Patterns of behaviour: Using AI-based algorithms to improve outcomes for patients with atrial fibrillation
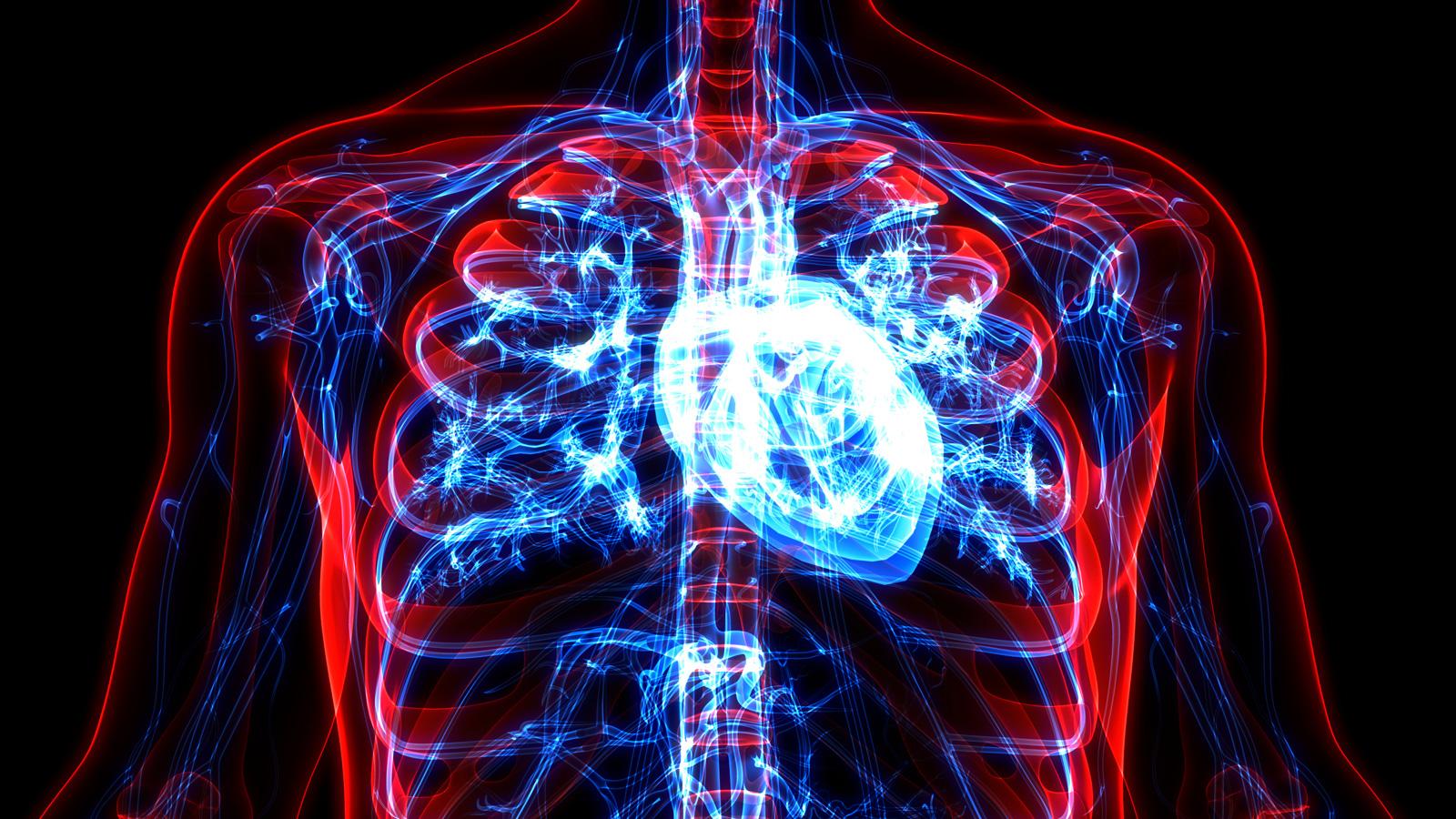
For those of us old enough to remember ringing in the year 2000, current concerns that artificial intelligence (AI) may usher in the end of the world as we know it may call to mind the fear that “Y2K” would similarly upend society. In both cases, legitimate concerns about technology acting unpredictably have led to doomsday scenarios that are unlikely to occur.
