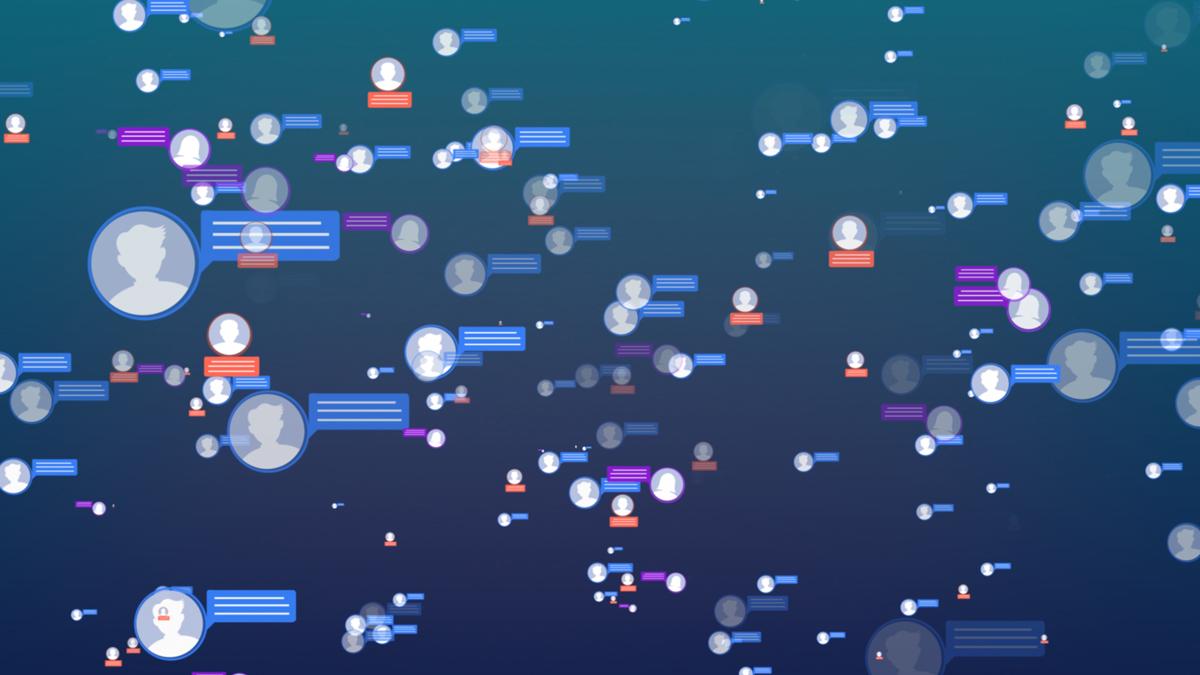
Orphan drugs are designed to treat rare diseases, but “rare” is a relative term. Each individual drug approved for an orphan disease could be relevant for as many as 200,000 people. Worldwide, some 300 million people are affected by more than 7,000 orphan diseases.
As with all drugs, development costs are high. But, compared to pharmaceuticals for more widespread conditions, the return on investment for orphan drugs is limited because, comparatively, there are fewer potential customers before long. That means it is more important than ever that, when a drug is commercialised, it reaches the right potential customers. AI-based systems can help companies accomplish this, and save money on highly targeted marketing and sales.
Although each drug may target relatively small numbers of patients, orphan drugs are a significant part of the pharmaceutical sector. Nearly half of all drugs now in development target orphan diseases. This is also a market badly in need of new drugs: data shows that one in ten Americans – some 30 million people – are affected by an orphan disease, with no treatment available for over 90% of these illnesses and conditions.
The expense of the orphan drug space
Many orphan drugs are developed, at least at the outset, by small pharma companies or start-ups (although bigger firms are beginning to get more involved as well). Whether big or small, though, companies that venture into the orphan drug space are taking on considerable expense. Development costs are similar to those for drugs for more widespread diseases, but, since the potential market is smaller, there is less opportunity to recover costs. That’s one of the reasons the median treatment cost was 17 times higher for orphan than non-orphan drugs. Part of this expense is allayed by the Orphan Drug Act, which in the US provides developers with tax credits of up to 50% of clinical development costs.
But, even with those credits, it can cost as much as upwards of $500 million to develop an orphan drug – a significant amount for even a large, established pharma firm, much less a start-up. Add to that commercialisation costs, and the bill goes up to far in excess of a billion dollars. These high stakes make it essential that companies get it right the first time – that development is done in the most successful manner possible, and that the process of commercialising a drug is as efficient as possible.
The latter is especially challenging; a Deloitte study shows that more than a third of newly- introduced drugs miss their sales expectations in their first year on the market. And some 70% of those drugs continue to miss expectations in subsequent years. The study finds that there are a host of factors that can cause these marketing and sales misses – among them, limited access to markets, a problem endemic to orphan drugs because of the difficulties in zeroing in on a very specific audience of patients and caregivers, along with an inadequate understanding of market needs, and poor product differentiation. Solving these issues is essential for drug companies seeking to introduce new products, especially in the orphan drug space.
The cost-cutting role of AI and machine learning
AI can play an important role here. The contributions of AI and machine learning – where algorithms review huge amounts of data seeking patterns that will indicate which molecules and elements are most effective in fighting a disease – are well known. But AI and machine learning also have a crucial role in commercialisation, and can help cut costs and increase chances of success significantly. This is especially important for companies developing orphan drugs. The Orphan Drug Act’s credits are for discovery and development; companies are largely on their own for commercialisation costs.
There are numerous ways AI can help with commercialisation. For example, AI systems can help marketing and sales teams discover target populations, including identifying healthcare providers and institutions that specialise in the specific diseases or conditions the drugs seek to treat. Advanced AI can even point drug companies to physicians treating individual patients with certain symptoms and conditions, based on anonymous patient level databases that track patient populations’ medical data (lab results, etc.).
Armed with that data, companies can more easily gauge commercial interest, determine sales margins, predict demand, and understand many other factors. Companies can also devise effective marketing campaigns, tailoring messages in a manner appropriate to the groups they are targeting based on this anonymous patient level data, engaging the right healthcare providers at the right time with the right information.
AI systems can also help determine the best channels through which to reach patients and caregivers with marketing and sales messages. Traditionally, companies would have had to do this work manually, taking months – if not longer – to gather and analyse the data and information. With AI and machine learning, they can accomplish these goals in a far shorter period of time – in a matter of hours instead of months.
AI and accessing the right HCPs, preventing churn
AI is also able to analyse more complex data, with many changing and moving parts, which is essential when it comes to understanding the needs of patients dealing with rare diseases and conditions. For example, AI-powered analysis can identify which patients undergoing certain types of treatment are most likely to experience side-effects and require a different course of treatment. Sales and marketing teams can then target the physicians overseeing these patients, offering the right treatments for the side-effects, and preventing churn. The AI-generated insights from this type of data are especially important when it comes to the ongoing marketing and sales of drugs, beyond their initial launch.
The best way for companies – especially smaller ones – to handle this AI analysis is by working with an AI platform designed for pharma commercialisation. Developing an in-house ability to research this data – much less having to find and hire personnel who can manage AI systems – is likely to be prohibitively expensive, raising the already high costs of commercialisation. And, alternatively, the common practice of relying on outside consulting firms to use their AI to undertake such analysis, often leaves drug companies with untimely insights, and too little control over the efforts.
Unlike with discovery and development costs, which end when the drug is ready for the market, commercialisation costs are ongoing. With advanced, high-quality AI systems, companies developing orphan drugs will be able to focus their efforts and cut these ongoing commercialisation costs significantly. Companies will also be able to dynamically and continuously adjust their sales and marketing strategy and tactics, as well as their messaging and targets, based on changing market needs. Hopefully those lower costs will leave more money to keep developing new innovative drugs, as well as encourage more companies to develop treatments for the thousands of rare diseases that currently have no treatment at all.