The concerns regarding AI are even less rational than those associated with what might happen when the computer clocks rolled over to the new millennium, given the many ways in which we use AI-based technologies in diverse aspects of our daily lives (mapping apps, digital assistants, e-commerce, etc.). The finance, agriculture, manufacturing, transportation, insurance, pharmaceutical, and medical device industries also use AI-enabled technologies to improve performance and simplify the user experience. In many of these examples, AI is used to recognise patterns that can then predict a desired outcome.
While AI offers a new and highly efficient way to identify patterns in very large and diverse data sets, the human brain has been doing this for millennia.
AI: Improving on the human
Medical device innovation offers an excellent example of how AI can improve on techniques that have been performed by human brains, eyes, and hands for decades.
For example, a radiologist or pathologist is taught during medical training how to identify cancerous cells or diseased tissue. With more and more experience, they can make these identifications more efficiently and accurately. AI-based radiology and pathology algorithms are similarly trained on very large data sets - many more than an individual physician will ever see in a lifetime - to recognise tissue of interest with greater speed and accuracy than even highly experienced practitioners. Such algorithms are easy to develop using existing images or other highly defined data sets.
However, developing AI models that need to integrate more diverse clinical information, including genetics, race, gender, age, and co-morbid factors can be more challenging. Recent advances in AI-based tools for identifying abnormal patterns of electrograms (EGMs) to aid physicians during cardiac ablation in the treatment of atrial fibrillation (AF), however, demonstrate that these challenges can be overcome, with the potential for improved patient outcomes.
Atrial fibrillation: The most common sustained cardiac arrhythmia in adults
AF, which is the most common sustained cardiac arrhythmia in adults, affects 37 million people worldwide1 and its prevalence is expected to double by 2026.2
Normally, the rhythmic contraction of the heart is regulated by a small region of tissue in the right atrium known as the sino-atrial node (SAN). Electrical impulses that form in the SAN are transmitted through the heart’s conduction system to both the left and right atria, causing the synchronised contraction of both atria and resulting in the simultaneous flow of blood from the atria to the left and right ventricles. In patients with AF, though, abnormal electrical impulses arise in the atria outside the SAN. This can cause asynchronous cardiac contractions that disrupt the normal flow of blood from the atria to the ventricles. Patients with AF experience rapid and irregular heart rhythms and the associated abnormal electrical signals can be recorded on an electrogram (EGM). These arrhythmic episodes can be brief (known as paroxysmal AF) or last for longer periods of time (persistent AF).
Current AF treatment paradigms include drug therapy, cardioversion (the use of drugs or electric shocks to restore normal heart rhythms), or a catheter ablation procedure in which the tissue from which the abnormal electrical impulse originates is destroyed.
Catheter ablation: An important treatment option for AF
Catheter ablation procedures are performed by interventional electrophysiologists, trained surgical specialists who perform procedures directly in the heart to diagnose and treat cardiac arrhythmias such as AF. During the procedure, the electrophysiologist introduces catheters into the heart through the veins. The catheters are used to measure electrical signals within the heart, which are then used to create a precise three-dimensional map of how these signals flow through the heart. This can identify areas within the heart that are driving arrhythmic activity. Elimination of these areas with radiofrequency, pulse field ablation or cryoablation can restore normal sinus rhythm and alleviate AF.
While this approach is conceptually straightforward, it may be significantly more complex in practice for several physiologic reasons, including the type of AF (paroxysmal vs persistent), location of the tissue triggering abnormal rhythms (within the pulmonary vein vs other areas of the heart), and the composition of the trigger tissue (scar tissue vs normal tissue). Additionally, other factors, such as gender, race, age, smoking status, and co-morbid conditions, appear to play a role in determining outcomes for patients with AF treated with catheter ablation.
Moreover, the existence of and interaction among multiple trigger regions can further complicate the selection of tissue for ablation. This complexity means that patient care and outcomes can vary based on the expertise of the treating electrophysiologist or the technology used at the facility where the procedure is performed.
AI for improvement, enabling standardisation
Electrophysiologists can select catheter ablation target areas by looking at specific features and patterns in a patient’s EGM. As with many other fields of medicine in which the ability to detect patterns effectively can improve outcomes, AI has the potential to more accurately identify regions of interests during catheter ablation procedures, especially in cases with multiple trigger points (known as dispersed EGMs) for which the interactions among multiple abnormal electrical signals need to be considered. Importantly, AI algorithms can be trained not only on EGM data, but on other relevant data sets noted above. This enables more tailored and personalised approaches to treating AF.
Rather than selecting ablation targets based solely on EGM data from a few trigger areas, AI-based systems can consider every trigger area, as well as the patient’s specific demographic factors, co-morbid conditions, and health history.
Promising results of this approach were demonstrated in the first large-scale clinical validation of a companion AI-based software solution (Volta Medical) that reproduces expert-physician EGM analysis and assists operators in the real-time identification of dispersed EGMs during ablation procedures in a persistent AF population. Results showed that the software solution enabled the construction of standardised maps that operators could use as reference to conduct EGM-guided assessment of the AF. The standardisation of the detection of abnormal EGMs resulted in uniform ablation strategies among patients and facilities, with no statistically significant differences between outcomes across the 8 study centres and 17 operators. Furthermore, after a one-year follow-up, patients demonstrated a high freedom from AF and from any atrial arrhythmia, 89% and 73% after an average of 1.3 procedures per patient, respectively. Also, notably there was acute AF termination of 88% for subjects in all centres.3
Additional patterns of behaviour that impact the use of AI in improving health outcomes
While AI has tremendous power to detect patterns of data that correlate with health outcomes, full realisation of that power requires changes in the patterns of human behaviour.
A key challenge to effectively deploying AI-based clinical solutions is the lack of a robust and expansive regulatory framework that can provide regulators, users, and patients with confidence that these solutions have been effectively validated. Overcoming this challenge requires that innovators provide detailed information on how these AI models have been developed and robust clinical evidence that the models work as predicted and are accurate across multiple patient populations. This last point is of particular concern, given the potential for AI-enabled solutions to reinforce existing health biases and inequities if they are trained on data sets that do not reflect the diversity of the patient population in which the solution will be used.
Innovators and regulators must also take a more collaborative approach to ensuring the safe, accurate, and effective use of AI-enabled clinical solutions, especially when it comes to standardising terminology, data guidelines, and metrics. In the AF space alone, there are more than 200 companies working on AI-enabled solutions. Yet, there is little communication among these groups and no specific standards that the community can work towards. Standardisation will be important for enabling head-to-head comparison of a growing number of AF treatment options - which is essential for enabling electrophysiologists and patients to make informed clinical decisions.
Improving health outcomes at the level of the individual requires global cooperation and data sharing among the expanding community of innovators working at the nexus of data science and human health.
References
- Global epidemiology of atrial fibrillation: An increasing epidemic and public health challenge” Giuseppe Lippi, Fabian Sanchis-Gomar, Gianfranco Cervellino
- Epidemiology of Atrial Fibrillation in the 21st Century” Jelena Kornej, Christin S. Börschel, Emelia J. Benjamin and Renate B. Schnabel
- Seitz J, Mohr-Durdez T, Albenque JP et al. Artificial intelligence software standardizes electrogram-based ablation outcome for persistent atrial fibrillation. J Cardiovas Electrophysiol. 2022;33(11):2250-2260.